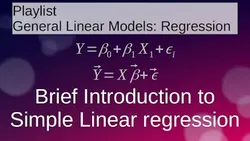
General Linear Models: Regression





This course introduces students to linear models, beginning with simple linear regression. It covers the properties of least squares estimators, estimating the residual variance, and matrix notation. It also covers multiple linear regression, diagnostics, and model selection. Finally, it covers generalized linear models and their applications. ▼
ADVERTISEMENT
Course Feature
Cost:
Free
Provider:
Youtube
Certificate:
Paid Certification
Language:
English
Start Date:
On-Demand
Course Overview
❗The content presented here is sourced directly from Youtube platform. For comprehensive course details, including enrollment information, simply click on the 'Go to class' link on our website.
Updated in [February 21st, 2023]
Introduction to Linear Models
Simple Linear Regression
Simple Linear Regression: Properties of Least Squares Estimators
Simple Linear Regression: Estimating the Residual Variance
Simple Linear regression: Matrix Notation
Simple Linear Regression: Maximum Likelihood Estimation
Simple Linear Regression: Partitioning Total Variability
Simple Linear Regression: Matrix Notation for Sum of Squares
Simple Linear Regression: ANOVA Table
Simple Linear Regression: Testing the Model is Useful
Simple Linear Regression: LSEs are Normally Distributed
Simple Linear Regression: Confidence intervals for Beta Parameters
Simple Linear Regression: Coefficient of Determination
Simple Linear Regression:Confidence and Prediction Intervals on the Mean and Individual Response
Simple Linear Regression: Simultaneous Inference on B0 and B1
Simple Linear Regression: Bonferroni and Working-Hotelling Adjustments
Simple Linear Regression: Residuals and their Properties
Simple Linear Regression: X and Y Random
Simple Linear Regression: Test for the Correlation Coefficient
Simple Linear Regression: Fixed Zero Intercept Model
Multiple Linear Regression: Introduction
Multiple Linear Regression: Least Squares Estimates
Multiple Linear Regression: The Hat Matrix
Multiple Linear Regression: Estimating the Error Variance
Multiple Linear Regression: Projection and Idempotent Matrices
Multiple Linear Regression: Gauss Markov Theorem
Multiple Linear Regression: Partitioning Total Variability
Multiple Linear Regression: Type I Sum of Squares
Multiple Linear Regression: Type II Sum of Squares
Multiple Linear Regression: Global F Test
Multiple Linear Regression: Partial F Tests
Multiple Linear Regression: t Tests for a Single Beta Parameter
Multiple Linear Regression: General Linear Hypotheses
Using R: Simple Linear Regression from Scratch
Multiple Linear Regression: CI&PI on the Mean and Individual Response
Multiple Linear Regression: Simultaneous Inference of B(B0B1 Bk)
Multiple Linear Regression: Partitioning the Residual Sum of Squares
Multiple Linear Regression: Repeated Observations and Lack of Fit Test
Multiple Linear Regression: Centering and Scaling the Design Matrix
Multiple Linear Regression: Condition Number & Multicollinearity
Multiple Linear Regression: Variance Inflation Factor (VIF) & Multicollinearity
Multiple Linear Regression: Variance Proportions & Multicollinearity
Multiple Linear Regression: Indicator & Dummy Variables
Multiple Linear Regression: AIC (Akaike Information Criterion)
Multiple Linear Regression: Choosing a model with R2 Adjusted R2 and MSE
Multiple Linear Regression: Mallows Cp
Multiple Linear Regression: Impact of Under or Over Fitting a Model
Multiple Linear Regression: The PRESS Prediction SS Statistic
Multiple Linear Regression: Residual Properties
Weighted Least Squares Regression: Mahalanobis Distance
Weighted Least Squares Regression: Hat Matrix
Weighted Least Squares Regression: Estimability & BLUE
Weighted Least Squares Regression: Estimating the Error Variance
Weighted Least Squares Regression: Testing for Estimable Functions
Weighted Least Squares Regression: Partial F Tests
Multiple Linear Regression: Canonical Form
Multiple Linear Regression: Canonical Form and Multicollinearity
Multiple Linear Regression: Principal Components Model
Ridge Regression (part 1 of 4): Variance Reduction
Ridge Regression (part 2 of 4): Deriving the Bias
Ridge Regression (part 3 of 4): Deriving from 1st principles
Ridge Regression (part 4 of 4): Canonical Form
Multiple Linear Regression: Box-Cox Transformation
Multiple Linear Regression: Box - Tidwell Transformation
Multiple Linear Regression: Studentized Residuals (Part 1 of 2)
Multiple Linear Regression: Studentized Residuals (Part 2 of 2)
Multiple Linear Regression: Partial Regression Plots (Added Variable Plots)
Multiple Linear Regression: Influence Measures (Part 1 of 2)
Multiple Linear Regression: Influence Measures (Part 2 of 2)
Best quadratic unbiased estimator of variance in a MLR model using Lagrange Multipliers
Course Provider
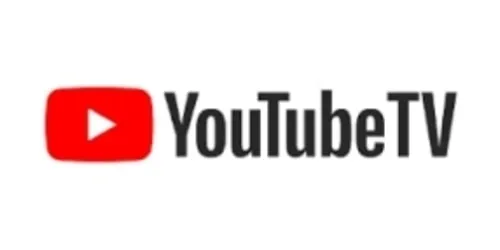
Provider Youtube's Stats at AZClass
Discussion and Reviews
0.0 (Based on 0 reviews)
Explore Similar Online Courses
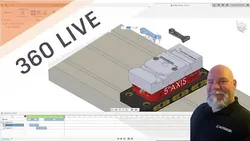
Rendering & Graphics

