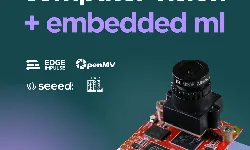
Computer Vision with Embedded Machine Learning




This course explores the field of Computer Vision (CV) and how it can be used to automate the process of assigning meaning to digital images or videos. It covers the use of Machine Learning (ML) algorithms and techniques to accomplish CV tasks, and how these techniques can be deployed to embedded systems. ▼
ADVERTISEMENT
Course Feature
Cost:
Free
Provider:
Coursera
Certificate:
Paid Certification
Language:
English
Start Date:
12th Jun, 2023
Course Overview
❗The content presented here is sourced directly from Coursera platform. For comprehensive course details, including enrollment information, simply click on the 'Go to class' link on our website.
Updated in [March 20th, 2023]
Computer vision (CV) is a fascinating field of study that attempts to automate the process of assigning meaning to digital images or videos. In other words, we are helping computers see and understand the world around us! A number of machine learning (ML) algorithms and techniques can be used to accomplish CV tasks, and as ML becomes faster and more efficient, we can deploy these techniques to embedded systems.
This course, offered by a partnership among Edge Impulse, OpenMV, Seeed Studio, and the TinyML Foundation, will give you an understanding of how deep learning with neural networks can be used to classify images and detect objects in images and videos. You will have the opportunity to deploy these machine learning models to embedded systems, which is known as embedded machine learning or TinyML.
Familiarity with the Python programming language and basic ML concepts (such as neural networks, training, inference, and evaluation) is advised to understand some topics as well as complete the projects. Some math (reading plots, arithmetic, algebra) is also required for quizzes and projects. If you have not done so already, taking the "Introduction to Embedded Machine Learning" course is recommended.
This course covers the concepts and vocabulary necessary to understand how convolutional neural networks (CNNs) operate, and it covers how to use them to classify images and detect objects. The hands-on projects will give you the opportunity to train your own CNNs and deploy them to a microcontroller and/or single board computer.
(Please note that we obtained the following content based on information that users may want to know, such as skills, applicable scenarios, future development, etc., combined with AI tools, and have been manually reviewed)
Learners can learn the following from this course:
1. Computer Vision (CV): Understand the fundamentals of CV and how to use ML algorithms and techniques to automate the process of assigning meaning to digital images or videos.
2. Embedded Machine Learning (TinyML): Learn how to deploy ML models to embedded systems and gain an understanding of deep learning with neural networks.
3. Python Programming: Become familiar with the Python programming language and basic ML concepts such as neural networks, training, inference, and evaluation.
4. Convolutional Neural Networks (CNNs): Gain an understanding of the concepts and vocabulary necessary to understand how CNNs operate, and learn how to use them to classify images and detect objects.
[Applications]
Upon completion of this course, participants should have a better understanding of how to use deep learning with neural networks to classify images and detect objects in images and videos. They should also have the skills to deploy these machine learning models to embedded systems. Participants should be able to apply these skills to their own projects and use them to create their own computer vision applications.
[Career Paths]
1. Computer Vision Engineer: Computer vision engineers are responsible for developing and deploying computer vision algorithms and systems. They must have a strong understanding of computer vision, machine learning, and embedded systems. They must also be able to design and implement efficient algorithms and systems that can be deployed to embedded systems. As the demand for computer vision applications increases, the need for computer vision engineers is expected to grow.
2. Machine Learning Engineer: Machine learning engineers are responsible for developing and deploying machine learning algorithms and systems. They must have a strong understanding of machine learning, computer vision, and embedded systems. They must also be able to design and implement efficient algorithms and systems that can be deployed to embedded systems. As the demand for machine learning applications increases, the need for machine learning engineers is expected to grow.
3. Embedded Systems Engineer: Embedded systems engineers are responsible for developing and deploying embedded systems. They must have a strong understanding of embedded systems, computer vision, and machine learning. They must also be able to design and implement efficient systems that can be deployed to embedded systems. As the demand for embedded systems increases, the need for embedded systems engineers is expected to grow.
4. TinyML Engineer: TinyML engineers are responsible for developing and deploying TinyML applications. They must have a strong understanding of TinyML, computer vision, and machine learning. They must also be able to design and implement efficient algorithms and systems that can be deployed to embedded systems. As the demand for TinyML applications increases, the need for TinyML engineers is expected to grow.
[Education Paths]
Recommended Degree Paths:
1. Bachelor of Science in Computer Science: This degree program provides students with a comprehensive understanding of computer science fundamentals, including programming, algorithms, data structures, operating systems, and computer architecture. It also covers topics such as artificial intelligence, machine learning, computer vision, and embedded systems. This degree is ideal for those interested in pursuing a career in computer vision and embedded machine learning.
2. Master of Science in Artificial Intelligence: This degree program focuses on the development of intelligent systems, including machine learning, natural language processing, computer vision, and robotics. It provides students with the skills and knowledge necessary to design, develop, and deploy AI-based systems. This degree is ideal for those interested in pursuing a career in computer vision and embedded machine learning.
3. Doctor of Philosophy in Computer Science: This degree program provides students with an in-depth understanding of computer science fundamentals, including programming, algorithms, data structures, operating systems, and computer architecture. It also covers topics such as artificial intelligence, machine learning, computer vision, and embedded systems. This degree is ideal for those interested in pursuing a career in computer vision and embedded machine learning research.
Developing Trends:
1. Edge Computing: Edge computing is a distributed computing paradigm that brings computation and data storage closer to the edge of the network, where data is generated and consumed. This technology is becoming increasingly important for computer vision and embedded machine learning applications, as it allows for faster and more efficient processing of data.
2. Autonomous Systems: Autonomous systems are systems that are capable of performing tasks without human intervention. This technology is becoming increasingly important for computer vision and embedded machine learning applications, as it allows for more efficient and accurate processing of data.
3. Deep Learning: Deep learning is a subset of machine learning that uses neural networks to learn from large amounts of data. This technology is becoming increasingly important for computer vision and embedded machine learning applications, as it allows for more accurate and efficient processing of data.
Pros & Cons
Clear and engaging explanations
Updated info and correct topics depth
Comprehensive and recommended
Great introduction to CV with EML
Amazing course
Not suitable without Edge Impulse
Open MV
and Raspberry Pi
Module 1 and 2 could be shorter
Object Detection needed more explanations
Videos could be shorter
3rd week was too fast
Course Provider

