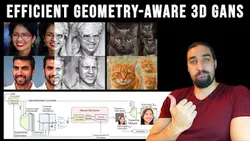
Efficient Geometry-aware 3D Generative Adversarial Networks GAN Paper Explained





Discover the power of 3D Generative Adversarial Networks (GANs) with this comprehensive paper explanation. Learn about tri-plane 3D scene representation, NeRF in depth, explicit voxel grid methods, pose correlated facial features, dual discrimination, and super-resolution. Explore ethical considerations and robustness of intrinsics and extrinsics. Get started now! ▼
ADVERTISEMENT
Course Feature
Cost:
Free
Provider:
Youtube
Certificate:
Paid Certification
Language:
English
Start Date:
On-Demand
Course Overview
❗The content presented here is sourced directly from Youtube platform. For comprehensive course details, including enrollment information, simply click on the 'Go to class' link on our website.
Updated in [June 30th, 2023]
This course provides an overview of the efficient geometry-aware 3D Generative Adversarial Networks (GAN) paper. It begins with an introduction to the tri-plane 3D scene representation and an in-depth look at NeRF. It then covers explicit voxel grid methods and explains the tri-plane 3D scene representation. It also covers the efficient 3D GANs pipeline, pose correlated facial features, dual discrimination, and super-resolution. The course then discusses the results, ethical considerations, and robustness of intrinsics and extrinsics. Finally, it concludes with an outro.
[Applications]
The application of this course can be seen in the development of 3D generative adversarial networks (GANs) for efficient geometry-aware 3D scene representation. This course provides an in-depth explanation of the NeRF algorithm, as well as an overview of explicit voxel grid methods. It also explains the advantages of using the tri-plane 3D scene representation, which is as expressive as the voxel grid methods. Additionally, this course covers the efficient 3D GANs pipeline, pose correlated facial features, and dual discrimination and super-resolution techniques. Finally, it provides ethical considerations and an assessment of the robustness of intrinsics and extrinsics.
[Career Paths]
The career path recommended to learners from this course is a 3D Generative Adversarial Networks (GAN) Engineer. This job position involves developing and deploying 3D GANs to generate realistic 3D scenes from a given set of input data. The engineer must be knowledgeable in 3D scene representation, NeRF, explicit voxel grid methods, dual discrimination, and super-resolution. They must also be able to understand the ethical considerations of deploying 3D GANs and be able to assess the robustness of the generated 3D scenes.
The development trend for 3D GANs is rapidly increasing as more and more applications are being developed that require realistic 3D scenes. This includes applications in virtual reality, augmented reality, gaming, and robotics. As the demand for 3D GANs increases, the need for engineers with the necessary skills to develop and deploy them will also increase. Therefore, the development trend for 3D GANs is expected to continue to grow in the near future.
[Education Paths]
The recommended educational path for learners interested in Geometry-aware 3D Generative Adversarial Networks (GANs) is to pursue a degree in Computer Science or a related field. This degree should include courses in 3D modeling, computer graphics, machine learning, and artificial intelligence. Additionally, courses in mathematics, physics, and engineering may be beneficial.
The development trend for this field is to focus on creating more efficient GANs pipelines, as well as exploring the ethical considerations of using GANs in 3D modeling. Additionally, research into the robustness of GANs in terms of intrinsics and extrinsics is becoming increasingly important. Finally, research into the use of pose-correlated facial features in GANs is also an area of interest.
Course Provider
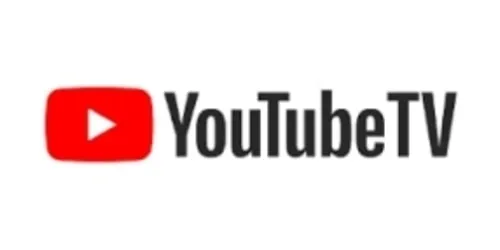
Provider Youtube's Stats at AZClass
Discussion and Reviews
0.0 (Based on 0 reviews)
Explore Similar Online Courses
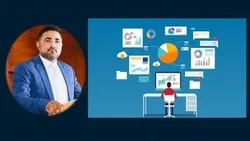
Power BI Dashboard Step by Step