Learn Fusion 360 in 30 days for Complete Beginners! (Part 2 of 3)
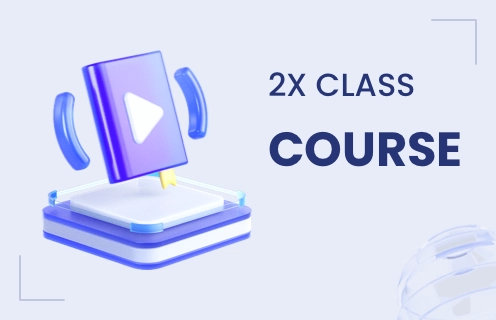
Python for Informatics: Exploring Information
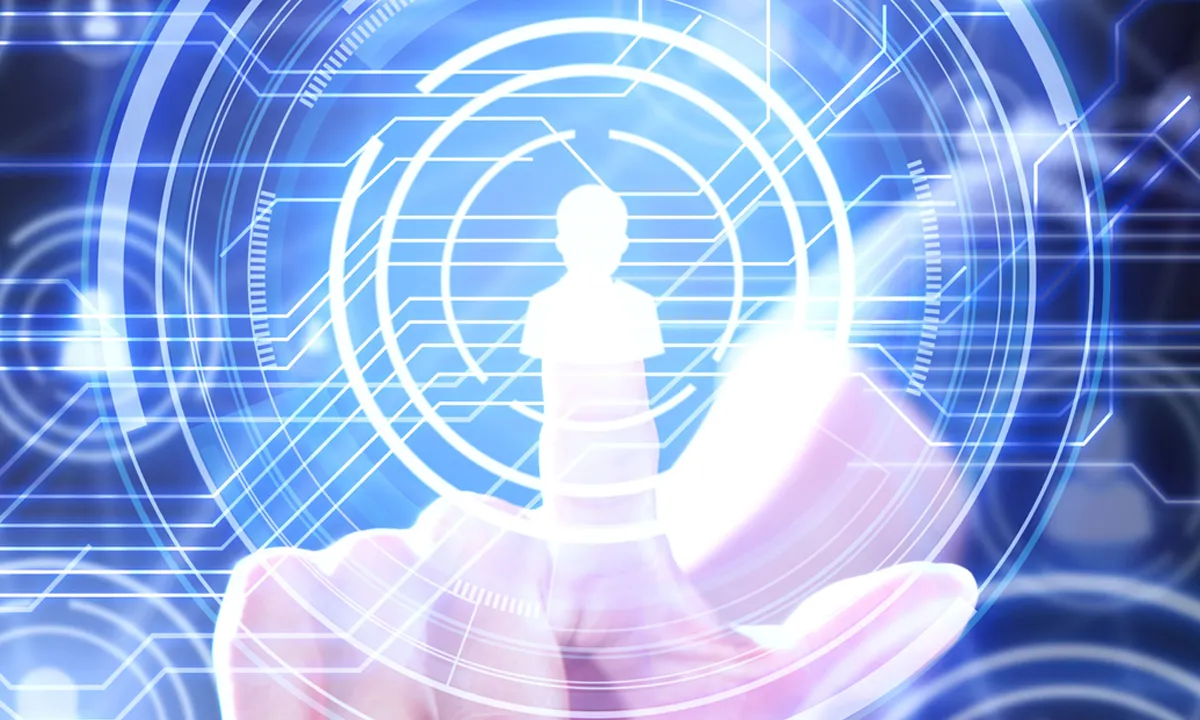
Social Network Analysis

