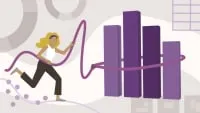
Data Wrangling in R : 2017





Data Wrangling in R: 2017 is a course that teaches users how to find and download free, public data sources on a wide range of topics. It introduces US government resources, international organisations, data search engines, web services, and language resources. After completing the course, users will be better equipped to locate the information they need for their data analysis efforts. ▼
ADVERTISEMENT
Course Feature
Cost:
Free Trial
Provider:
LinkedIn Learning
Certificate:
No Information
Language:
English
Course Overview
❗The content presented here is sourced directly from LinkedIn Learning platform. For comprehensive course details, including enrollment information, simply click on the 'Go to class' link on our website.
Updated in [June 30th, 2023]
This course, Data Wrangling in R : 2017, provides an overview of how to find free, public data sources on a wide range of business, education, and health issues, and how to download the data for your own analysis. It introduces a number of US government resources, ranging from the US Census Bureau to the US Patent and Trademark Office, and surveys datasets from international organisations such as the World Bank and the United Nations. It also goes over data search engines, web services, and even language resources like Google Books' Ngram Viewer. Upon completion of this course, students will be better equipped to locate the information they require for their scholarship and data analysis efforts.
[Applications]
After completing this course, learners can apply their knowledge of data wrangling in R to locate and analyze public data sources. They can use the resources and datasets from US government sources, international organisations, data search engines, web services, and language resources to find the information they need for their research and data analysis. Learners can also use the techniques they have learned to create their own datasets and to clean and manipulate existing datasets.
[Career Paths]
The career path recommended to learners of this course is Data Wrangling in R. Data Wrangling is the process of cleaning, transforming, and organizing data to make it easier to analyze. It involves extracting data from various sources, cleaning it, and transforming it into a format that is suitable for analysis. Data Wrangling in R involves using the R programming language to manipulate data and create visualizations.
Data Wrangling in R is a growing field, as more and more organizations are looking for ways to make sense of their data. Companies are increasingly relying on data-driven decisions, and Data Wranglers are in high demand to help them make sense of their data. Data Wranglers are expected to have a strong understanding of the R programming language, as well as a good understanding of data analysis and visualization techniques.
Data Wranglers are also expected to have a good understanding of the data sources available, and how to access and manipulate them. They must be able to identify patterns in data and draw meaningful conclusions from it. As the demand for data-driven decisions increases, the demand for Data Wranglers is expected to grow as well.
[Education Paths]
The recommended educational path for learners who have completed this course is to pursue a degree in Data Science. Data Science is a field of study that combines mathematics, statistics, computer science, and domain knowledge to analyze and interpret data. It involves the use of data wrangling techniques to extract, clean, and transform data into meaningful insights. Data Science also involves the use of machine learning algorithms to identify patterns and trends in data.
A degree in Data Science typically requires a combination of coursework in mathematics, statistics, computer science, and domain knowledge. Students may also be required to complete a project or internship in order to gain practical experience in data wrangling and analysis.
The development trend of Data Science is towards the use of more sophisticated algorithms and techniques to analyze and interpret data. This includes the use of artificial intelligence and deep learning to identify patterns and trends in data. Additionally, the use of cloud computing and big data technologies is becoming increasingly important in the field of Data Science.
Course Syllabus
What is tidy data?
