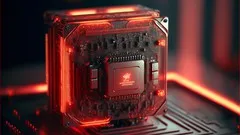
Advanced Reinforcement Learning in Python: from DQN to SAC





This Advanced Reinforcement Learning course on Udemy is the most comprehensive of its kind. It will teach you to implement powerful Deep Reinforcement Learning algorithms in Python using PyTorch and PyTorch lightning. You will learn to create adaptive Artificial Intelligence agents that can solve decision-making tasks, combining Reinforcement Learning techniques with Neural Networks and Deep Learning methods. This course will also prepare you for the next courses in the series, where you will explore other advanced methods. With practical modules and jupyter notebooks, you will be able to develop your skills from scratch. Don't miss this opportunity to become an expert in Reinforcement Learning! ▼
ADVERTISEMENT
Course Feature
Cost:
Paid
Provider:
Udemy
Certificate:
Paid Certification
Language:
English
Start Date:
2023-03-15
Course Overview
❗The content presented here is sourced directly from Udemy platform. For comprehensive course details, including enrollment information, simply click on the 'Go to class' link on our website.
Updated in [August 18th, 2023]
Skills and Knowledge:
This course will provide students with the skills and knowledge to develop practical Reinforcement Learning algorithms in Python using PyTorch and PyTorch Lightning. Students will learn to implement adaptive algorithms that solve control tasks based on experience, as well as combine these techniques with Neural Networks and Deep Learning methods to create adaptive Artificial Intelligence agents. Additionally, students will gain an understanding of the Markov decision process (MDP), Q-Learning, Neural Networks, Deep Q-Learning, Policy Gradient methods, Hyperparameter tuning with Optuna, Deep Q-Learning for continuous action spaces (Normalized advantage function - NAF), Deep Deterministic Policy Gradient (DDPG), Twin Delayed DDPG (TD3), Soft Actor-Critic (SAC), and Hindsight Experience Replay (HER).
Professional Growth:
This course provides a comprehensive introduction to the field of Advanced Reinforcement Learning. It covers the fundamentals of the Markov decision process (MDP), Q-Learning, Neural Networks, Deep Q-Learning, Policy Gradient methods, PyTorch Lightning, Hyperparameter tuning with Optuna, Deep Q-Learning for continuous action spaces, Deep Deterministic Policy Gradient (DDPG), Twin Delayed DDPG (TD3), Soft Actor-Critic (SAC), and Hindsight Experience Replay (HER). By learning these techniques, professionals can gain the skills necessary to develop adaptive Artificial Intelligence agents capable of solving decision-making tasks. This course will also prepare professionals for the next courses in this series, where they will explore other advanced methods that excel in other types of task.
Further Education:
Yes, this course is suitable for preparing further education. It covers a wide range of topics related to advanced reinforcement learning, from the Markov decision process (MDP) to Soft Actor-Critic (SAC). It also provides refresher modules on Q-Learning, Neural Networks, and Deep Q-Learning, as well as introducing students to PyTorch Lightning and Optuna for hyperparameter tuning. This course will provide students with the necessary knowledge and skills to pursue further education in the field of reinforcement learning.
