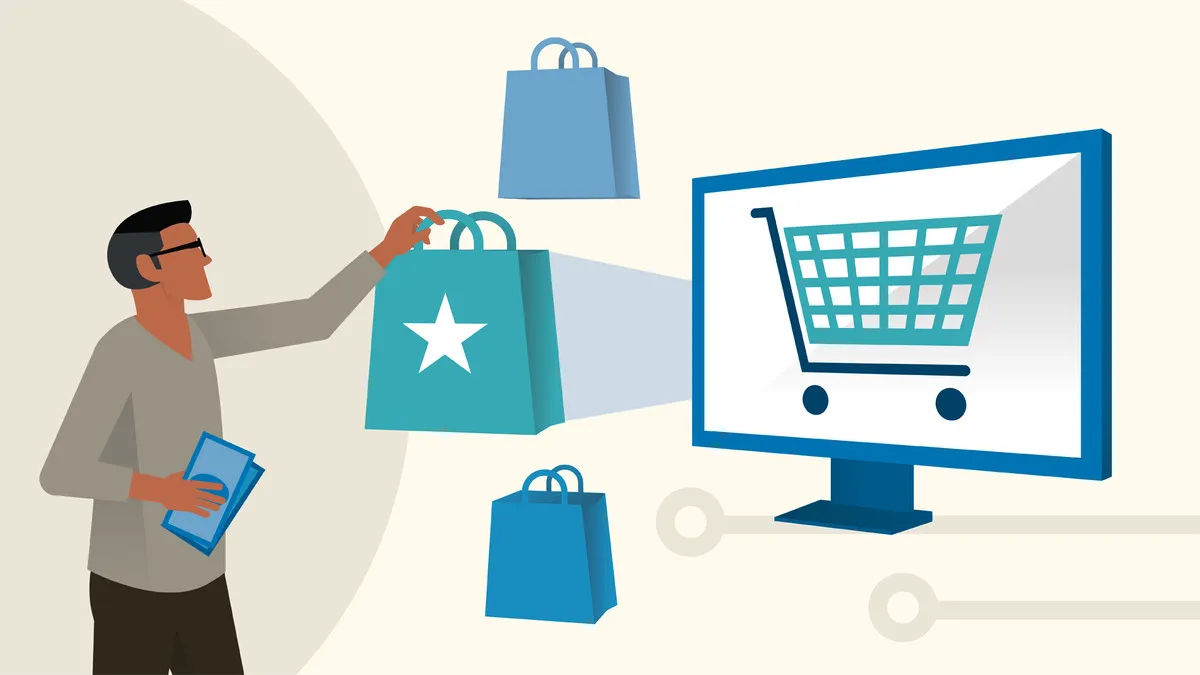
Building Recommender Systems with Machine Learning and AI





This course provides an introduction to building recommender systems using machine learning and AI. Students will gain an understanding of how to use deep learning, neural networks, and machine learning to create personalized recommendations for users. ▼
ADVERTISEMENT
Course Feature
Cost:
Free Trial
Provider:
LinkedIn Learning
Certificate:
No Information
Language:
English
Start Date:
Self Paced
Course Overview
❗The content presented here is sourced directly from LinkedIn Learning platform. For comprehensive course details, including enrollment information, simply click on the 'Go to class' link on our website.
Updated in [March 06th, 2023]
This course, Building Recommender Systems with Machine Learning and AI, provides an overview of the various recommendation algorithms based on neighborhood-based collaborative filtering and more modern techniques, including matrix factorization and even deep learning with artificial neural networks. Participants will gain an understanding of the real-world challenges of applying these algorithms at a large scale with real-world data. Additionally, participants will learn how to develop their own framework to test algorithms and build their own neural networks using technologies like Amazon DSSTNE, AWS SageMaker, and TensorFlow.