Variables, observations, and values
Common data problems
Using the tidyverse
Course Provider
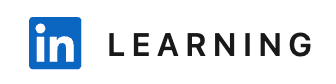
Provider LinkedIn Learning's Stats at AZClass
Discussion and Reviews
0.0 (Based on 0 reviews)
Explore Similar Online Courses
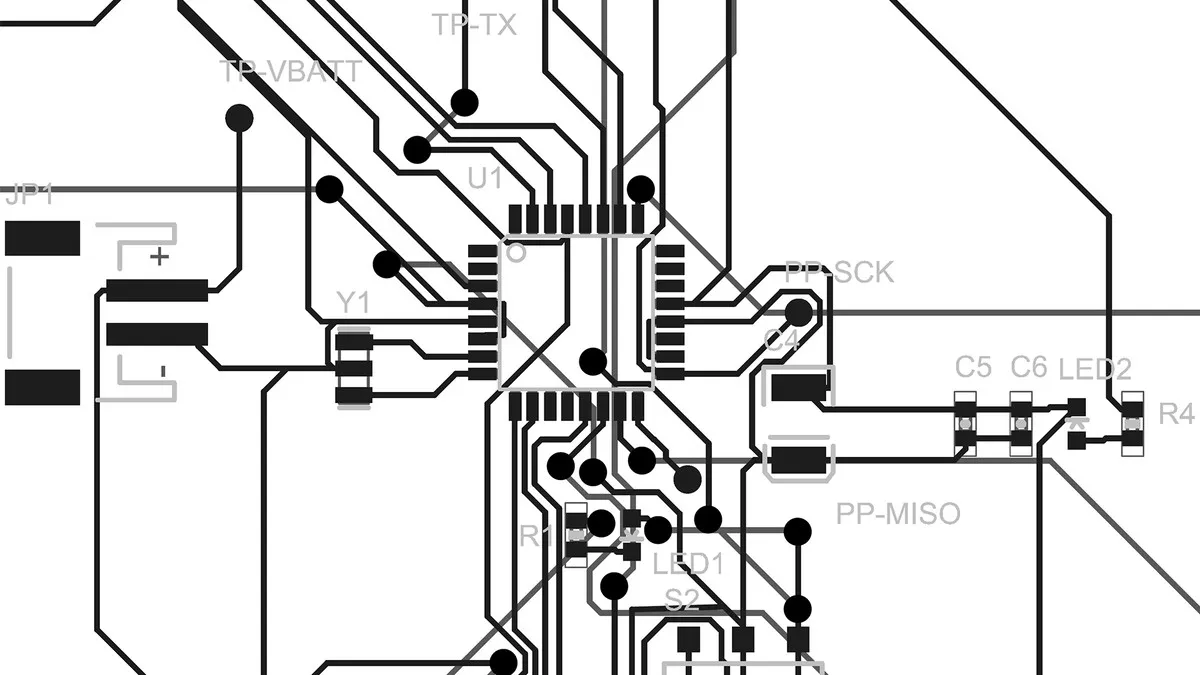
Learning Solidworks PCB
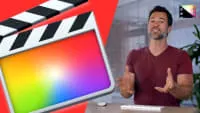
