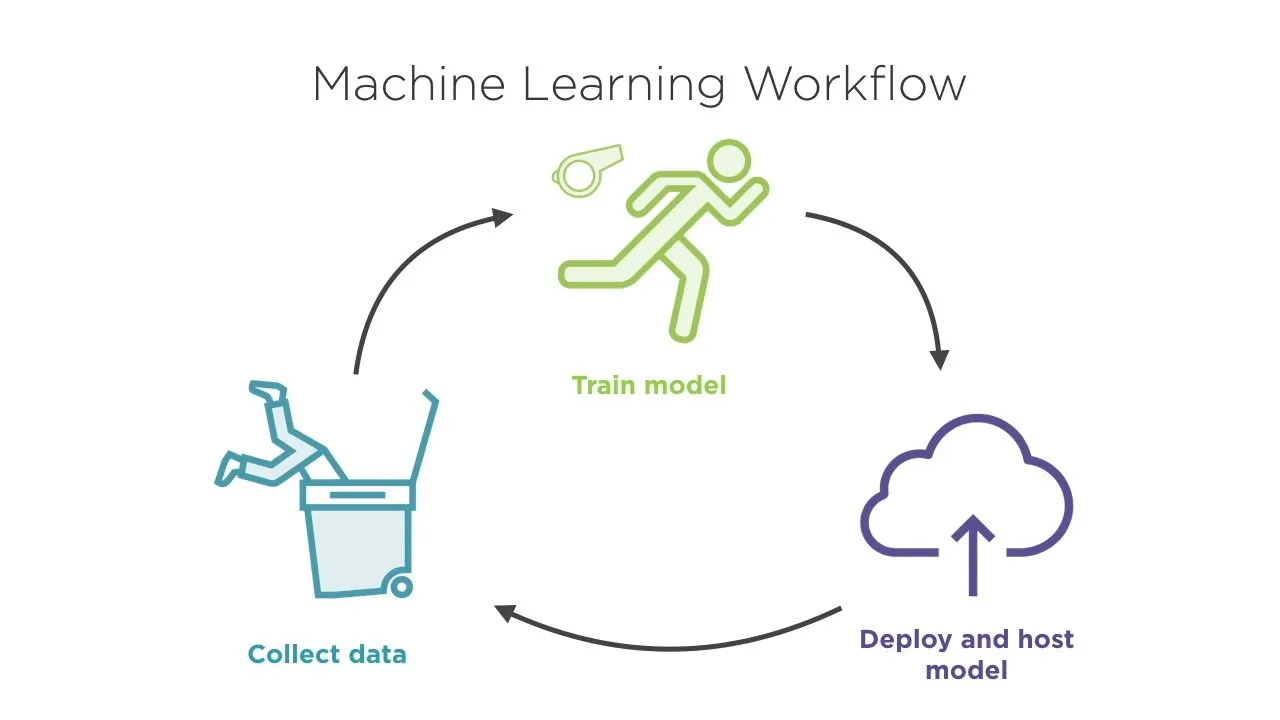
Deploying TensorFlow Models to AWS Azure and the GCP




This course provides an overview of how to deploy TensorFlow models to the cloud platforms of Azure, AWS, and GCP. Learn how to take your TensorFlow model and deploy it locally or to the cloud of your choice. ▼
ADVERTISEMENT
Course Feature
Cost:
Free Trial
Provider:
Pluralsight
Certificate:
Paid Certification
Language:
English
Start Date:
On-Demand
Course Overview
❗The content presented here is sourced directly from Pluralsight platform. For comprehensive course details, including enrollment information, simply click on the 'Go to class' link on our website.
Updated in [March 06th, 2023]
This course, Deploying TensorFlow Models to AWS, Azure, and the GCP, provides an overview of how to take a trained TensorFlow model and deploy it locally or to the cloud platform of your choice - Azure, AWS, or the GCP. It covers topics such as using TensorFlow Serving, containerizing TensorFlow models using Docker on Microsoft Azure, deploying TensorFlow models on Amazon AWS, and deploying TensorFlow models on the Google Cloud Platform. Participants will learn how to save the model parameters of a trained model using the Saved Model interface, a universal interface for TensorFlow models, and how to scale the locally hosted model by packaging all dependencies in a Docker container. They will also get introduced to the AWS SageMaker service, the fully managed ML service offered by Amazon, and learn how to deploy their model on the Google Cloud Platform using the Cloud ML Engine. By the end of the course, participants will be familiar with how a production-ready TensorFlow model is set up as well as how to build and train their models end to end on their local machine and on the three major cloud platforms. Software required: TensorFlow, Python.
[Applications]
After completing this course, learners should be able to apply their knowledge to deploy TensorFlow models to AWS, Azure, and the GCP. Learners should be able to save model parameters using the Saved Model interface, package all dependencies in a Docker container, and deploy models on the Google Cloud Platform using the Cloud ML Engine. Additionally, learners should be able to build and train models end to end on their local machine and on the three major cloud platforms.
[Career Paths]
1. Machine Learning Engineer: Machine learning engineers are responsible for developing and deploying machine learning models. They use a variety of tools and techniques to build, train, and deploy models. They must have a strong understanding of the underlying algorithms and be able to apply them to real-world problems. The demand for machine learning engineers is growing rapidly as more companies are looking to leverage the power of machine learning to improve their products and services.
