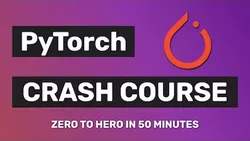
PyTorch Crash Course - Getting Started with Deep Learning




This course provides an introduction to deep learning using PyTorch. It covers installation and overview, tensor basics, autograd, linear regression, autograd model, loss and optimizer, neural network, convolutional neural network, recurrent neural network, and transfer learning. It is designed to help learners understand the fundamentals of deep learning and how to use PyTorch to build and train models. ▼
ADVERTISEMENT
Course Feature
Cost:
Free
Provider:
Youtube
Certificate:
Paid Certification
Language:
English
Start Date:
On-Demand
Course Overview
❗The content presented here is sourced directly from Youtube platform. For comprehensive course details, including enrollment information, simply click on the 'Go to class' link on our website.
Updated in [February 21st, 2023]
This PyTorch Crash Course is designed to provide an introduction to deep learning using the PyTorch library. Participants will learn the basics of installation and overview of PyTorch, tensor basics, autograd, linear regression autograd, model, loss and optimizer, neural network and convolutional neural net. By the end of the course, participants will have a better understanding of the fundamentals of deep learning and how to use PyTorch to build and train models.
[Applications]
After completing this course, students can apply their knowledge of PyTorch to create deep learning models for various applications. They can use the concepts of Tensor Basics, Autograd, Linear Regression Autograd, Model, Loss & Optimizer, Neural Network, and Convolutional Neural Net to build and train models for image classification, natural language processing, and other tasks. Additionally, they can use PyTorch to deploy their models to production and use them in real-world applications.
[Career Paths]
1. Deep Learning Engineer: Deep Learning Engineers are responsible for developing and deploying deep learning models. They use frameworks such as PyTorch to build and train models, and then deploy them to production. They also need to be able to troubleshoot and optimize models. This is a rapidly growing field, as deep learning is becoming increasingly popular for a variety of applications.
2. Data Scientist: Data Scientists use PyTorch to analyze and interpret data. They use the framework to build models and use them to make predictions and draw insights from data. They also need to be able to communicate their findings to stakeholders.