Comprehensive Guide to Final Cut Pro: Part Three
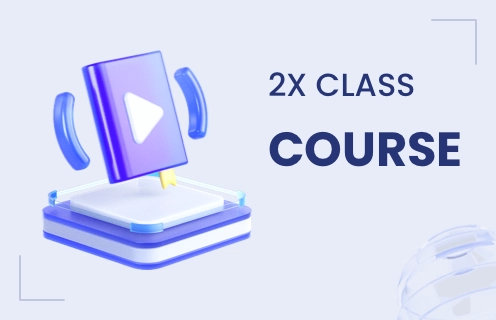
Python for Informatics: Exploring Information
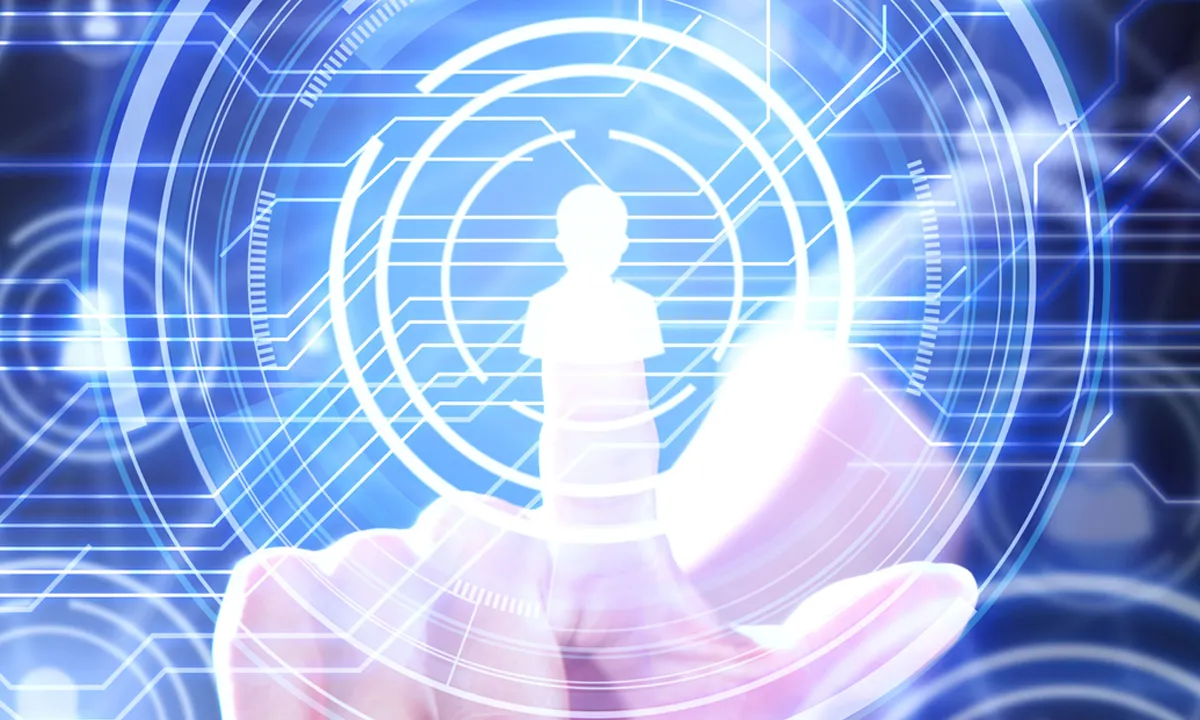
Social Network Analysis

Introduction to Systematic Review and Meta-Analysis
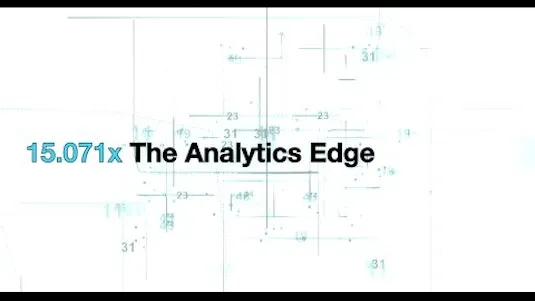
The Analytics Edge

DCO042 - Python For Informatics

Causal Diagrams: Draw Your Assumptions Before Your Conclusions
