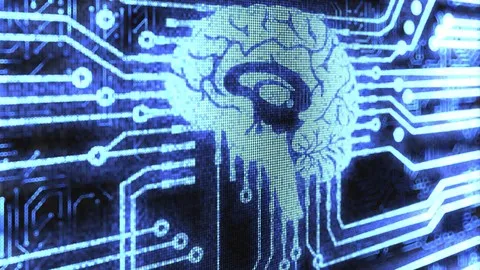
Data Science: Supervised Machine Learning in Python





Learn supervised machine learning in Python with this comprehensive course! Explore K-Nearest Neighbor, Naive Bayes Classifier, Decision Tree, and Perceptron algorithms. Discover hyperparameters, cross-validation, feature extraction, feature selection, and multiclass classification. Plus, learn to write a web service that runs a machine learning model and makes predictions. ▼
ADVERTISEMENT
Course Feature
Cost:
Paid
Provider:
Udemy
Certificate:
No Information
Language:
English
Start Date:
Self Paced
Course Overview
❗The content presented here is sourced directly from Udemy platform. For comprehensive course details, including enrollment information, simply click on the 'Go to class' link on our website.
Updated in [June 30th, 2023]
This course provides an introduction to supervised machine learning in Python. It covers the K-Nearest Neighbor algorithm, Naive Bayes Classifier, General Bayes Classifier, Decision Tree algorithm, and Perceptron algorithm. It also covers topics such as hyperparameters, cross-validation, feature extraction, feature selection, and multiclass classification. Additionally, the course will discuss the Sci-Kit Learn library and provide a real-world example of writing a web service that runs a machine learning model and makes predictions.
[Applications]
Upon completion of this course, participants will be able to apply the supervised machine learning algorithms they have learned in Python to real-world problems. They will be able to use the Sci-Kit Learn library to optimize their code and make predictions with their models. Additionally, they will be able to create web services that run machine learning models and make predictions.
[Career Paths]
The career path recommended to learners of this course is Data Scientist. A Data Scientist is a professional who uses data to gain insights and solve complex problems. They use a variety of techniques such as machine learning, statistical analysis, and data mining to analyze large datasets and uncover patterns and trends. They also use their knowledge of programming languages such as Python, R, and SQL to develop algorithms and models that can be used to make predictions and decisions.
The development trend of Data Science is towards automation and artificial intelligence. Data Scientists are increasingly using machine learning and deep learning algorithms to automate the process of data analysis and make predictions. They are also using natural language processing and computer vision to develop systems that can understand and interpret data. As the technology advances, Data Scientists will be able to use more sophisticated algorithms and models to gain insights from data.
[Education Paths]
The recommended educational path for learners of this course is to pursue a degree in Data Science. This degree typically involves courses in mathematics, computer science, and statistics, as well as courses in machine learning and artificial intelligence. Students will learn the fundamentals of data science, including data collection, analysis, and visualization, as well as supervised machine learning algorithms such as K-Nearest Neighbor, Naive Bayes Classifier, Decision Tree, and Perceptron. They will also learn about hyperparameters, cross-validation, feature extraction, feature selection, and multiclass classification. Additionally, they will learn about the Sci-Kit Learn library and how to write a web service that runs a machine learning model and makes predictions.
The development trend of data science degrees is to focus more on the practical applications of data science, such as machine learning and artificial intelligence. This means that students will be expected to have a deeper understanding of the algorithms and techniques used in data science, as well as the ability to apply them to real-world problems. Additionally, data science degrees are increasingly focusing on the ethical implications of data science, such as data privacy and security.
Pros & Cons
Well-organized and covers all machine learning algorithms.
Clear explanations and examples for beginners.
Extensive course with projects and exercises for practical learning.
Instructor explains the holistic view and math concepts well.
Bottom-up approach is helpful for understanding from scratch.
Topics are well-explained with illustrations and demos.
Provides a great learning experience for those interested in becoming experts in machine learning.
Does not cover deep learning.
Coding level is not applied, requires additional practice.
Some users may find the course too basic.