Provider Coursera's Stats at AZClass
Discussion and Reviews
0.0 (Based on 0 reviews)
Explore Similar Online Courses

Microsoft Excel tricks (MacBook & Windows) beginner-advanced
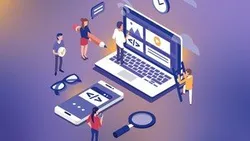
Introduction to TDD in C#
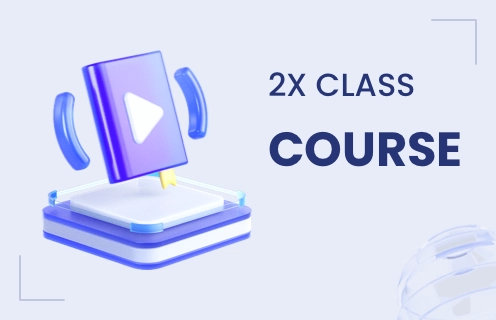
Python for Informatics: Exploring Information
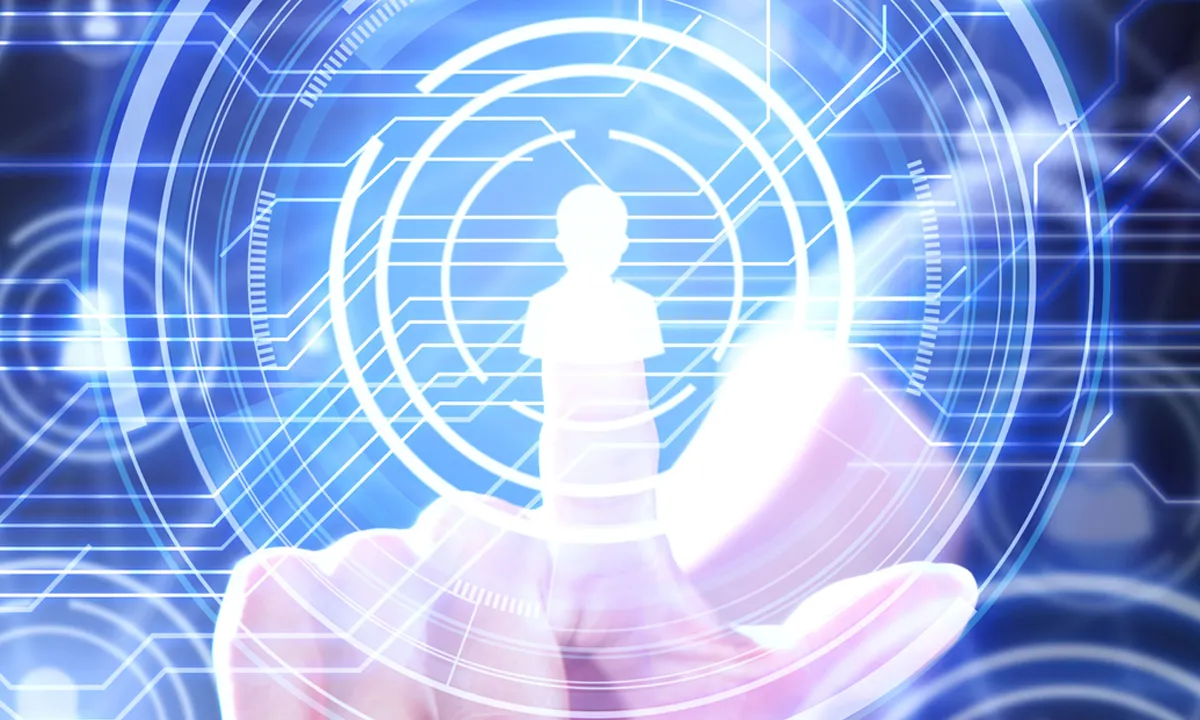
Social Network Analysis

