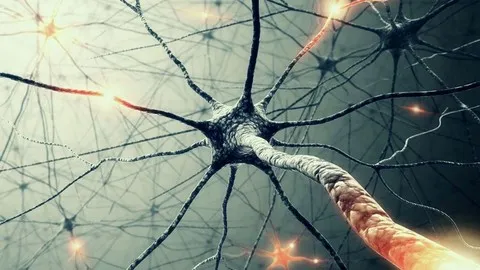
Unsupervised Deep Learning in Python





This course provides an in-depth exploration of unsupervised deep learning techniques. It covers the theory behind principal components analysis (PCA), t-SNE, autoencoders, restricted Boltzmann machines (RBMs) and deep belief networks (DBNs). Through hands-on coding exercises, you will learn how to write the code for PCA, t-SNE, autoencoders, and RBMs in Theano and Tensorflow. You will also gain an understanding of the limitations of PCA and t-SNE, and how stacked autoencoders are used in deep learning. ▼
ADVERTISEMENT
Course Feature
Cost:
Paid
Provider:
Udemy
Certificate:
No Information
Language:
English
Start Date:
Self Paced
Course Overview
❗The content presented here is sourced directly from Udemy platform. For comprehensive course details, including enrollment information, simply click on the 'Go to class' link on our website.
Updated in [August 31st, 2023]
Skills and Knowledge:
By the end of this course, you will have acquired the following skills and knowledge:
- Understanding of the theory behind principal components analysis (PCA)
- Knowledge of why PCA is useful for dimensionality reduction, visualization, de-correlation, and denoising
- Ability to derive the PCA algorithm by hand
- Ability to write the code for PCA
- Understanding of the theory behind t-SNE
- Ability to use t-SNE in code
- Understanding of the limitations of PCA and t-SNE
- Understanding of the theory behind autoencoders
- Ability to write an autoencoder in Theano and Tensorflow
- Understanding of how stacked autoencoders are used in deep learning
- Ability to write a stacked denoising autoencoder in Theano and Tensorflow
- Understanding of the theory behind restricted Boltzmann machines (RBMs)
- Understanding of why RBMs are hard to train
- Understanding of the contrastive divergence algorithm to train RBMs
- Ability to write your own RBM and deep belief network (DBN) in Theano and Tensorflow
- Ability to visualize and interpret the features learned by autoencoders and RBMs
Professional Growth:
This course contributes to professional growth by providing a comprehensive understanding of unsupervised deep learning in Python. Participants will gain a deep understanding of the theory behind principal components analysis (PCA), t-SNE, autoencoders, and restricted Boltzmann machines (RBMs). They will also learn how to write code for PCA, t-SNE, autoencoders, and RBMs in Theano and Tensorflow. Additionally, they will learn how to use stacked autoencoders and deep belief networks (DBNs) in deep learning. By the end of the course, participants will have a comprehensive understanding of unsupervised deep learning and be able to apply it to their own projects.
Further Education:
This course titled "Unsupervised Deep Learning in Python" seems suitable for preparing for further education. It covers various topics related to unsupervised deep learning, such as principal components analysis (PCA), t-SNE, autoencoders, and restricted Boltzmann machines (RBMs). These concepts are fundamental in the field of machine learning and can be applied in various domains. Additionally, the course includes hands-on coding exercises using popular deep learning frameworks like Theano and TensorFlow, which can enhance practical skills. Overall, this course can provide a solid foundation for further education in the field of deep learning and related areas.
Course Syllabus
Principal Components Analysis
t-SNE (t-distributed Stochastic Neighbor Embedding)
Autoencoders
Restricted Boltzmann Machines
The Vanishing Gradient Problem
Extras + Visualizing what features a neural network has learned
Applications to NLP (Natural Language Processing)
Applications to Recommender Systems
Theano and Tensorflow Basics Review
Setting Up Your Environment (FAQ by Student Request)
Extra Help With Python Coding for Beginners (FAQ by Student Request)
Effective Learning Strategies for Machine Learning (FAQ by Student Request)
Course Provider
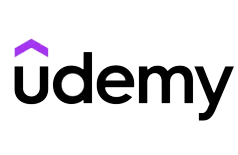
Provider Udemy's Stats at AZClass
Discussion and Reviews
0.0 (Based on 0 reviews)
Explore Similar Online Courses

