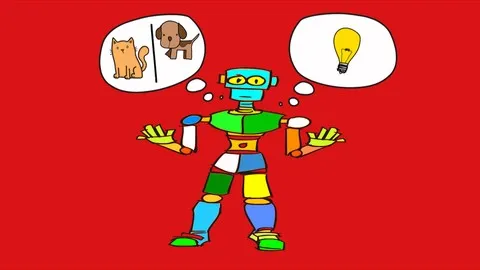
From 0 to 1: Machine Learning NLP & Python-Cut to the Chase





This course is designed to help you quickly learn the fundamentals of Machine Learning, Natural Language Processing & Python. From 0 to 1, you'll learn supervised/unsupervised learning, classification, clustering, association detection, anomaly detection, dimensionality reduction, regression, Naive Bayes, K-nearest neighbours, support vector machines, artificial neural networks, K-means, hierarchical clustering, principal components analysis, linear regression, logistics regression, random variables, Bayes theorem, bias-variance tradeoff, corpora, stopwords, sentence and word parsing, auto-summarization, sentiment analysis, TF-IDF, document distance, text summarization, text classification, decision trees, overfitting, ensemble learning, random forests, content based filtering, collaborative filtering, association rules learning and more. Learn why it's useful and how to apply it to real-world problems. ▼
ADVERTISEMENT
Course Feature
Cost:
Paid
Provider:
Udemy
Certificate:
No Information
Language:
English
Start Date:
Self Paced
Course Overview
❗The content presented here is sourced directly from Udemy platform. For comprehensive course details, including enrollment information, simply click on the 'Go to class' link on our website.
Updated in [June 30th, 2023]
This course, From 0 to 1: Machine Learning NLP & Python-Cut to the Chase, provides an overview of the fundamentals of Machine Learning, Natural Language Processing (NLP) and Python. It covers topics such as supervised and unsupervised learning, classification, clustering, association detection, anomaly detection, dimensionality reduction, regression, Naive Bayes, K-nearest neighbours, support vector machines, artificial neural networks, K-means, hierarchical clustering, principal components analysis, linear regression, logistics regression, random variables, Bayes theorem, bias-variance tradeoff, corpora, stopwords, sentence and word parsing, auto-summarization, sentiment analysis, TF-IDF, document distance, text summarization, text classification with Naive Bayes and K-Nearest Neighbours and clustering with K-Means. It also covers why it’s useful, approaches to solving rule-based, ML-based, training, feature extraction, sentiment lexicons, regular expressions, Twitter API, sentiment analysis of tweets with Python, decision trees and decision tree learning, overfitting in decision trees, techniques to mitigate overfitting (cross validation, regularization), ensemble learning and random forests, content based filtering, collaborative filtering and association rules learning. Finally, it will apply multi-layer perceptrons to the MNIST digit recognition problem.
[Applications]
The application of this course can be seen in various areas such as natural language processing, machine learning, and Python programming. Natural language processing can be used to create corpora, stopwords, sentence and word parsing, auto-summarization, sentiment analysis, TF-IDF, document distance, text summarization, and text classification. Machine learning can be used to create supervised/unsupervised learning, classification, clustering, association detection, anomaly detection, dimensionality reduction, regression, Naive Bayes, K-nearest neighbours, support vector machines, artificial neural networks, K-means, hierarchical clustering, principal components analysis, linear regression, logistics regression, random variables, Bayes theorem, and bias-variance tradeoff. Python programming can be used to create decision trees and decision tree learning, overfitting in decision trees, techniques to mitigate overfitting (cross validation, regularization), ensemble learning and random forests, content based filtering, collaborative filtering, and association rules learning. Additionally, multi-layer perceptrons can be applied to the MNIST digit recognition problem.
[Career Paths]
The career path recommended to learners of this course is Machine Learning NLP & Python Developer. This job position involves developing and deploying machine learning models and algorithms to solve complex problems in natural language processing (NLP) and Python. The developer will be responsible for designing, coding, testing, and maintaining software applications that use machine learning and NLP techniques. They will also be responsible for researching and developing new algorithms and techniques to improve the accuracy and efficiency of the software.
The development trend for this job position is to focus on the use of deep learning and artificial intelligence (AI) to improve the accuracy and efficiency of machine learning models and algorithms. Deep learning and AI techniques are becoming increasingly popular in the field of NLP and Python, and developers must be able to use these techniques to create more accurate and efficient software applications. Additionally, developers must be able to use the latest tools and technologies to create more efficient and accurate software applications.
[Education Paths]
The recommended educational path for learners is to pursue a degree in Machine Learning and Natural Language Processing (NLP). This degree will provide learners with the knowledge and skills to develop and apply machine learning algorithms to solve real-world problems. Learners will gain an understanding of supervised and unsupervised learning, classification, clustering, association detection, anomaly detection, dimensionality reduction, regression, naive bayes, k-nearest neighbours, support vector machines, artificial neural networks, k-means, hierarchical clustering, principal components analysis, linear regression, logistics regression, random variables, bayes theorem, bias-variance tradeoff, corpora, stopwords, sentence and word parsing, auto-summarization, sentiment analysis, TF-IDF, document distance, text summarization, text classification, decision trees, decision tree learning, overfitting in decision trees, techniques to mitigate overfitting, ensemble learning, random forests, content based filtering, collaborative filtering, and association rules learning.