Course Provider
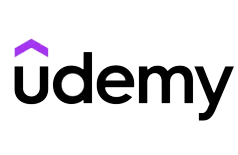
Provider Udemy's Stats at AZClass
Discussion and Reviews
0.0 (Based on 0 reviews)
Explore Similar Online Courses
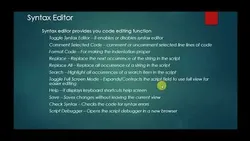
ServiceNow Scripting & Advance
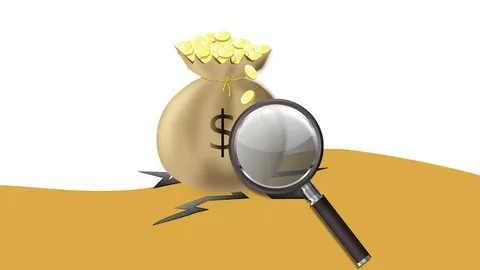
Level I CFA Prep Course (2023) - Fixed Income
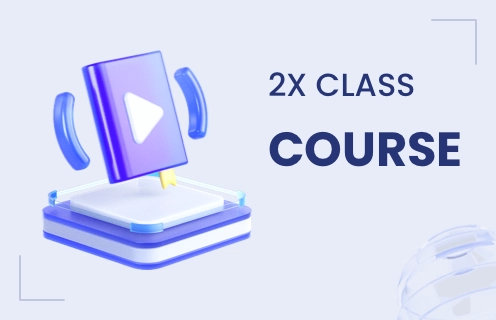
Python for Informatics: Exploring Information
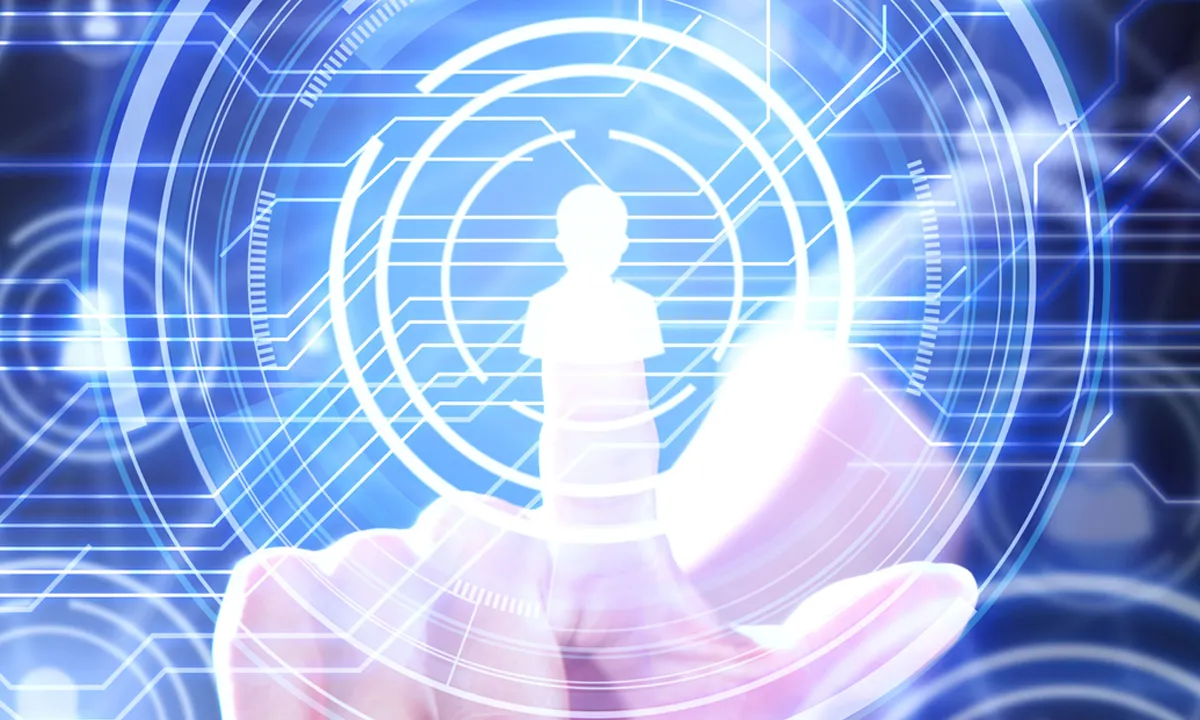
Social Network Analysis

Introduction to Systematic Review and Meta-Analysis
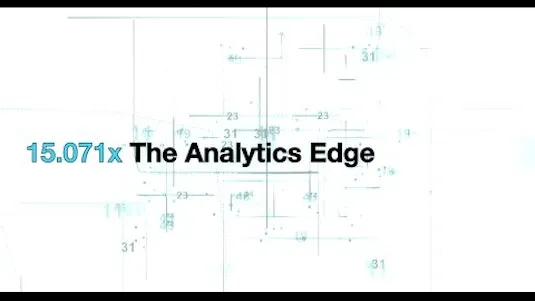