3. Machine Learning Engineer: Machine Learning Engineers use PyTorch to build and deploy machine learning models. They need to be able to design and implement models, as well as optimize them for performance. They also need to be able to troubleshoot and debug models.
4. AI Developer: AI Developers use PyTorch to develop and deploy AI applications. They need to be able to design and implement AI algorithms, as well as optimize them for performance. They also need to be able to troubleshoot and debug AI applications.
[Education Paths]
1. Bachelor of Science in Computer Science: This degree path provides a comprehensive overview of computer science fundamentals, including programming, algorithms, data structures, and software engineering. It also covers topics such as artificial intelligence, machine learning, and deep learning. This degree is ideal for those interested in developing and applying cutting-edge technologies to solve complex problems.
2. Master of Science in Artificial Intelligence: This degree path focuses on the development and application of artificial intelligence technologies, such as machine learning, deep learning, and natural language processing. It covers topics such as data mining, computer vision, and robotics. This degree is ideal for those interested in developing and deploying AI-based solutions to real-world problems.
3. Doctor of Philosophy in Machine Learning: This degree path focuses on the development and application of machine learning algorithms and techniques. It covers topics such as supervised and unsupervised learning, reinforcement learning, and neural networks. This degree is ideal for those interested in researching and developing advanced machine learning algorithms and applications.
4. Master of Science in Data Science: This degree path focuses on the development and application of data science technologies, such as data mining, data visualization, and predictive analytics. It covers topics such as data wrangling, data analysis, and machine learning. This degree is ideal for those interested in leveraging data to gain insights and make decisions.
Course Provider
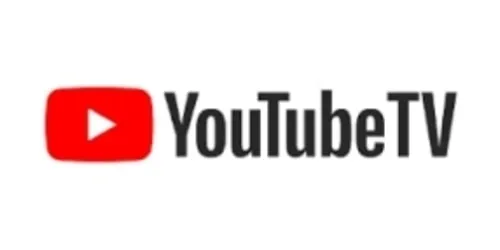
Provider Youtube's Stats at AZClass
Discussion and Reviews
0.0 (Based on 0 reviews)
Explore Similar Online Courses
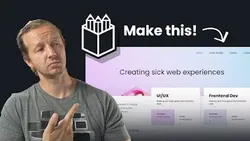
PenPot Tutorial - The Open Source UI&UX Design App
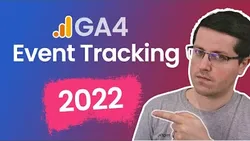
How to Track Events with Google Analytics 4 (Updated in 2022)
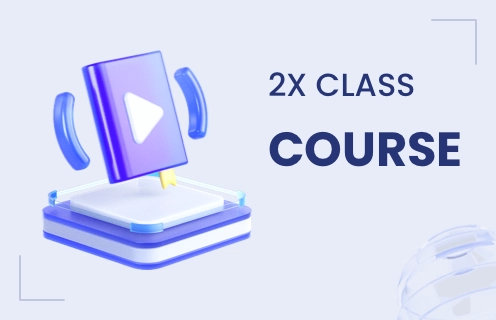
Python for Informatics: Exploring Information
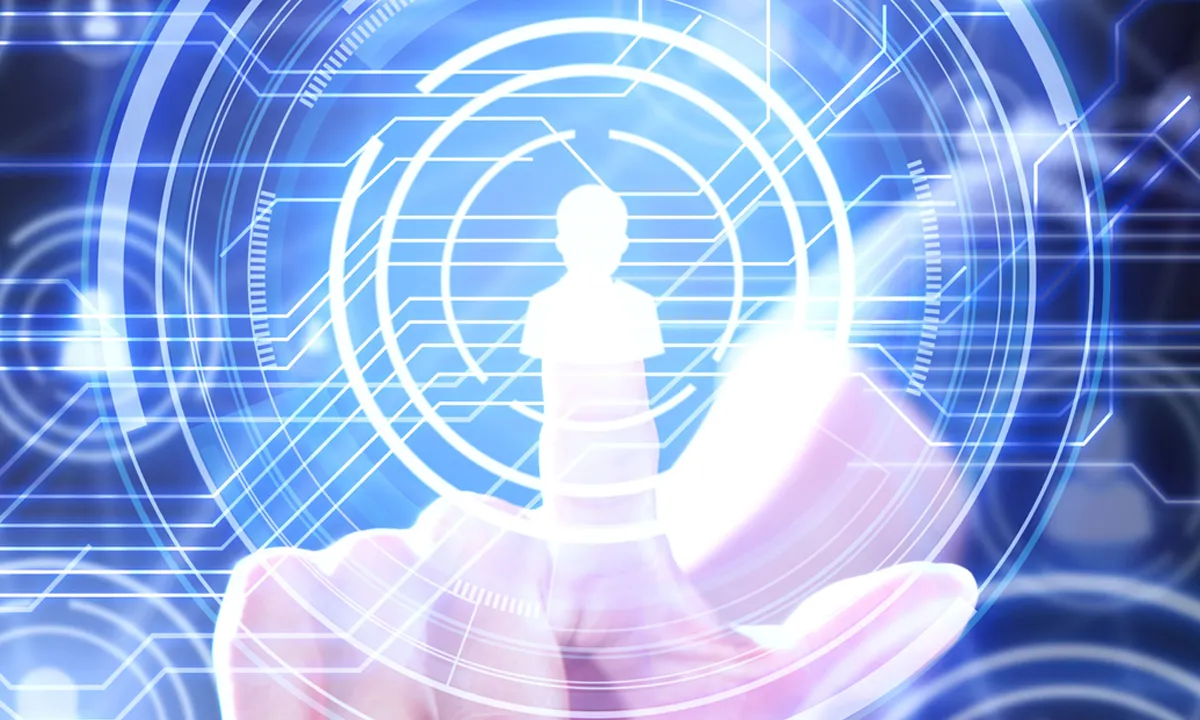
Social Network Analysis

