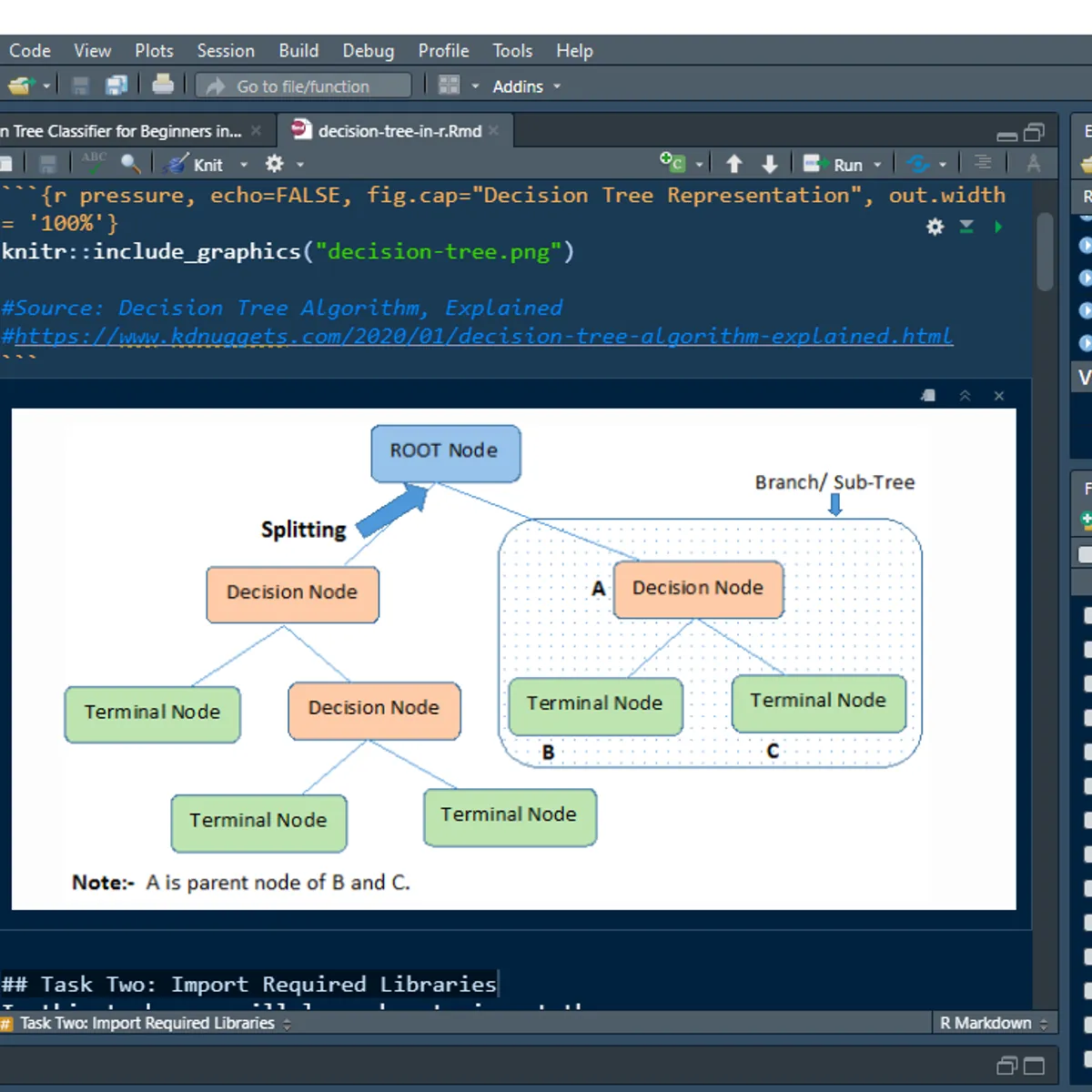
Decision Tree Classifier for Beginners in R




Join this project-based course, "Decision Tree Classifier for Beginners in R," and dive into the world of statistical modeling. In just 2 hours, you'll learn how to build decision tree models using the tree and rpart libraries in R. Explore the Sonar dataset, understand the intuition behind the decision tree algorithm, and evaluate model performance using metrics like Accuracy, Sensitivity, and F1-Score. No prior data analyst or data scientist experience required, but basic knowledge of R is necessary. If you're ready to get your hands dirty, join now and embark on this beautiful journey! ▼
ADVERTISEMENT
Course Feature
Cost:
Paid
Provider:
Coursera
Certificate:
Paid Certification
Language:
English
Start Date:
12th Jun, 2023
Course Overview
❗The content presented here is sourced directly from Coursera platform. For comprehensive course details, including enrollment information, simply click on the 'Go to class' link on our website.