Sell Your Expert Opinion Online: Be The Guru You Already Are
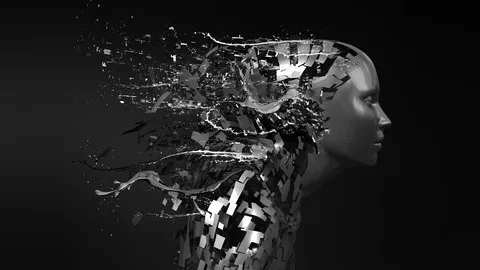
Deep Learning A-Z: Hands-On Artificial Neural Networks
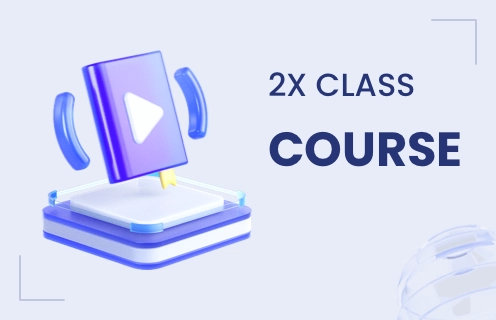
Python for Informatics: Exploring Information
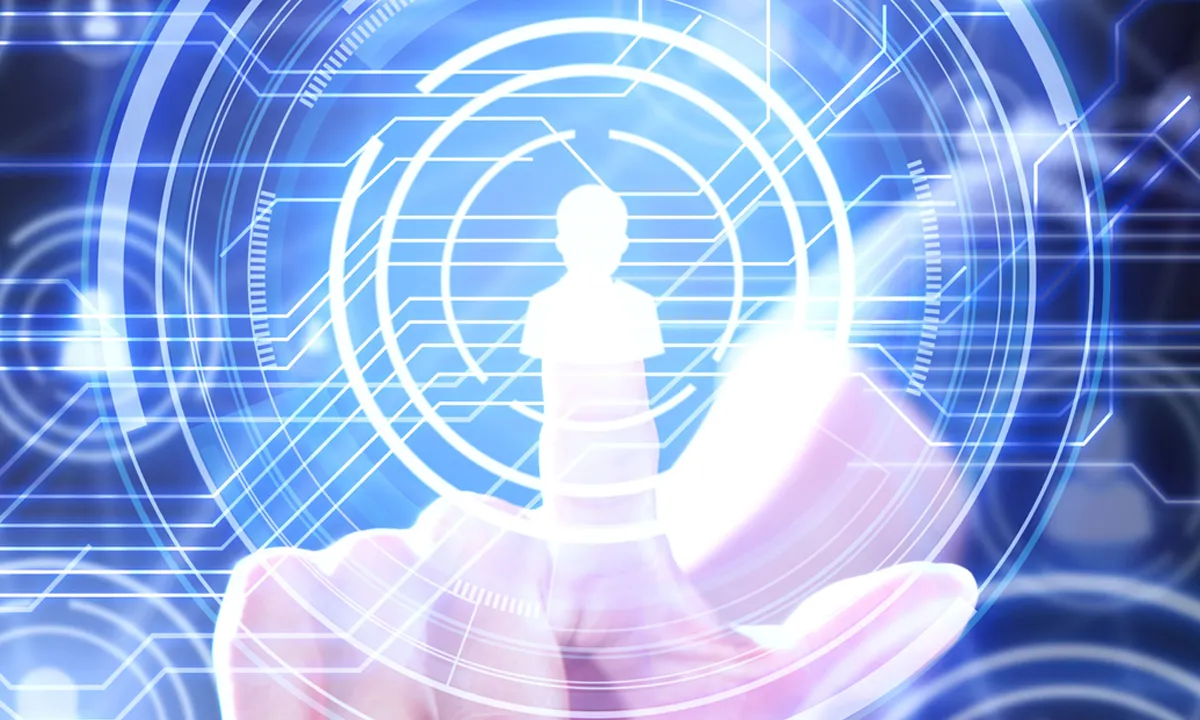
Social Network Analysis

