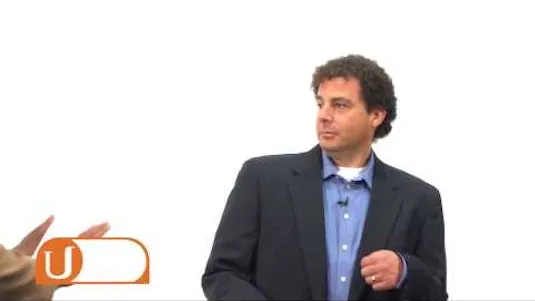
Machine Learning: Unsupervised Learning





Enroll in this Machine Learning Series and learn how to use Unsupervised Learning to identify structure in data. With Professor Isbell and Professor Littman as your guides, you'll gain a deep understanding of the powerful techniques used to uncover patterns in data. ▼
ADVERTISEMENT
Course Feature
Cost:
Free
Provider:
Udacity
Certificate:
No Information
Language:
English
Start Date:
On-Demand
Course Overview
❗The content presented here is sourced directly from Udacity platform. For comprehensive course details, including enrollment information, simply click on the 'Go to class' link on our website.
Updated in [June 30th, 2023]
What skills and knowledge will you acquire during this course?
The skills and knowledge that will be acquired during this course include understanding the concept of Unsupervised Learning and its application in analyzing data and identifying patterns. Students will learn about randomized optimization, clustering, and feature selection and transformation techniques used in Unsupervised Learning. The course will provide insights into how Unsupervised Learning approaches can be used to find structure in unlabeled data.
How does this course contribute to professional growth?
The course contributes to professional growth by providing knowledge and skills in analyzing data and identifying patterns. It focuses on various Unsupervised Learning approaches such as randomized optimization, clustering, and feature selection and transformation. This course is part of a graduate-level series on Machine Learning, which aims to enhance understanding and proficiency in Artificial Intelligence.
Is this course suitable for preparing further education?