Updated in [September 19th, 2023]
What does this course tell?
(Please note that the following overview content is from the original platform)
Welcome to this project-based course Decision Tree Classifier for Beginners in R. This is a hands-on project that introduces beginners to the world of statistical modeling. In this project, you will learn how to build decision tree models using the tree and rpart libraries in R. We will start this hands-on project by importing the Sonar data into R and exploring the dataset.By the end of this 2-hour long project, you will understand the basic intuition behind the decision tree algorithm and how it works. To build the model, we will divide or partition the data into the training and testing data set. Finally, you will learn how to evaluate the model’s performance using metrics like Accuracy, Sensitivity, Specificity, F1-Score, and so on. By extension, you will learn how to save the trained model on your local system. Although you do not need to be a data analyst expert or data scientist to succeed in this guided project, it requires a basic knowledge of using R, especially writing R syntaxes. Therefore, to complete this project, you must have prior experience with using R. If you are not familiar with working with using R, please go ahead to complete my previous project titled: “Getting Started with R”. It will hand you the needed knowledge to go ahead with this project on Decision Tree. However, if you are comfortable with working with R, please join me on this beautiful ride! Let’s get our hands dirty!We consider the value of this course from multiple aspects, and finally summarize it for you from three aspects: personal skills, career development, and further study:
(Kindly be aware that our content is optimized by AI tools while also undergoing moderation carefully from our editorial staff.)
What skills and knowledge will you acquire during this course?
During this course, the learner will acquire the skills and knowledge necessary to build decision tree models using the tree and rpart libraries in R. They will learn how to import data into R and explore the dataset. By the end of the project, they will have a basic understanding of the decision tree algorithm and how it works. They will also learn how to partition the data into training and testing sets, evaluate the model's performance using metrics like Accuracy, Sensitivity, Specificity, and F1-Score, and save the trained model on their local system. Prior experience with using R is required to successfully complete this project.
How does this course contribute to professional growth?
This course on Decision Tree Classifier for Beginners in R contributes to professional growth by providing a hands-on project that introduces beginners to the world of statistical modeling. By completing this course, individuals will gain practical experience in building decision tree models using the tree and rpart libraries in R.
Through this project, individuals will learn how to import data into R, explore datasets, and understand the basic intuition behind the decision tree algorithm. They will also learn how to divide data into training and testing datasets, build models, and evaluate their performance using metrics like Accuracy, Sensitivity, Specificity, and F1-Score.
By gaining proficiency in these skills, individuals will enhance their ability to analyze and interpret data, which is a valuable asset in various professional fields such as data analysis, data science, and research. Additionally, individuals will learn how to save trained models on their local systems, allowing them to apply their knowledge to real-world scenarios.
While prior experience with R is required to complete this project, individuals who already have a basic understanding of R will find this course to be a valuable resource for expanding their skill set and advancing their professional growth. By actively participating in this project, individuals will gain practical experience and confidence in using R for statistical modeling, which can open up new opportunities for career advancement and professional success.
Is this course suitable for preparing further education?