The Analytics Edge

DCO042 - Python For Informatics

Causal Diagrams: Draw Your Assumptions Before Your Conclusions
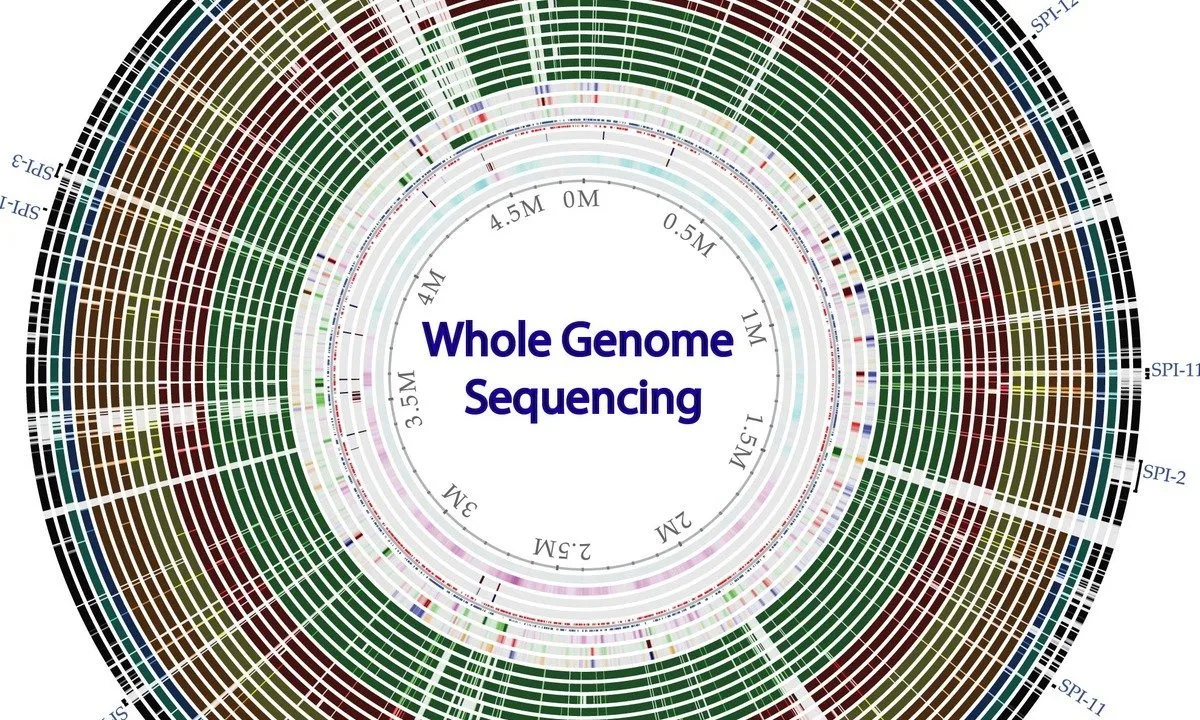
Whole genome sequencing of bacterial genomes - tools and applications
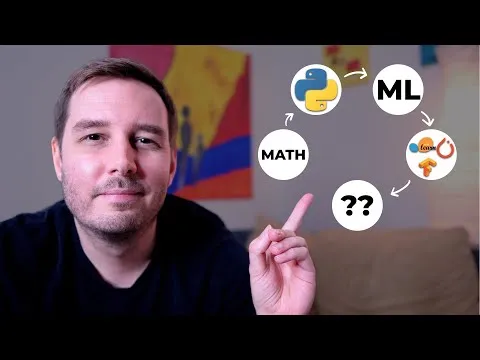
How I would learn Machine Learning (if I could start over)
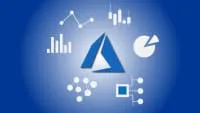
Learn Data Science and Machine Learning on Microsoft Azure
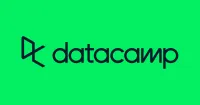
Start your review of Data Science: Supervised Machine Learning in Python