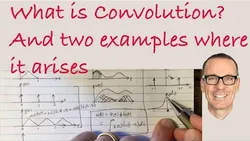
Convolution





Convolution is a mathematical operation which involves two functions, usually in the form of signals or images, to produce a third function. It is commonly used in signal processing, image processing, and other areas of mathematics. Two examples of convolution are the convolution of a square with a rectangle, and the convolution equation which can be explained using YouTube videos. Understanding convolution can be difficult, but there are many resources available to help. ▼
ADVERTISEMENT
Course Feature
Cost:
Free
Provider:
Youtube
Certificate:
Paid Certification
Language:
English
Start Date:
On-Demand
Course Overview
❗The content presented here is sourced directly from Youtube platform. For comprehensive course details, including enrollment information, simply click on the 'Go to class' link on our website.
Updated in [February 21st, 2023]
What is Convolution? And Two Examples where it arises.
Convolution Equation Explained ("Best explanation on YouTube").
How to Understand Convolution ("This is an incredible explanation").
Convolution of Square with Rectangle.
Convolution with Delta Function.
Convolution Square with Exponential.
Convolution Using the Fourier Transform - Example.
Discrete Time System Output Example.
Convolution of two Exponentials.
How are Correlation and Convolution Related in Digital Communications?.
Discrete Time Convolution Example.
(Please note that we obtained the following content based on information that users may want to know, such as skills, applicable scenarios, future development, etc., combined with AI tools, and have been manually reviewed)
1. Understanding the Basics of Convolution: Learners can gain an understanding of the basics of convolution, including what it is and two examples where it arises. They can also learn the convolution equation and how to understand it.
2. Working with Examples: Learners can gain hands-on experience with convolution by working through examples. This includes convolution of a square with a rectangle, convolution with a delta function, convolution of a square with an exponential, convolution using the Fourier transform, discrete time system output example, convolution of two exponentials, and how correlation and convolution are related in digital communications.
3. Visualizing the Process: Learners can gain a better understanding of convolution by visualizing the process. This includes understanding how the convolution equation works and how it can be used to solve problems.
4. Applying the Knowledge: Learners can apply their knowledge of convolution to real-world problems. This includes understanding how convolution can be used in digital communications and how it can be used to solve problems in other areas.
5. Developing Problem-Solving Skills: Learners can develop their problem-solving skills by working through examples and applying their knowledge of convolution to real-world problems. This can help them become better problem solvers and more confident in their ability to solve complex problems.
[Applications]
Students can apply their knowledge of convolution to a variety of fields. For example, they can use convolution to analyze signals in digital communications, or to understand the behavior of discrete time systems. They can also use convolution to analyze the behavior of square and rectangular shapes, or to understand the relationship between correlation and convolution. Additionally, students can use convolution to analyze the behavior of delta functions, exponentials, and Fourier transforms.
[Career Paths]
1. Machine Learning Engineer: Machine learning engineers are responsible for developing and deploying machine learning models. They use convolutional neural networks (CNNs) to create models that can recognize patterns in data and make predictions. They also use convolutional layers to extract features from images and videos. As the demand for machine learning increases, the need for machine learning engineers is expected to grow.
2. Computer Vision Engineer: Computer vision engineers use convolutional neural networks (CNNs) to create models that can recognize objects in images and videos. They also use convolutional layers to extract features from images and videos. As the demand for computer vision applications increases, the need for computer vision engineers is expected to grow.
3. Data Scientist: Data scientists use convolutional neural networks (CNNs) to create models that can recognize patterns in data and make predictions. They also use convolutional layers to extract features from images and videos. As the demand for data science increases, the need for data scientists is expected to grow.
4. Robotics Engineer: Robotics engineers use convolutional neural networks (CNNs) to create models that can recognize objects in images and videos. They also use convolutional layers to extract features from images and videos. As the demand for robotics applications increases, the need for robotics engineers is expected to grow.