This course appears to be suitable for preparing further education. It is a project-based course that introduces beginners to statistical modeling and specifically focuses on building decision tree models using R. The course covers importing and exploring data, understanding the decision tree algorithm, partitioning data into training and testing sets, evaluating model performance, and saving trained models. While prior experience with R is required, the course does not require expertise in data analysis or being a data scientist. Therefore, individuals with basic knowledge of R can benefit from this course to enhance their skills and knowledge in preparation for further education.
Course Provider

Provider Coursera's Stats at AZClass
Discussion and Reviews
0.0 (Based on 0 reviews)
Explore Similar Online Courses
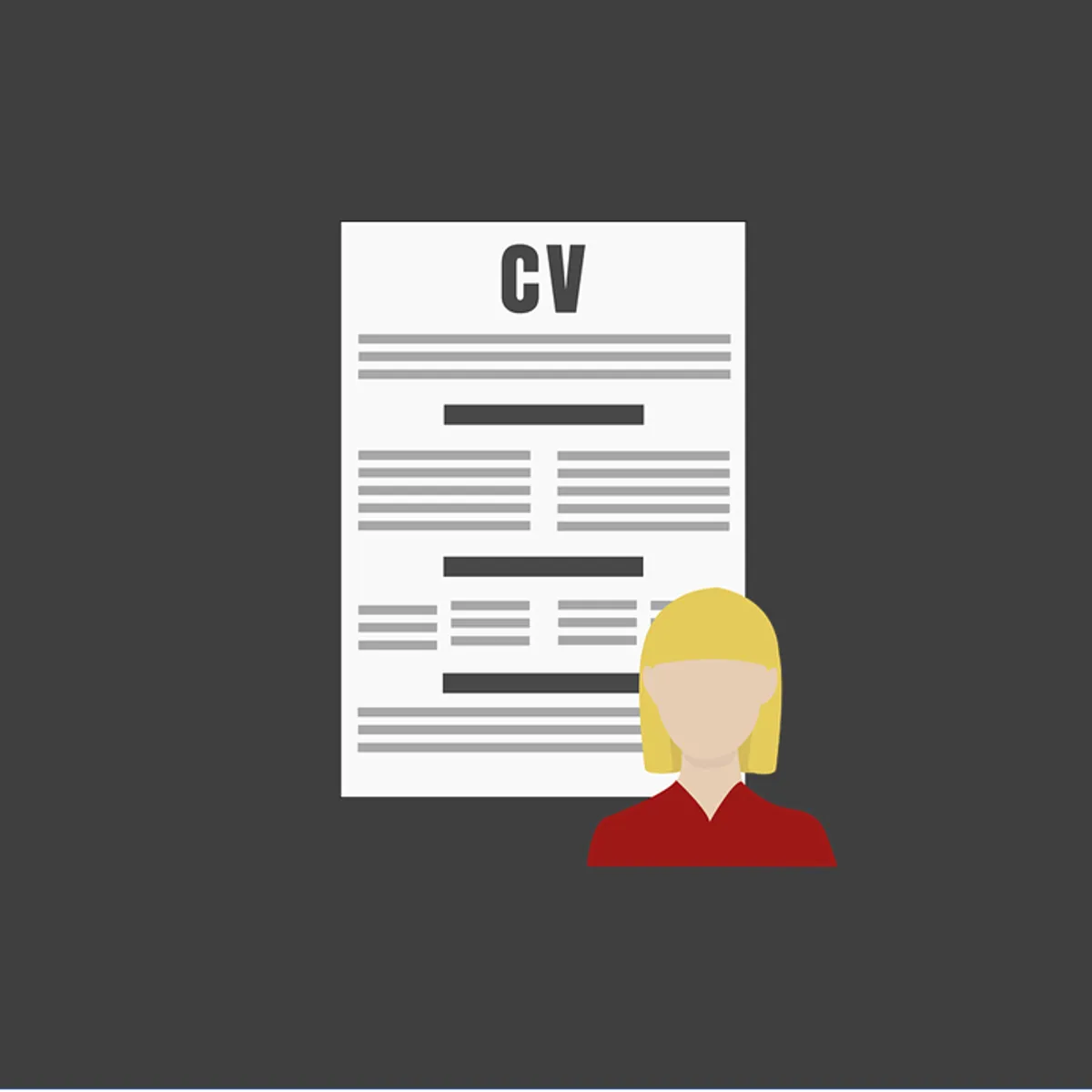