Excel: Skills Development & Training
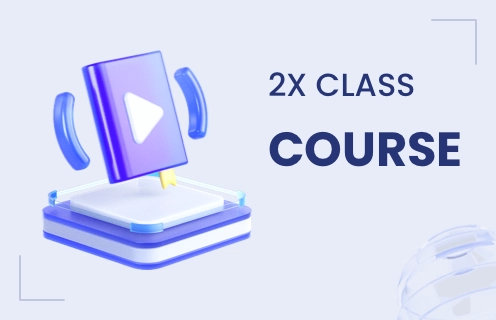
Python for Informatics: Exploring Information
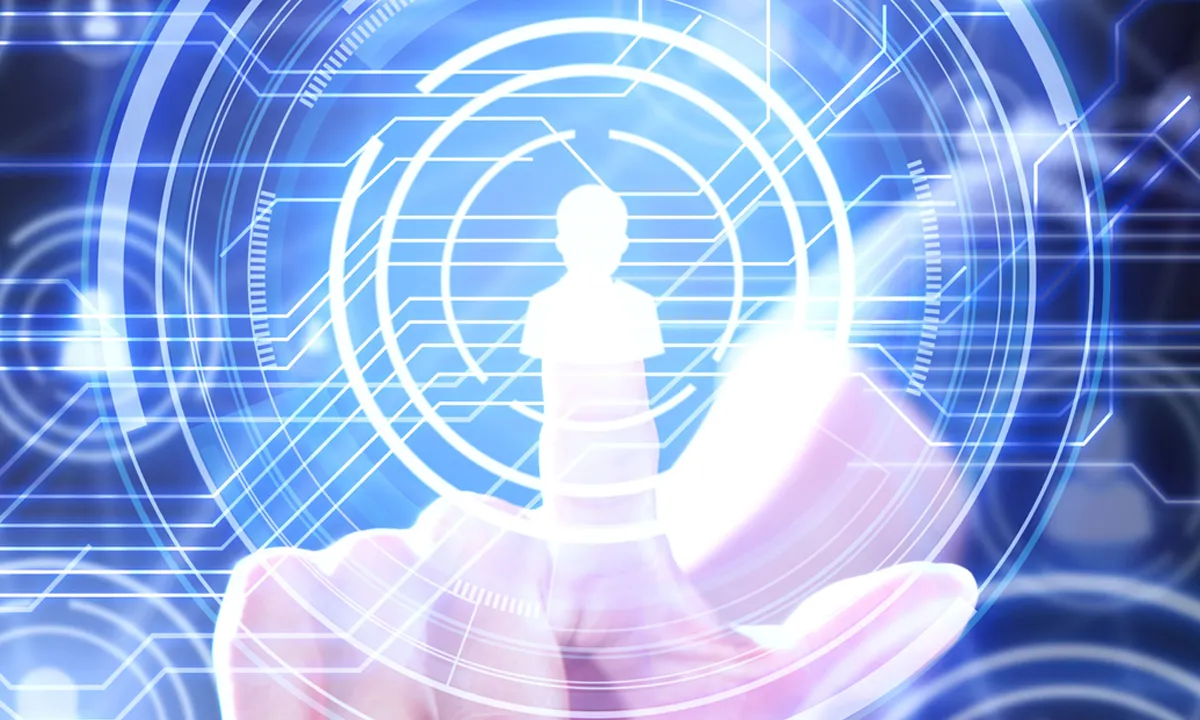
Social Network Analysis

Introduction to Systematic Review and Meta-Analysis
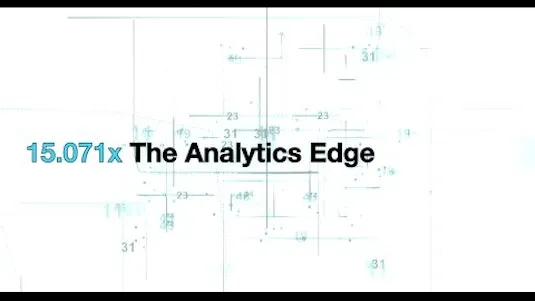
The Analytics Edge