Course Provider
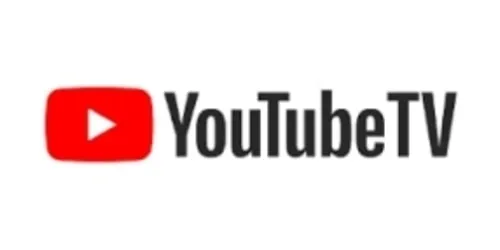
Provider Youtube's Stats at AZClass
Discussion and Reviews
0.0 (Based on 0 reviews)
Explore Similar Online Courses

"Drawing Houses Step by Step" based on a very nice example of a wooden cottage
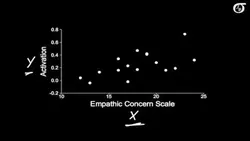
Simple Linear Regression
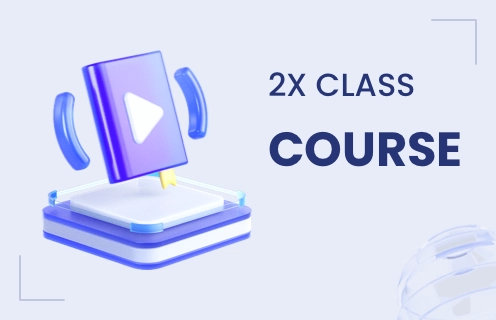
Python for Informatics: Exploring Information
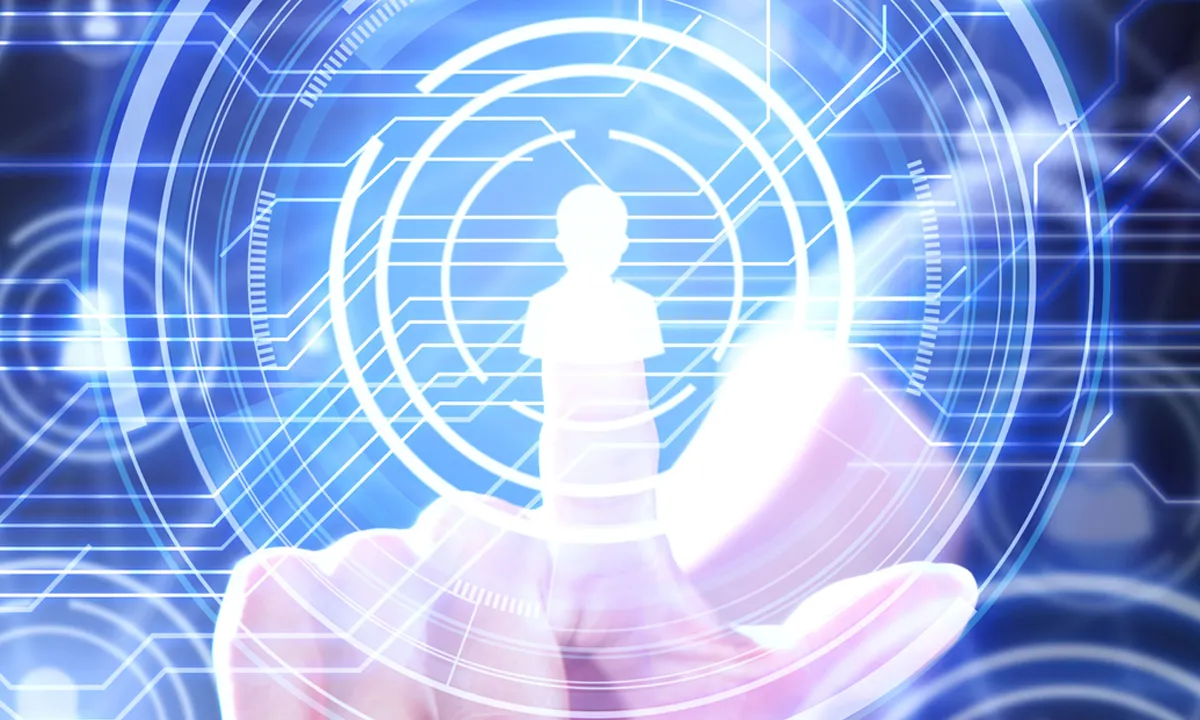
Social Network Analysis

Introduction to Systematic Review and Meta-Analysis
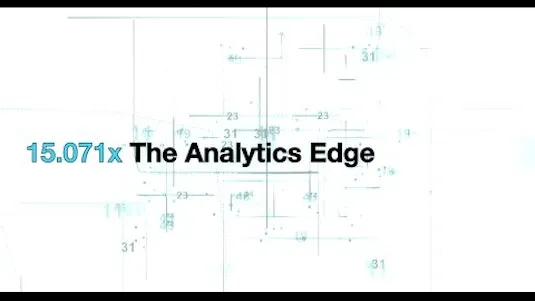
The Analytics Edge

DCO042 - Python For Informatics

Causal Diagrams: Draw Your Assumptions Before Your Conclusions