Naive Bayes 101: Resume Selection with Machine Learning
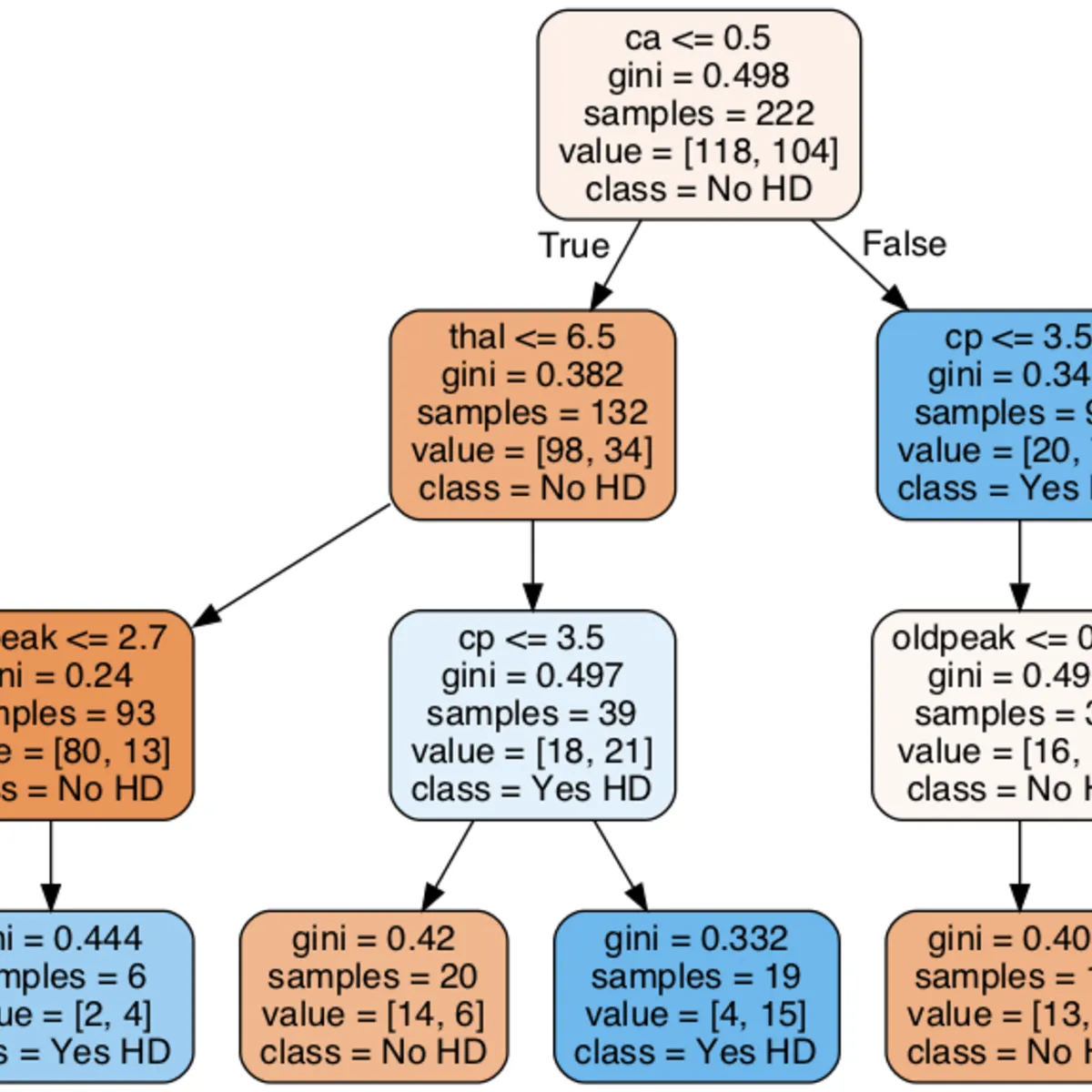
Classification Trees in Python From Start To Finish
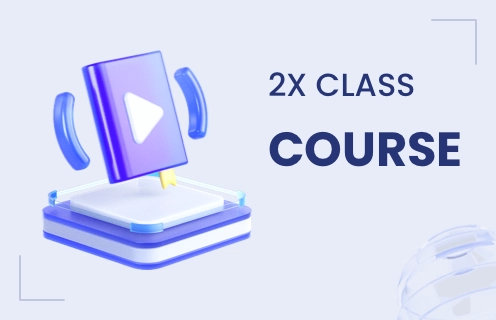
Python for Informatics: Exploring Information
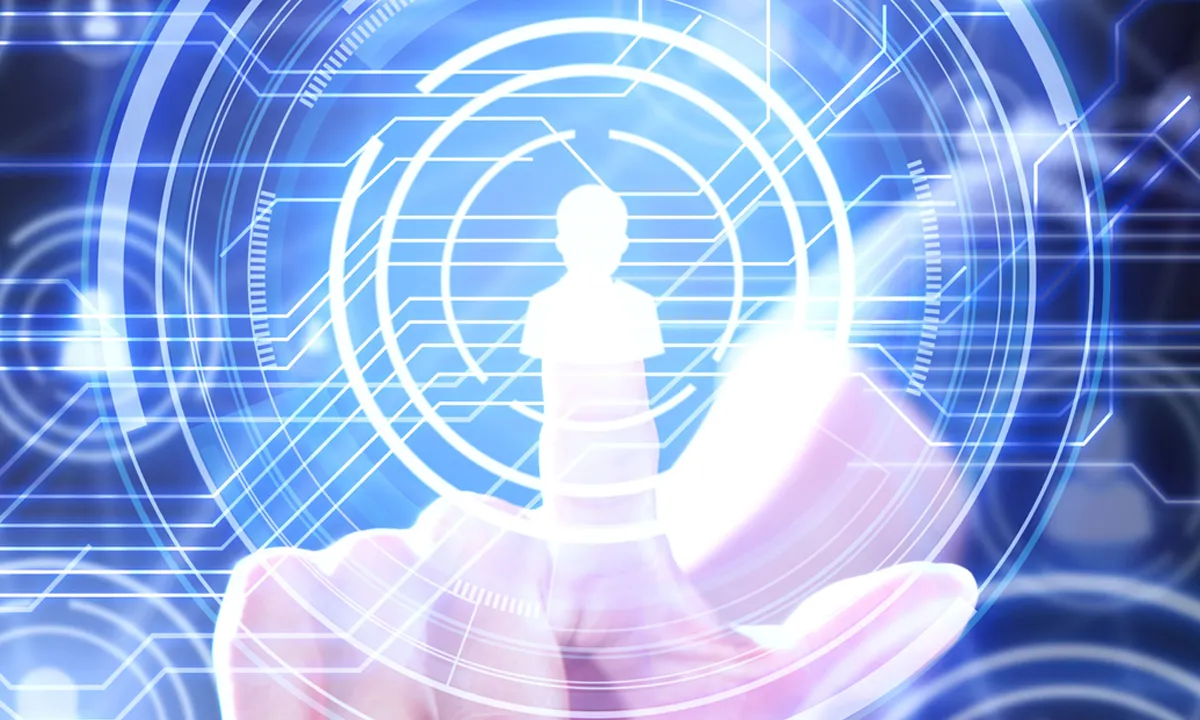
Social Network Analysis