DCO042 - Python For Informatics

Causal Diagrams: Draw Your Assumptions Before Your Conclusions
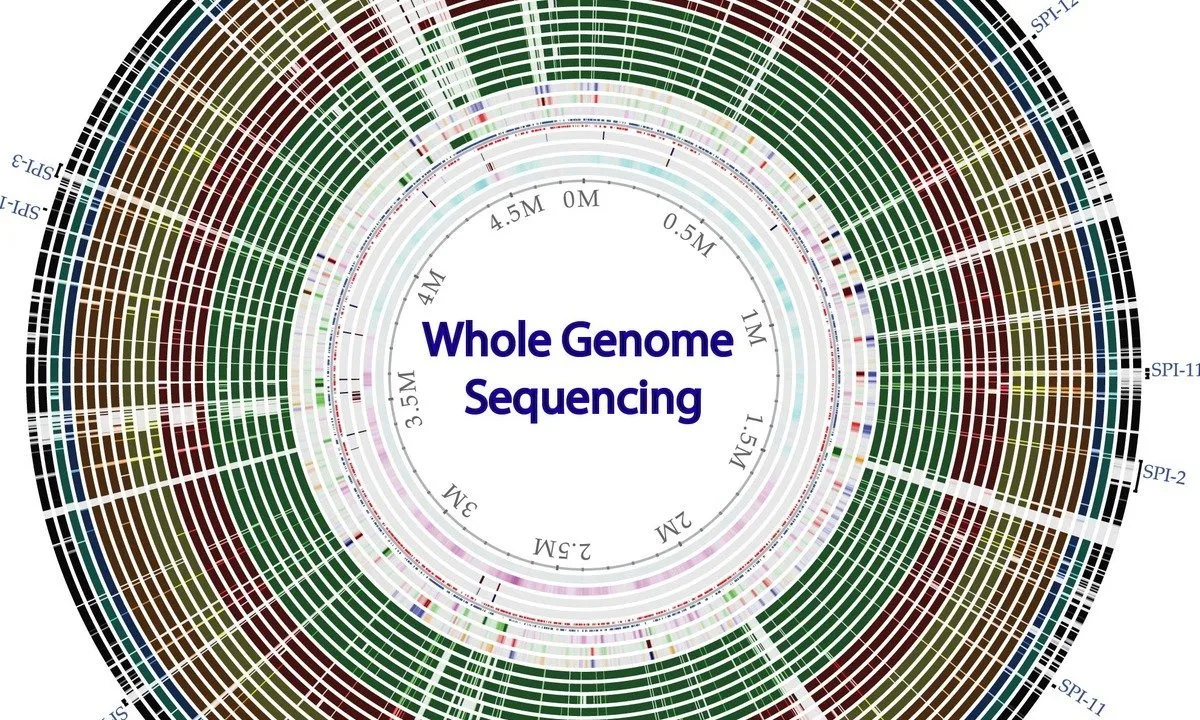
Whole genome sequencing of bacterial genomes - tools and applications
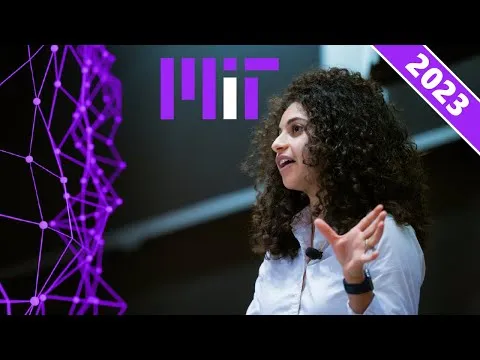
MIT 6S191: Recurrent Neural Networks Transformers and Attention
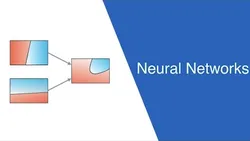
Neural Networks

Start your review of Efficient Geometry-aware 3D Generative Adversarial Networks GAN Paper Explained