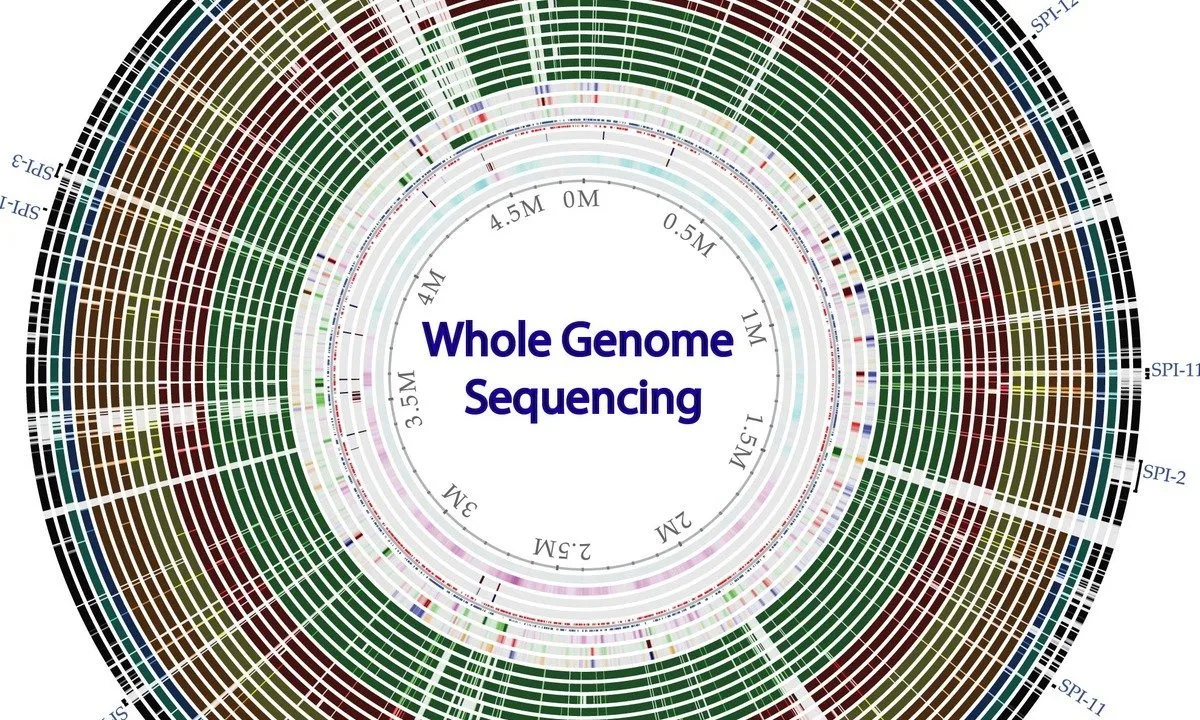
Whole genome sequencing of bacterial genomes - tools and applications
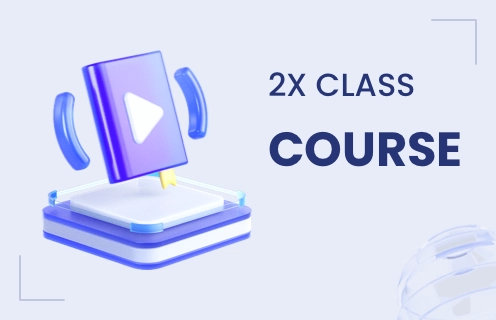
Applied Data Science: An Introduction
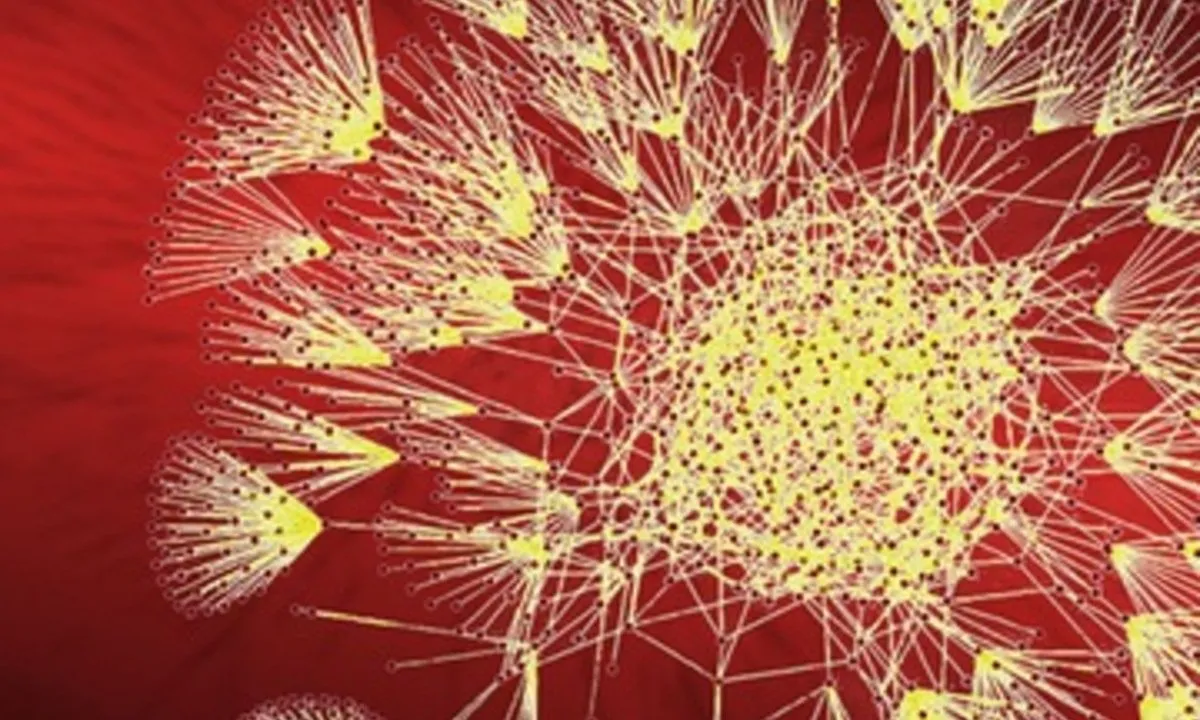
Big Data Integration and Processing
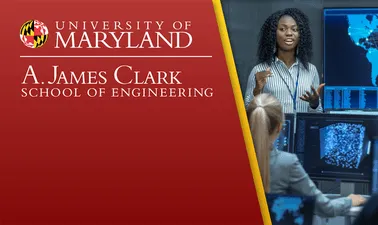
Start your review of Data Wrangling in R : 2017