Introduction to Systematic Review and Meta-Analysis
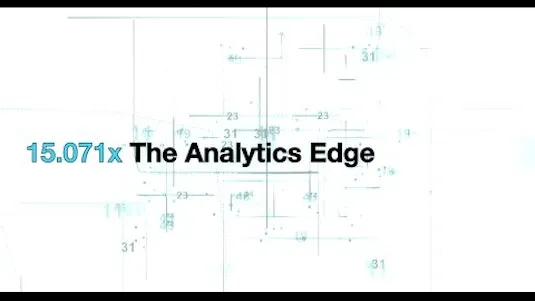
The Analytics Edge

DCO042 - Python For Informatics

Causal Diagrams: Draw Your Assumptions Before Your Conclusions
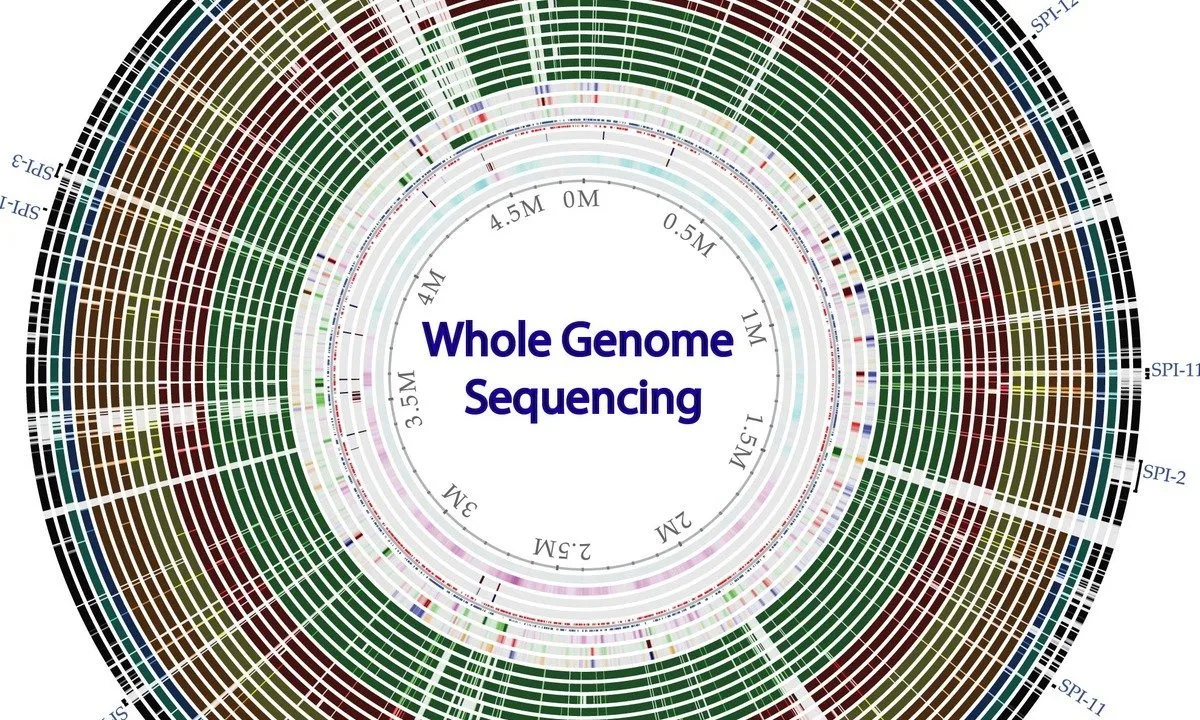
Whole genome sequencing of bacterial genomes - tools and applications