Introduction to Systematic Review and Meta-Analysis
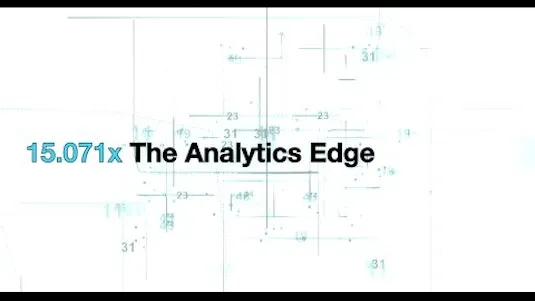
The Analytics Edge

DCO042 - Python For Informatics

Causal Diagrams: Draw Your Assumptions Before Your Conclusions
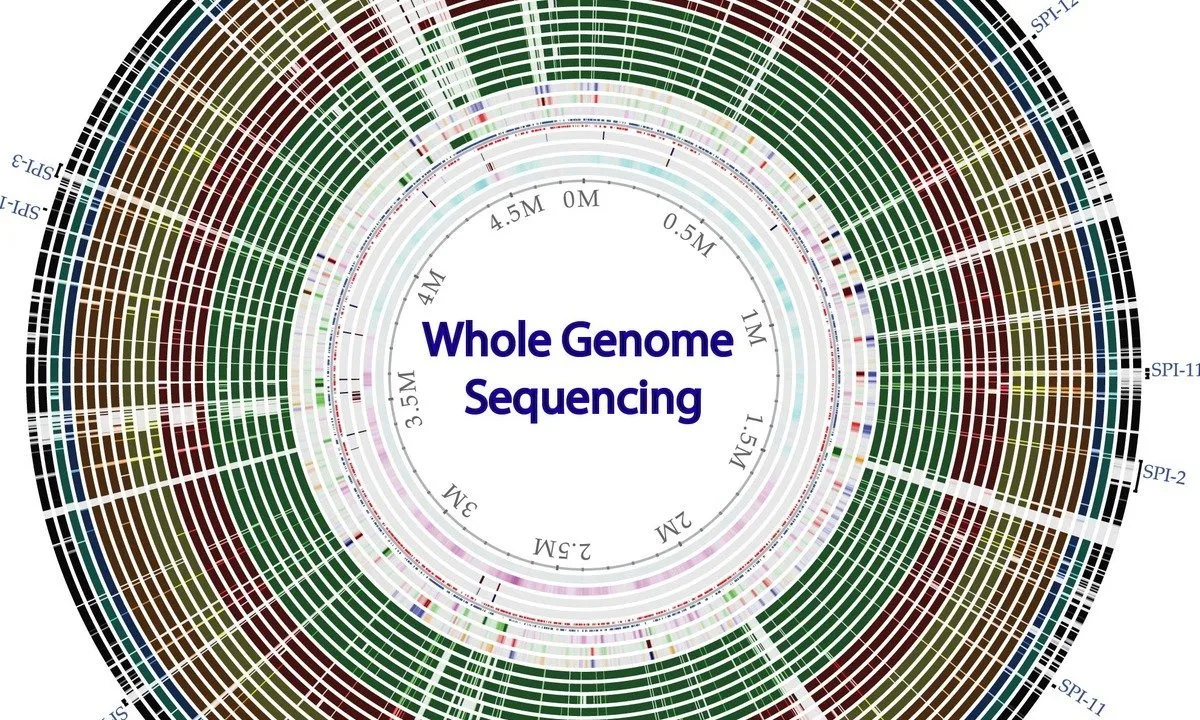
Whole genome sequencing of bacterial genomes - tools and applications
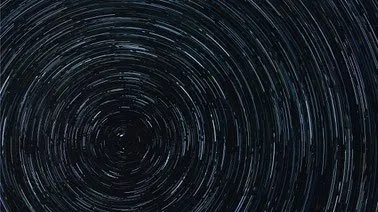
Data Science: Linear Regression

Simple Linear Regression for the Absolute Beginner
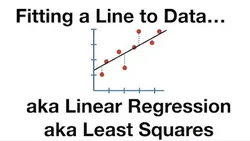
Start your review of General Linear Models: Regression