Introduction to Systematic Review and Meta-Analysis
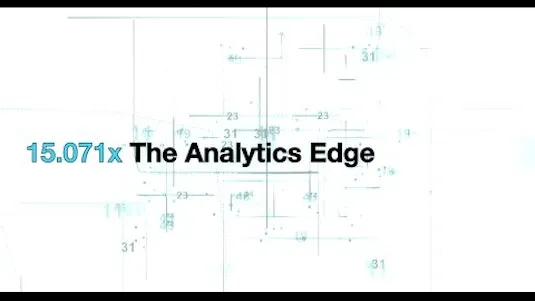
The Analytics Edge

DCO042 - Python For Informatics

Causal Diagrams: Draw Your Assumptions Before Your Conclusions
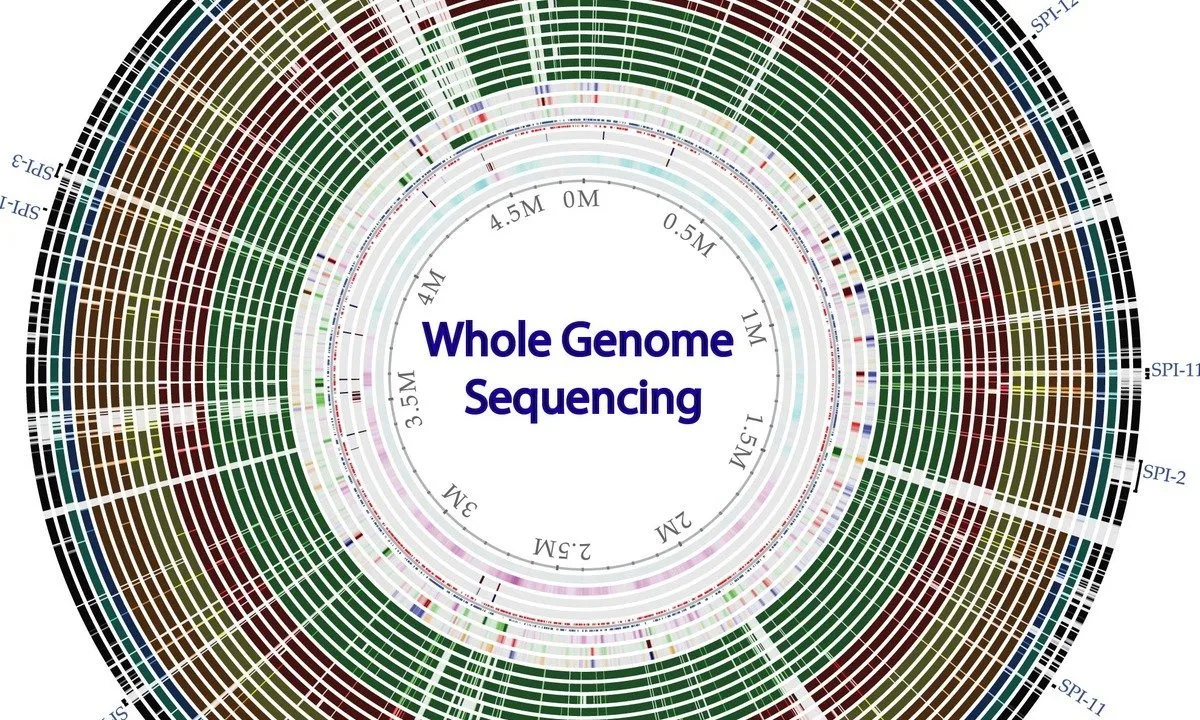
Whole genome sequencing of bacterial genomes - tools and applications
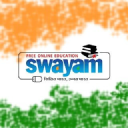
Introduction to Computer Vision
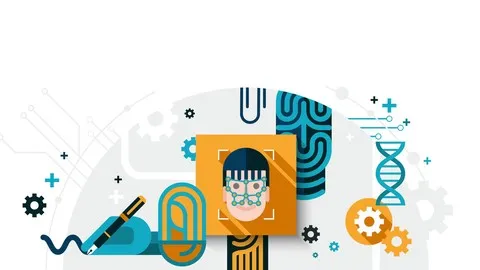
Learn Computer Vision with OpenCV Library using Python
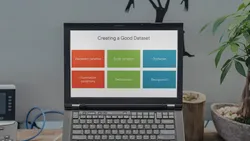
Build an Object Detection Model with Python
Related Categories
Popular Providers
Quiz
Submitted Sucessfully
1. What is the prerequisite for this course?
2. What is the main focus of this course?
3. What is the recommended course to take before this one?
4. What is the partnership between Edge Impulse, OpenMV, Seeed Studio, and the TinyML Foundation?


Start your review of Computer Vision with Embedded Machine Learning