Course Syllabus
Introduction
Refresher: The Markov Decision Process (MDP)
Refresher: Q-Learning
Refresher: Brief introduction to Neural Networks
Refresher: Deep Q-Learning
PyTorch Lightning
Hyperparameter tuning with Optuna
Deep Q-Learning for continuous action spaces (Normalized Advantage Function)
Refresher: Policy gradient methods
Deep Deterministic Policy Gradient (DDPG)
Twin Delayed DDPG (TD3)
Soft Actor-Critic (SAC)
Hindsight Experience Replay
Final steps
Course Provider
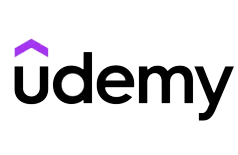
Provider Udemy's Stats at AZClass
Discussion and Reviews
0.0 (Based on 0 reviews)
Explore Similar Online Courses
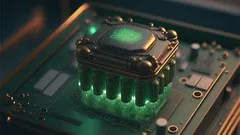
Advanced Reinforcement Learning in Python: cutting-edge DQNs
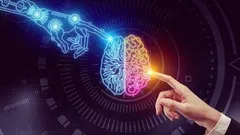
Machine Learning: Beginner Reinforcement Learning in Python
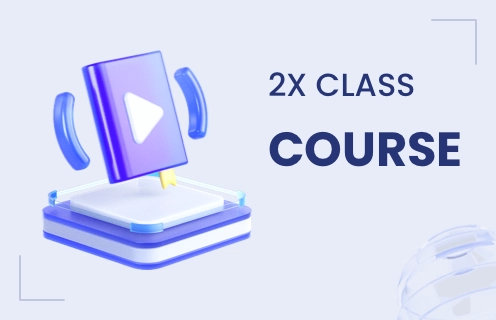
Python for Informatics: Exploring Information
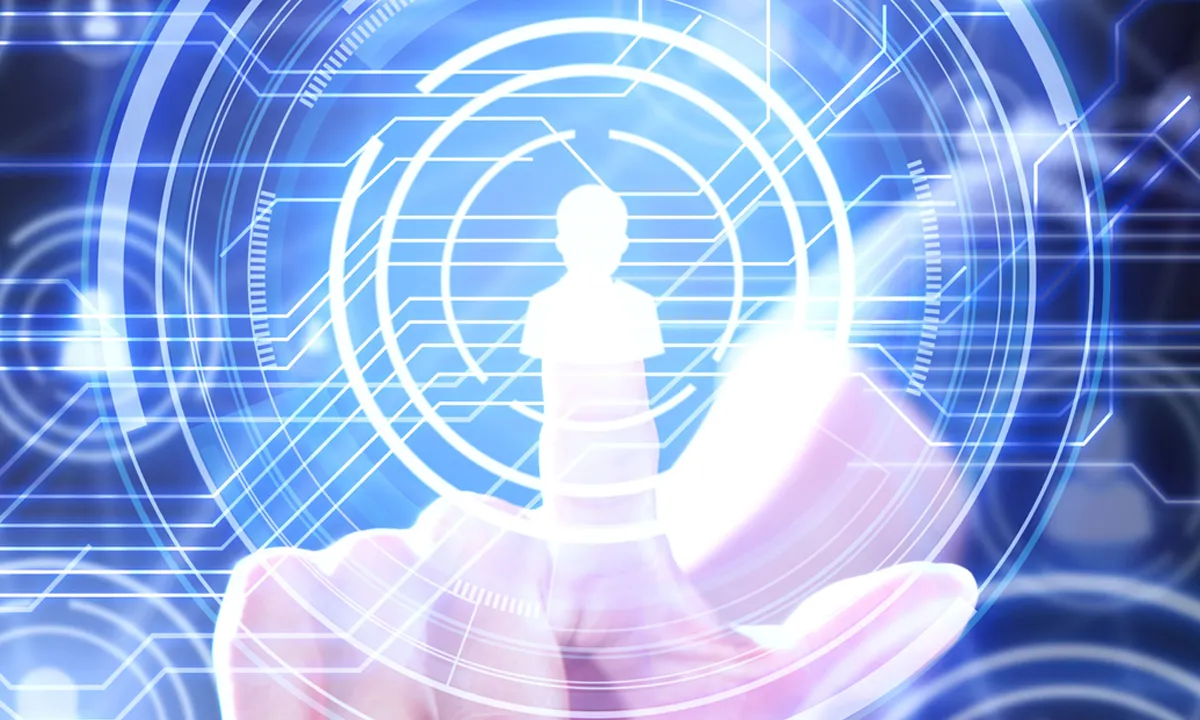
Social Network Analysis

Introduction to Systematic Review and Meta-Analysis
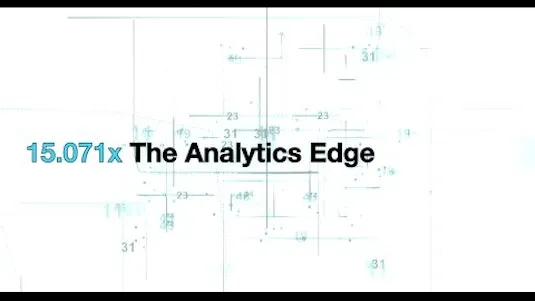
The Analytics Edge

DCO042 - Python For Informatics

Causal Diagrams: Draw Your Assumptions Before Your Conclusions
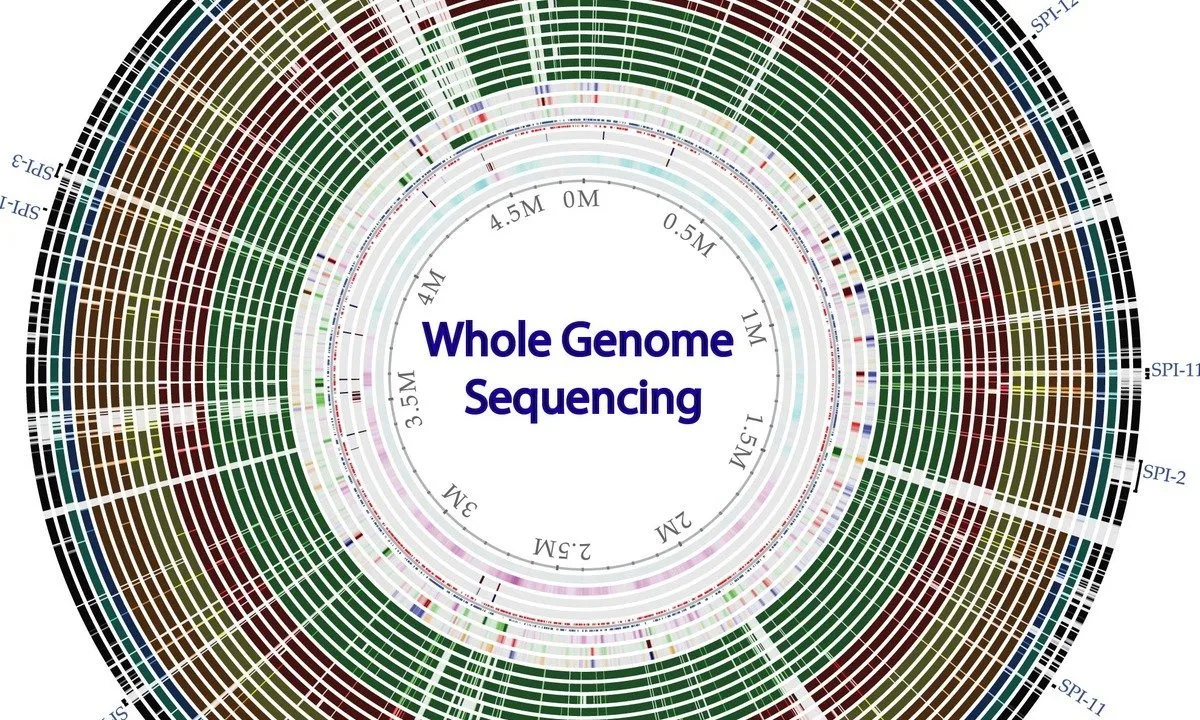
Whole genome sequencing of bacterial genomes - tools and applications
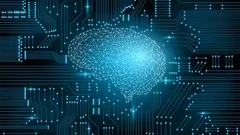
State-of-the-art Research of Deep Reinforcement-learning
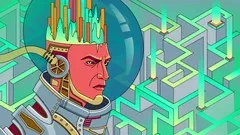
Deep Reinforcement Learning 20
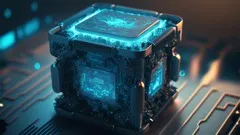
Start your review of Advanced Reinforcement Learning in Python: from DQN to SAC