[Applications]
After completing this course, students will be able to apply their knowledge to develop their own recommender systems. They will be able to use neighborhood-based collaborative filtering and more modern techniques, such as matrix factorization and deep learning with artificial neural networks. Additionally, they will be able to develop their own frameworks to test algorithms and build their own neural networks using technologies such as Amazon DSSTNE, AWS SageMaker, and TensorFlow.
[Career Paths]
1. Data Scientist: Data Scientists are responsible for analyzing large datasets to uncover trends and insights. They use a variety of techniques, including machine learning and AI, to develop models and algorithms that can be used to make predictions and recommendations. Data Scientists are in high demand and the field is expected to continue to grow in the coming years.
2. Machine Learning Engineer: Machine Learning Engineers are responsible for developing and deploying machine learning models and algorithms. They use a variety of technologies, such as Amazon DSSTNE, AWS SageMaker, and TensorFlow, to build and deploy models. Machine Learning Engineers are in high demand and the field is expected to continue to grow in the coming years.
3. AI Engineer: AI Engineers are responsible for developing and deploying AI-based solutions. They use a variety of technologies, such as natural language processing, computer vision, and deep learning, to build and deploy AI-based solutions. AI Engineers are in high demand and the field is expected to continue to grow in the coming years.
4. Recommendation System Engineer: Recommendation System Engineers are responsible for developing and deploying recommendation systems. They use a variety of techniques, such as neighborhood-based collaborative filtering and matrix factorization, to build and deploy recommendation systems. Recommendation System Engineers are in high demand and the field is expected to continue to grow in the coming years.
[Education Paths]
1. Bachelor of Science in Computer Science: This degree path focuses on the fundamentals of computer science, including programming, algorithms, data structures, and software engineering. It also covers topics such as artificial intelligence, machine learning, and natural language processing. With the increasing demand for AI and machine learning professionals, this degree path is becoming increasingly popular.
2. Master of Science in Artificial Intelligence: This degree path focuses on the development of AI systems and their applications. It covers topics such as machine learning, natural language processing, computer vision, robotics, and more. It also provides students with the opportunity to develop their own AI projects and gain hands-on experience.
3. Master of Science in Data Science: This degree path focuses on the analysis and interpretation of large datasets. It covers topics such as data mining, machine learning, and predictive analytics. It also provides students with the opportunity to develop their own data science projects and gain hands-on experience.
4. Doctor of Philosophy in Machine Learning: This degree path focuses on the development of advanced machine learning algorithms and their applications. It covers topics such as deep learning, reinforcement learning, and natural language processing. It also provides students with the opportunity to develop their own machine learning projects and gain hands-on experience.
Course Syllabus
Install Anaconda, review course materials, and create movie recommendations
Course roadmap
Understanding you through implicit and explicit ratings
Top-N recommender architecture
Review the basics of recommender systems
Course Provider
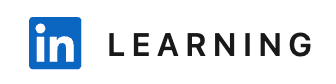
Provider LinkedIn Learning's Stats at AZClass
Building Recommender Systems with Machine Learning and AI describes how to build recommender systems using machine learning and artificial intelligence. Learners will understand the real-world challenges of applying these algorithms at scale using real-world data. They will also learn how to develop their own frameworks to test algorithms and build their own neural networks using technologies such as Amazon DSSTNE, AWS SageMaker, and TensorFlow. This course is ideal for those interested in data science, machine learning, and artificial intelligence, as it provides a comprehensive overview of the fundamentals of building recommender systems.