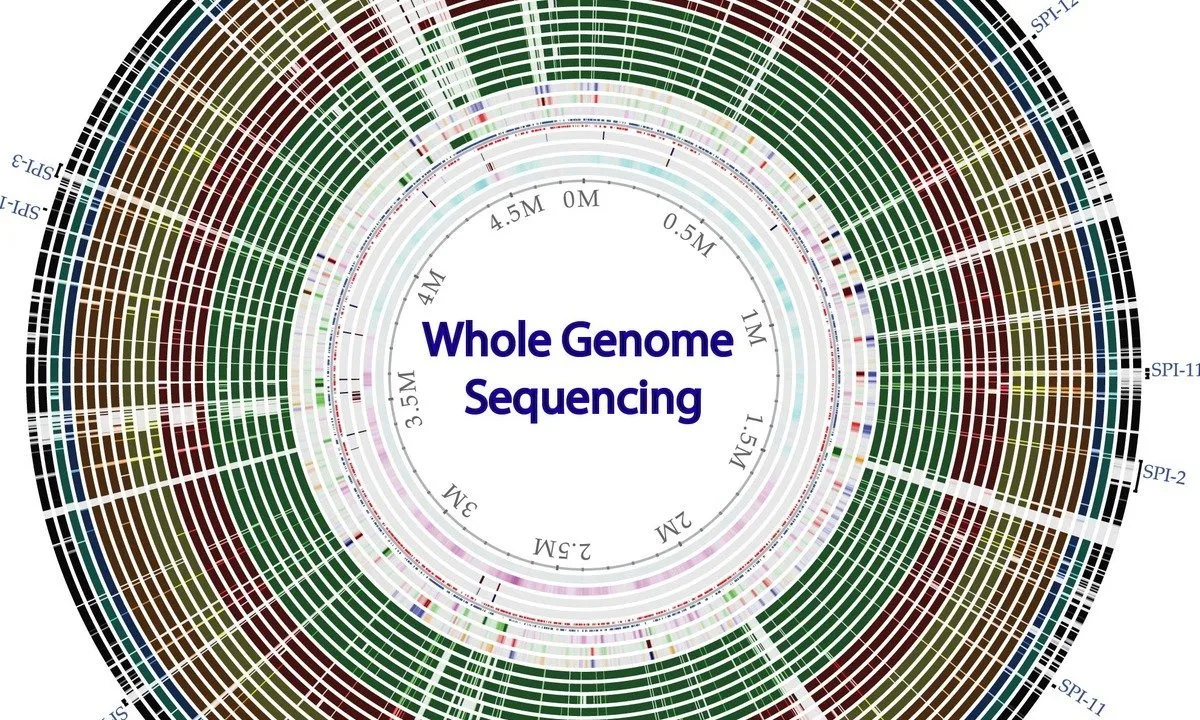
Whole genome sequencing of bacterial genomes - tools and applications
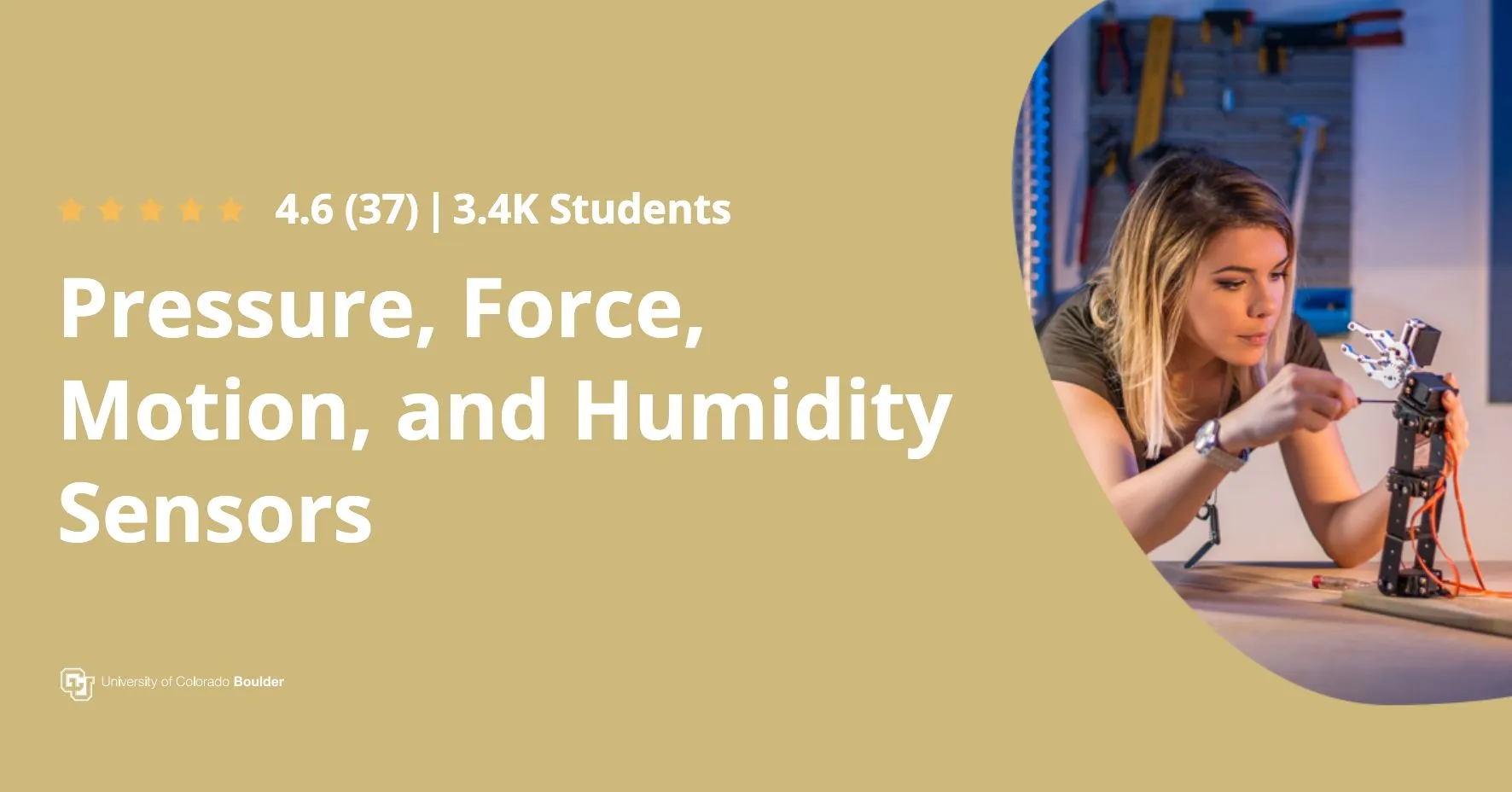
Pressure Force Motion and Humidity Sensors
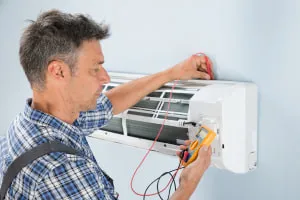
Appliance Testing Fundamentals

Start your review of Convolution