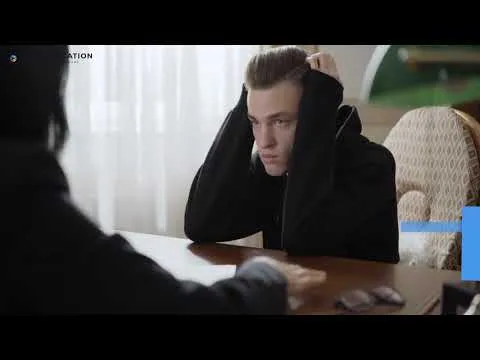
Criminal Intelligence Analyst
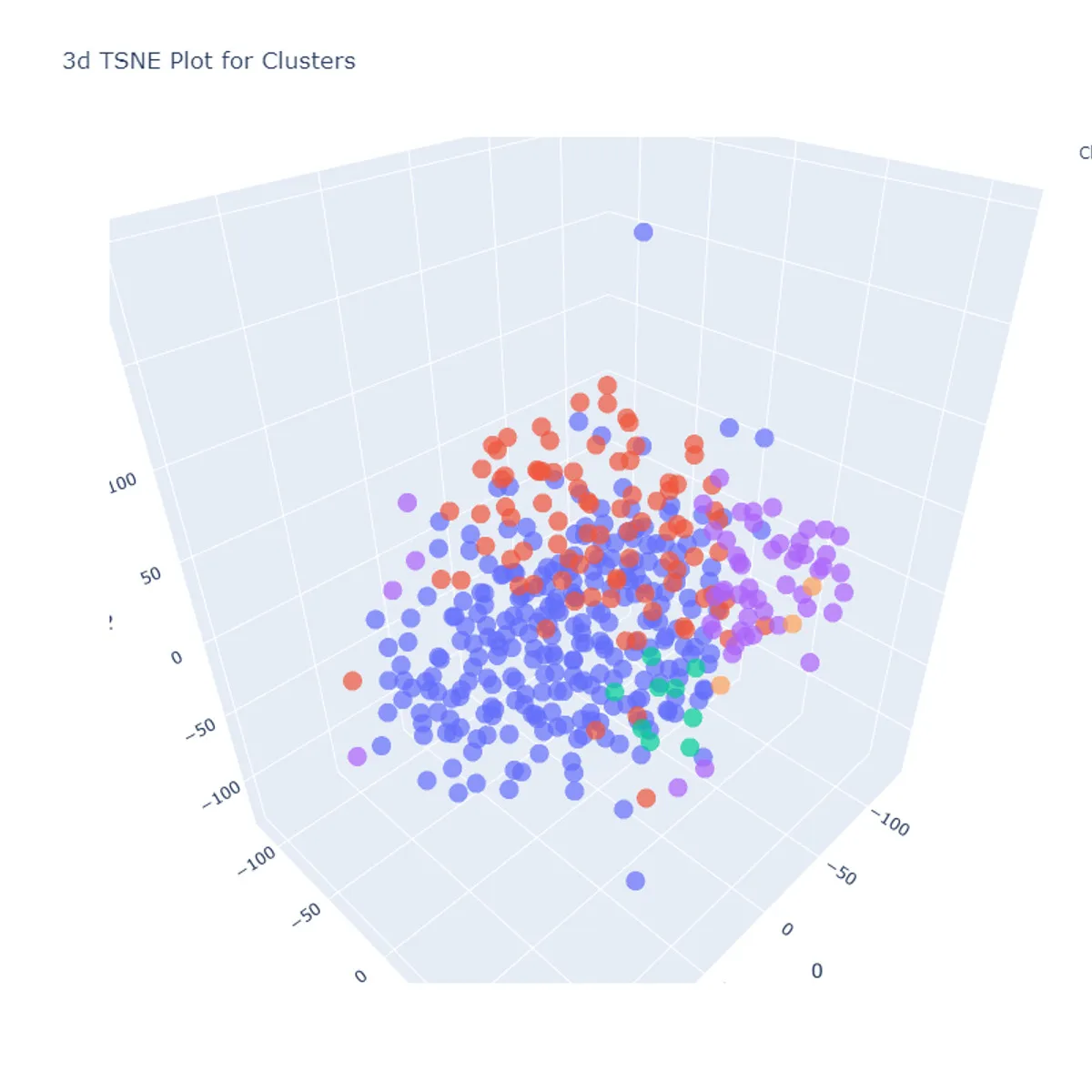
Build a Clustering Model using PyCaret
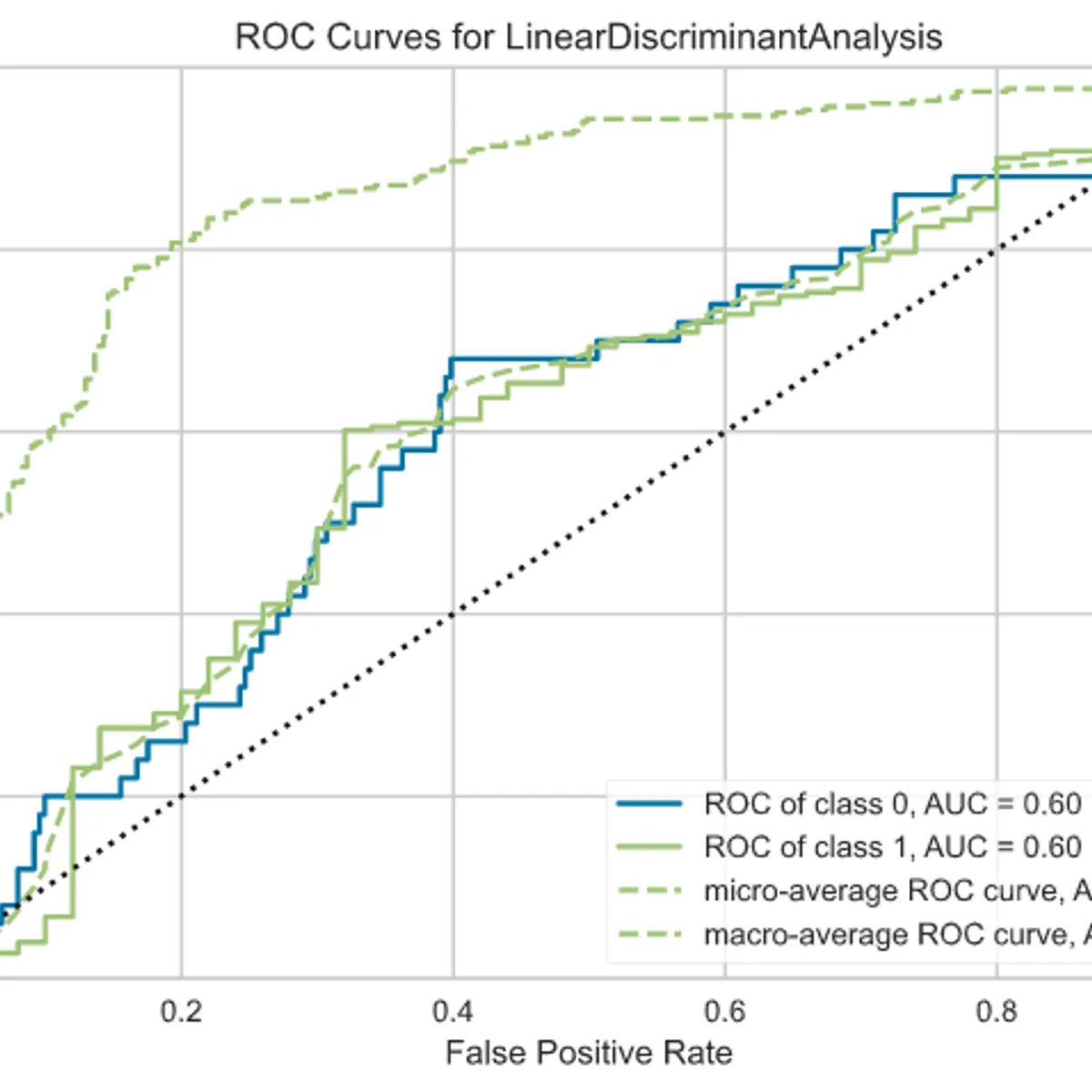
Start your review of Decision Tree Classifier for Beginners in R