Introduction to Systematic Review and Meta-Analysis
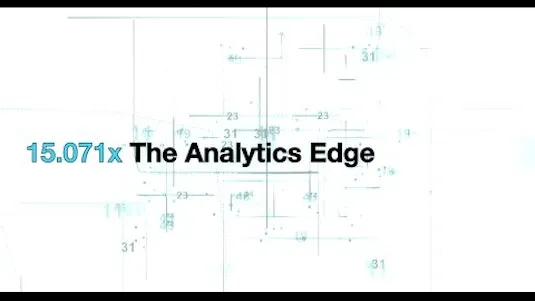
The Analytics Edge

DCO042 - Python For Informatics

Causal Diagrams: Draw Your Assumptions Before Your Conclusions
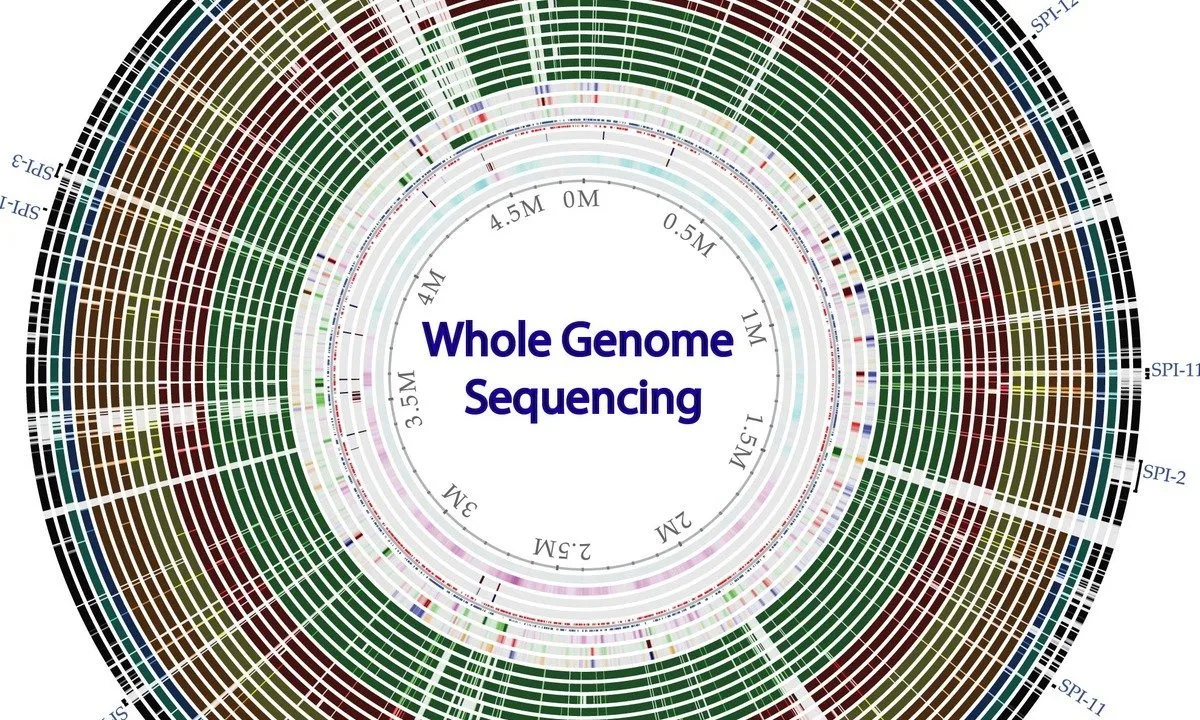
Whole genome sequencing of bacterial genomes - tools and applications
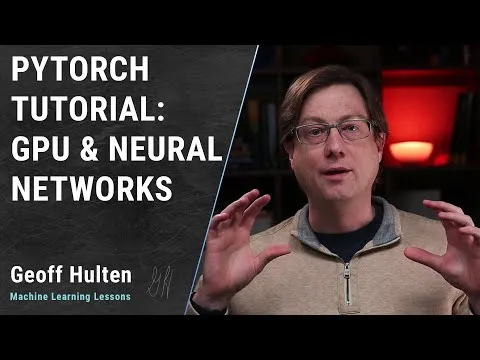
PyTorch Tutorial - Neural Networks & GPU

Applied Deep Learning with PyTorch - Full Course
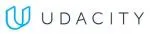
Start your review of PyTorch Crash Course - Getting Started with Deep Learning