Discussion and Reviews
0.0 (Based on 0 reviews)
Explore Similar Online Courses
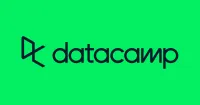
Bayesian Data Analysis in Python

Payroll Processing in India - An Introduction
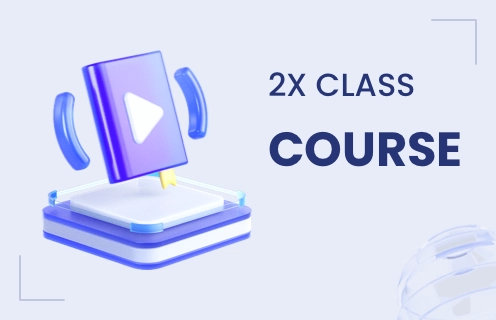
Python for Informatics: Exploring Information
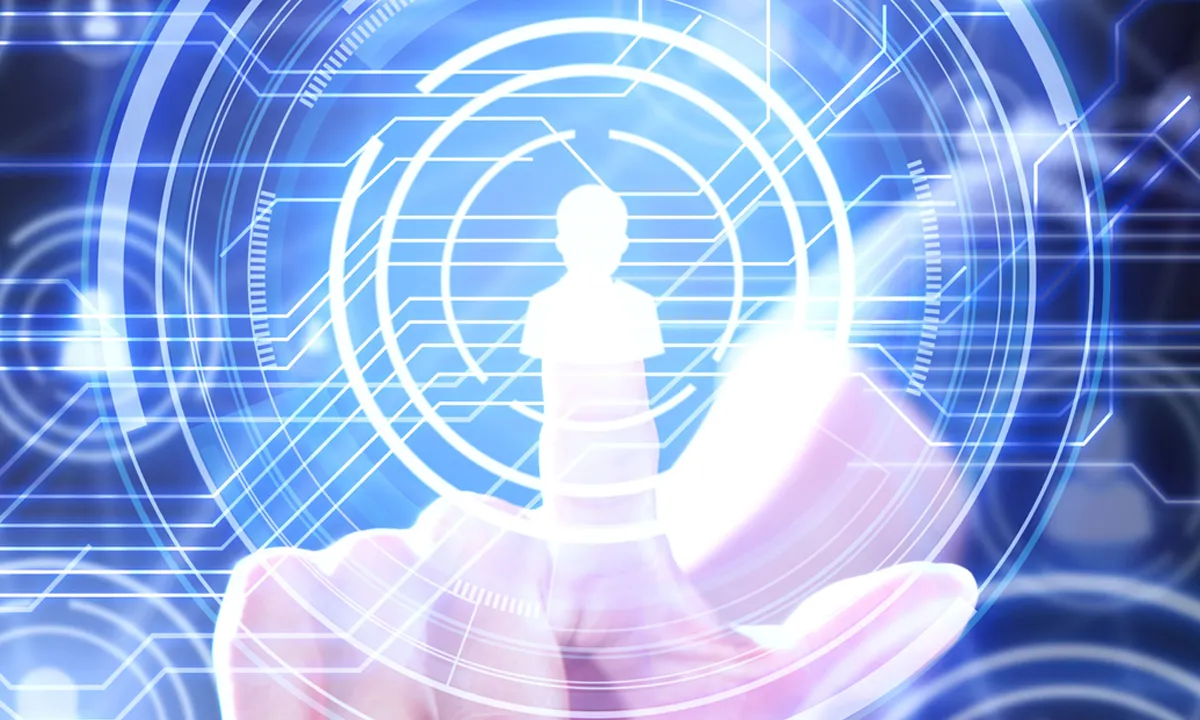
Social Network Analysis

Introduction to Systematic Review and Meta-Analysis
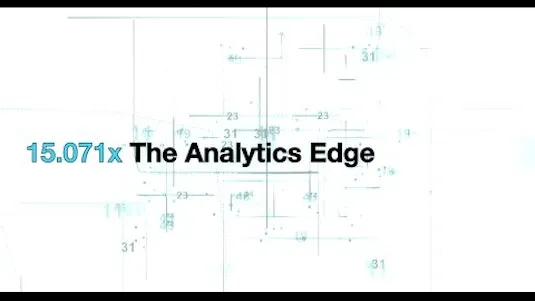
The Analytics Edge

DCO042 - Python For Informatics