2. Data Scientist: Data scientists are responsible for analyzing large datasets and extracting insights from them. They use a variety of tools and techniques to analyze data and develop predictive models. They must have a strong understanding of statistics and be able to interpret and communicate the results of their analysis. Data scientists are in high demand as companies are looking to leverage the power of data to make better decisions.
3. Cloud Engineer: Cloud engineers are responsible for managing and deploying cloud-based applications and services. They must have a strong understanding of cloud computing technologies and be able to design and implement solutions that are secure, reliable, and cost-effective. Cloud engineers are in high demand as more companies are looking to move their applications and services to the cloud.
4. AI Developer: AI developers are responsible for developing AI-based applications and services. They must have a strong understanding of AI algorithms and be able to apply them to real-world problems. AI developers are in high demand as more companies are looking to leverage the power of AI to improve their products and services.
[Education Paths]
1. Bachelor of Science in Computer Science: This degree program focuses on the fundamentals of computer science, such as programming, software engineering, and computer architecture. It also covers topics such as artificial intelligence, machine learning, and data science. With the increasing demand for data-driven solutions, this degree path is becoming increasingly popular.
2. Master of Science in Artificial Intelligence: This degree program focuses on the development of intelligent systems and their applications. It covers topics such as natural language processing, computer vision, robotics, and machine learning. This degree path is becoming increasingly popular as AI is being used in more and more industries.
3. Master of Science in Data Science: This degree program focuses on the analysis and interpretation of large datasets. It covers topics such as data mining, machine learning, and predictive analytics. With the increasing demand for data-driven solutions, this degree path is becoming increasingly popular.
4. Master of Science in Machine Learning: This degree program focuses on the development of algorithms and models for machine learning. It covers topics such as supervised and unsupervised learning, deep learning, and reinforcement learning. With the increasing demand for AI-driven solutions, this degree path is becoming increasingly popular.
Course Provider
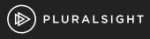
Provider Pluralsight's Stats at AZClass
Pluralsight ranked 16th on the Best Medium Workplaces List.
Pluralsight ranked 20th on the Forbes Cloud 100 list of the top 100 private cloud companies in the world.
Pluralsight Ranked on the Best Workplaces for Women List for the second consecutive year.
AZ Class hope that this free trial Pluralsight course can help your Tensorflow skills no matter in career or in further education. Even if you are only slightly interested, you can take Deploying TensorFlow Models to AWS Azure and the GCP course with confidence!
Discussion and Reviews
0.0 (Based on 0 reviews)
Explore Similar Online Courses
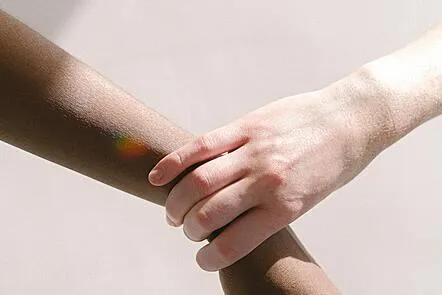
Personal Trainers Toolkit: Mental Health First Aid for Fitness Trainers
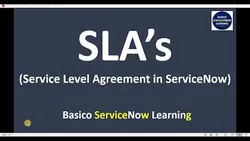
ServiceNow SLA Configuration SLA Definition
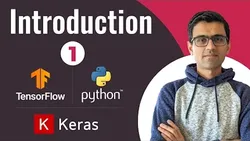
Deep Learning With Tensorflow 20 Keras and Python
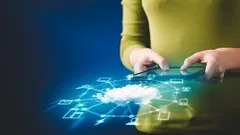
Tensorflow on Googles Cloud Platform for Data Engineers
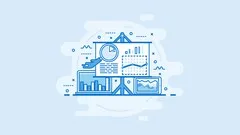
Deep Learning and Reinforcement Learning with Tensorflow
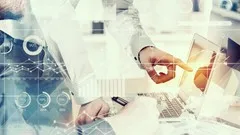
Tensorflow Deep Learning - Data Science in Python
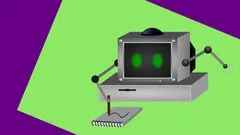
Neural Networks with Tensorflow
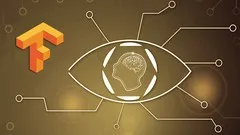
TensorFlow Hub: Deep Learning Computer Vision and NLP
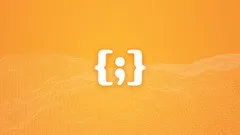
LEARNING PATH: TensorFlow: Computer Vision with TensorFlow
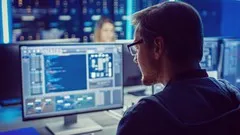
Hands-On Transfer Learning with TensorFlow 20
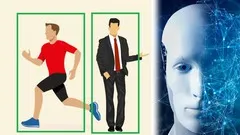
Human Tracking & Counting using TensorFlow & Deep Learning
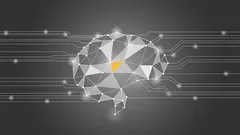
Start your review of Deploying TensorFlow Models to AWS Azure and the GCP