The development trend of this degree is to focus on the application of machine learning and NLP to solve real-world problems. This includes the development of algorithms to process large datasets, the use of deep learning to improve accuracy and efficiency, and the development of applications to automate tasks. Additionally, the degree will focus on the ethical implications of machine learning and NLP, such as data privacy and security.
Pros & Cons
Well-balanced content of explanations and hands-on practice.
Clear and engaging delivery of course content.
Ability to explain complex topics in a clear and understandable manner.
Concise and practical approach to machine learning.
Organized and digestible segments for complex theory.
Provides a good introduction to the concepts of machine learning and NLP.
Enables learners to develop a deep understanding of the subject overall.
Code examples during the NLP part do not work.
False statement about not needing programming experience.
Lack of coverage on Neural Network and Google Tensor Flow.
Some sections of the course are becoming dated.
Lectures working through the code need a slower pace and deeper explanation.
Lack of responsiveness from the instructor in answering questions.
Repetition in the course content.
Course Provider
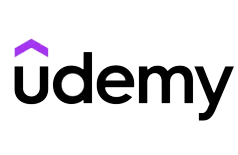
Provider Udemy's Stats at AZClass
Discussion and Reviews
0.0 (Based on 0 reviews)
Explore Similar Online Courses
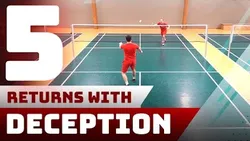
Badminton Deception Shots
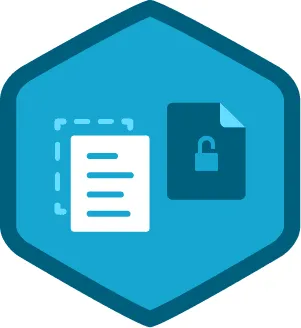
REST API Validation with Express Course
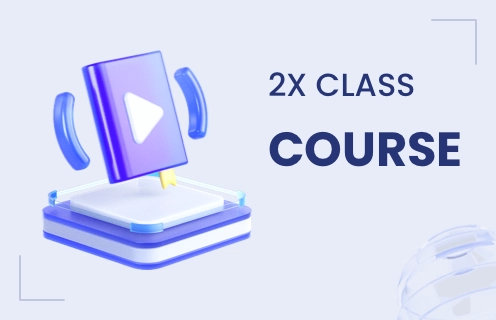
Python for Informatics: Exploring Information
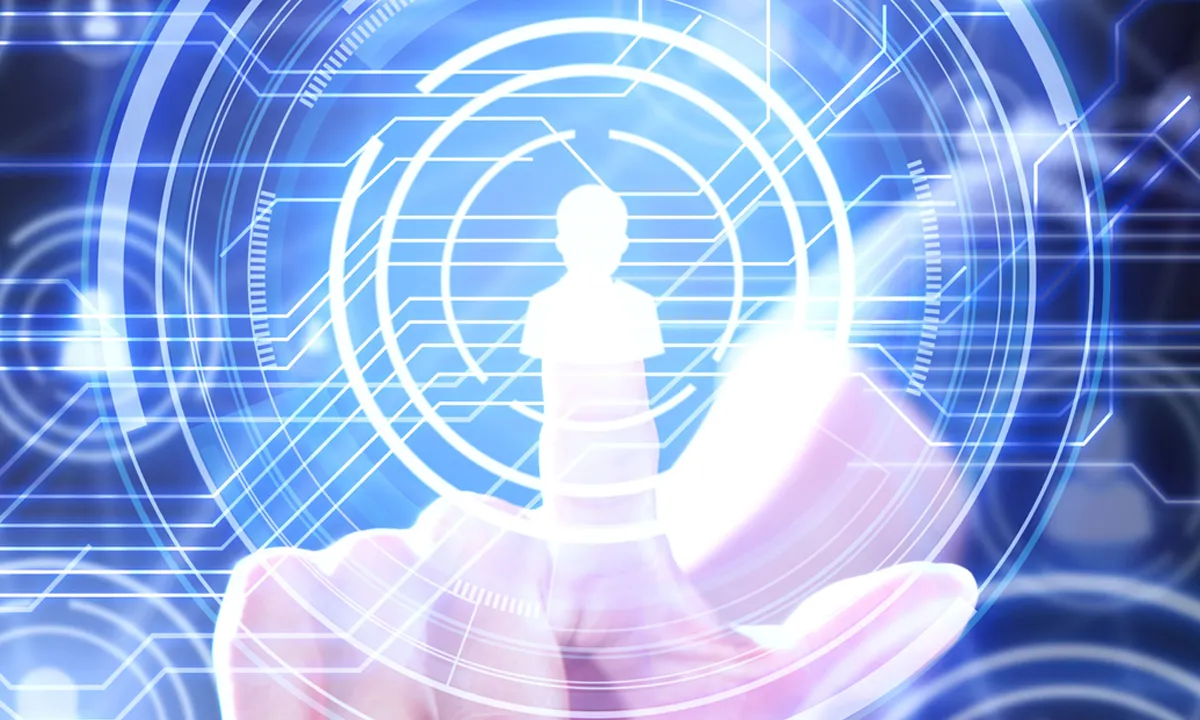
Social Network Analysis

Introduction to Systematic Review and Meta-Analysis
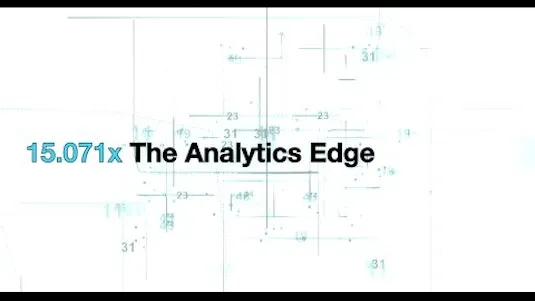
The Analytics Edge

DCO042 - Python For Informatics

Causal Diagrams: Draw Your Assumptions Before Your Conclusions
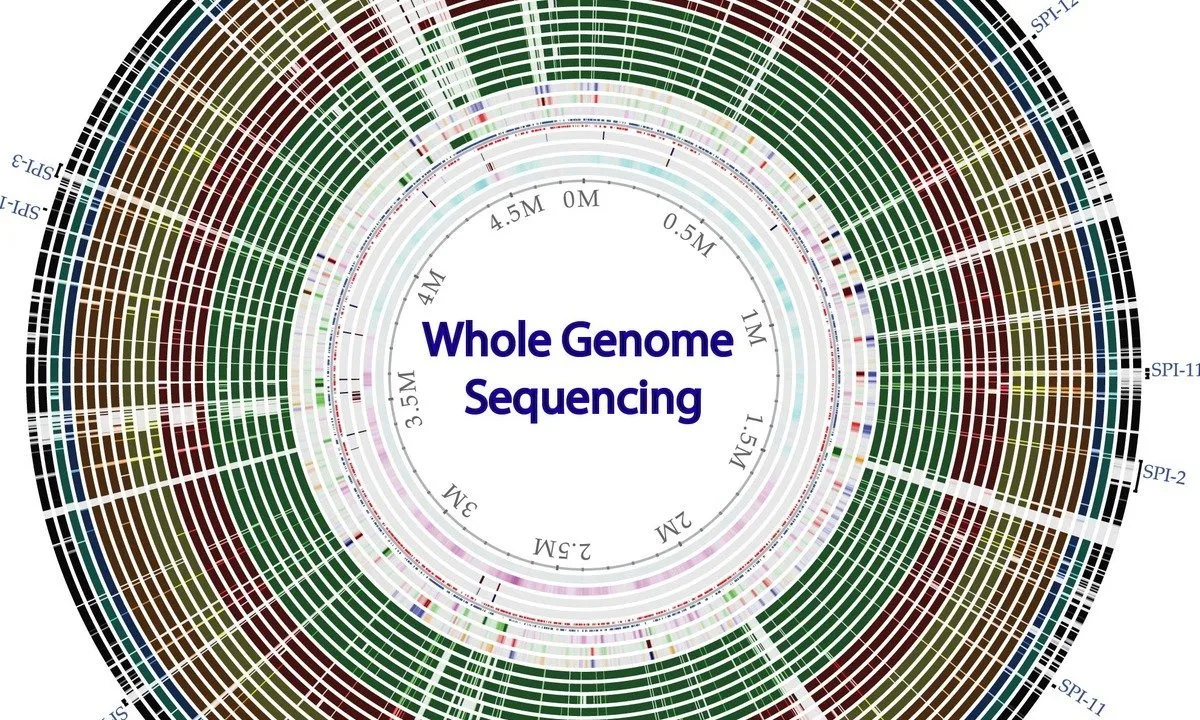
Whole genome sequencing of bacterial genomes - tools and applications
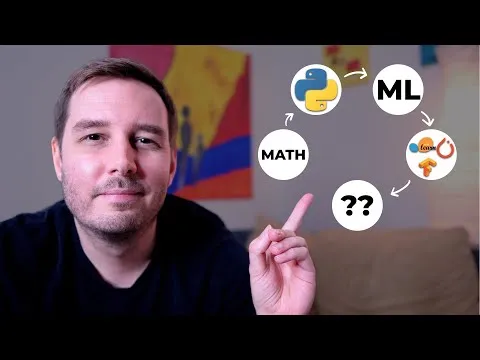
How I would learn Machine Learning (if I could start over)
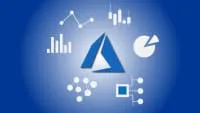
Learn Data Science and Machine Learning on Microsoft Azure
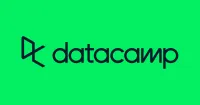
Start your review of From 0 to 1: Machine Learning NLP & Python-Cut to the Chase