Introduction to Systematic Review and Meta-Analysis
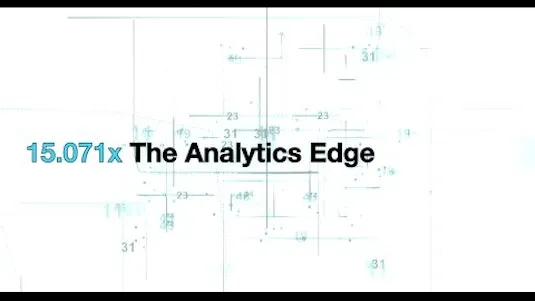
The Analytics Edge

DCO042 - Python For Informatics

Causal Diagrams: Draw Your Assumptions Before Your Conclusions
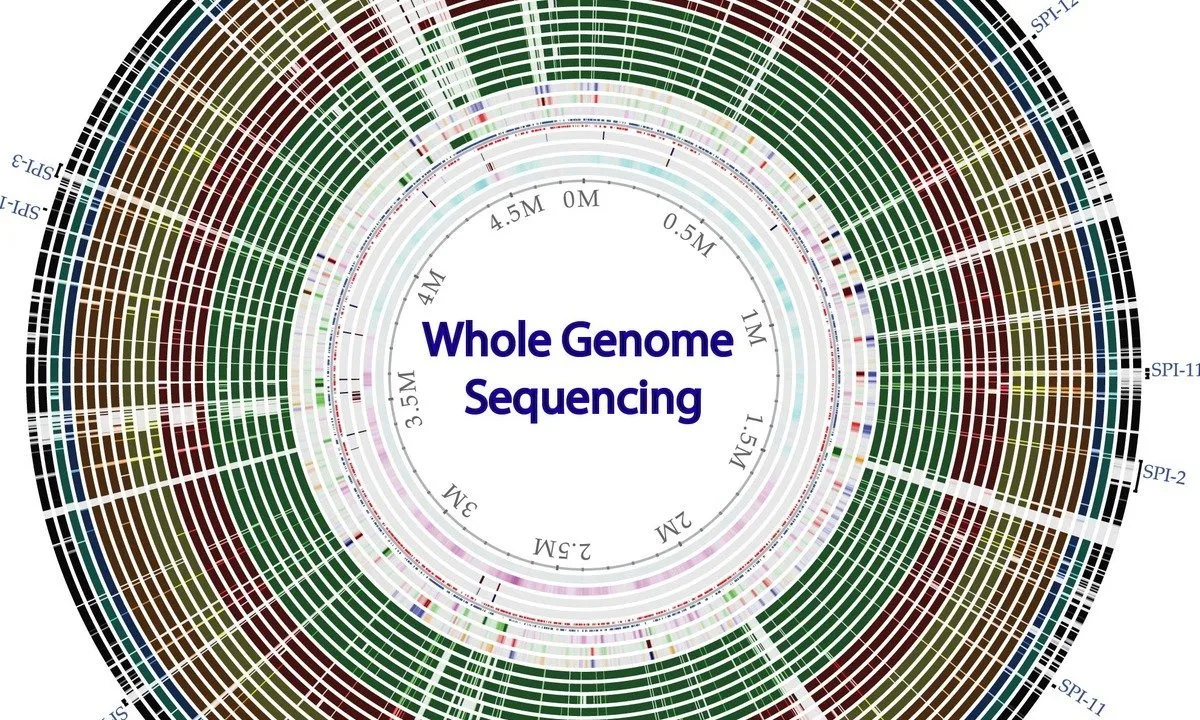
Whole genome sequencing of bacterial genomes - tools and applications

Deep Learning & Neural Networks Python Keras For Dummies

Deep Learning

Start your review of Unsupervised Deep Learning in Python