Causal Diagrams: Draw Your Assumptions Before Your Conclusions
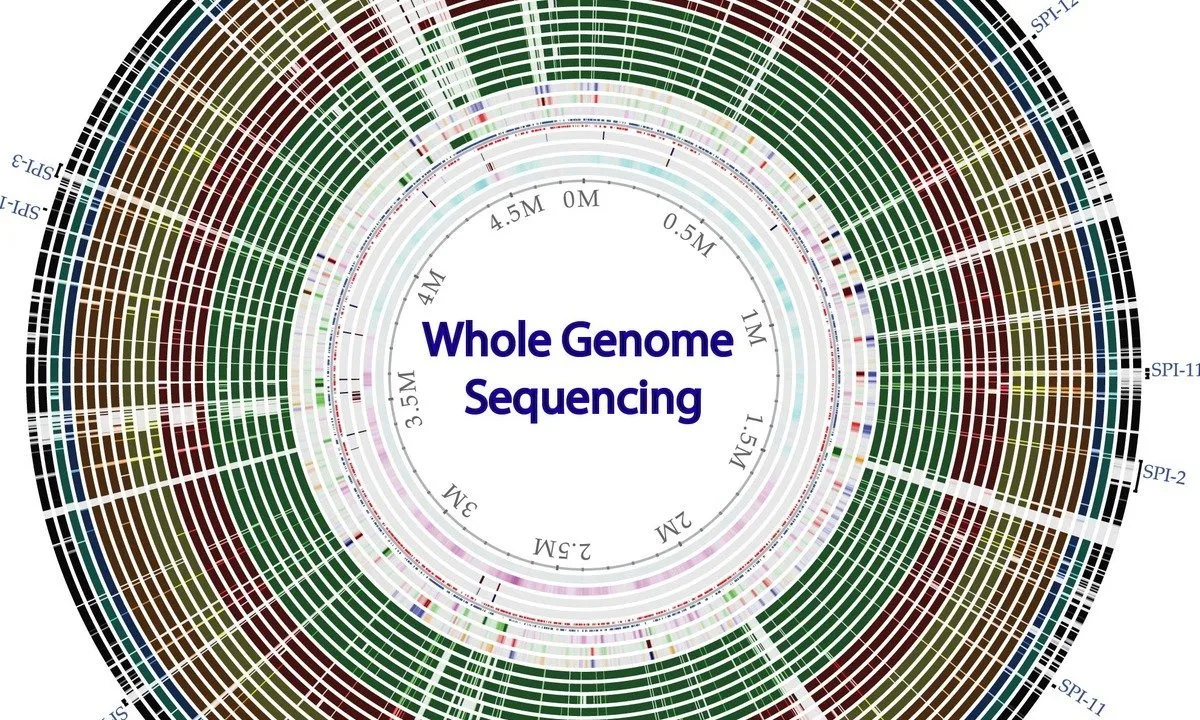
Whole genome sequencing of bacterial genomes - tools and applications
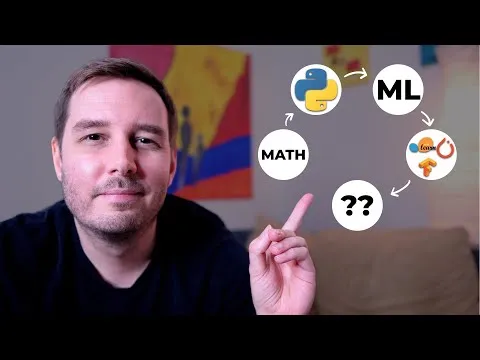
How I would learn Machine Learning (if I could start over)
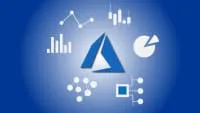
Learn Data Science and Machine Learning on Microsoft Azure
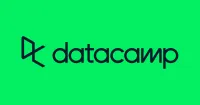
Start your review of Building Recommender Systems with Machine Learning and AI