The course "Machine Learning: Unsupervised Learning" focuses on using Unsupervised Learning approaches to find structure in unlabeled data. It covers topics such as randomized optimization, clustering, and feature selection and transformation. This course is part of a graduate-level series of 3 courses on Machine Learning, which is taught by Professor Charles Isbell from Georgia Tech and Professor Michael Littman from Brown University.
Course Syllabus
Randomized optimization
Optimization, randomized,Hill climbing,Random restart hill climbing,Simulated annealing,Annealing algorithm,Properties of simulated annealing,Genetic algorithms,GA skeleton,Crossover example,What have we learned,MIMIC,MIMIC: A probability model,MIMIC: Pseudo code,MIMIC: Estimating distributions,Finding dependency trees,Probability distributionClustering
Clustering and expectation maximization,Basic clustering problem,Single linkage clustering (SLC),Running time of SLC,Issues with SLC,K-means clustering,K-means in Euclidean space,K-means as optimization,Soft clustering,Maximum likelihood Gaussian,Expectation Maximization (EM),Impossibility theoremFeature Selection
Algorithms,Filtering and Wrapping,Speed,Searching,Relevance,Relevance vs. UsefulnessFeature Transformation
Feature Transformation,Words like Tesla,Principal Components Analysis,Independent Components Analysis,Cocktail Party Problem,Matrix,AlternativesInformation Theory
History -Sending a Message,Expected size of the message,Information between two variables,Mutual information,Two Independent Coins,Two Dependent Coins,Kullback Leibler DivergenceCourse Provider
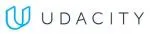
Provider Udacity's Stats at AZClass
Discussion and Reviews
0.0 (Based on 0 reviews)
Explore Similar Online Courses
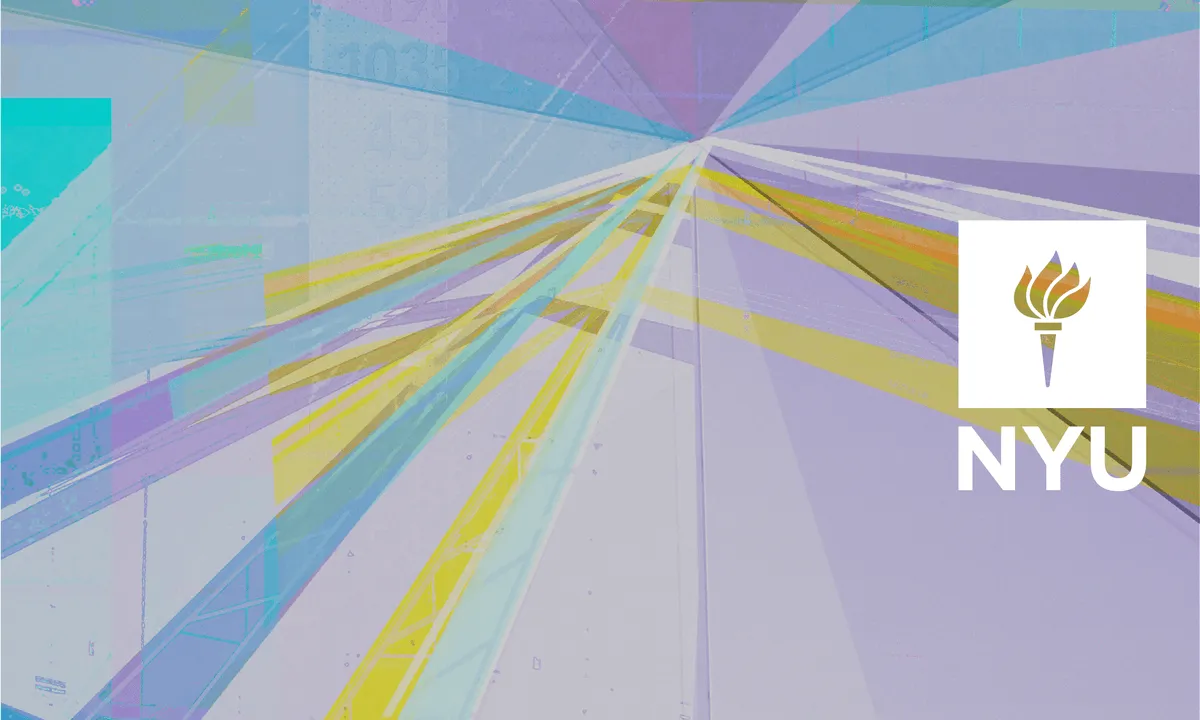
Information Visualization: Programming with D3js
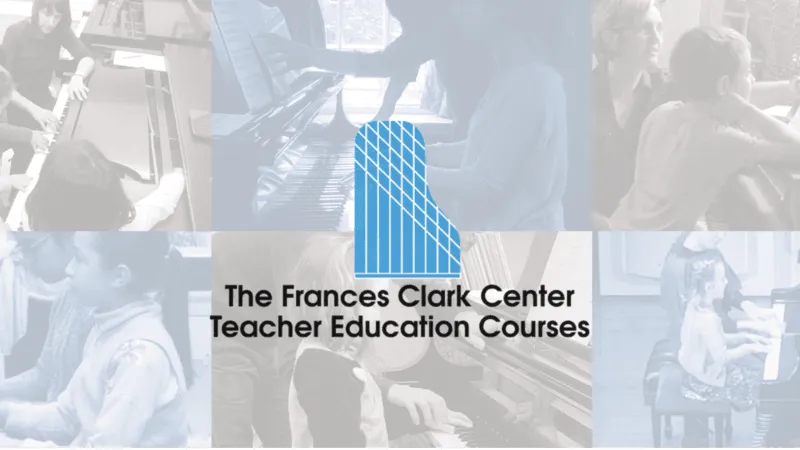
Developing Musical Skills in Young Pianists: Ideas from Music Learning Theory (from The Beginner Course)
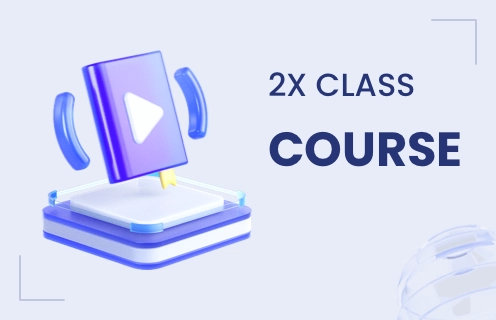
Python for Informatics: Exploring Information
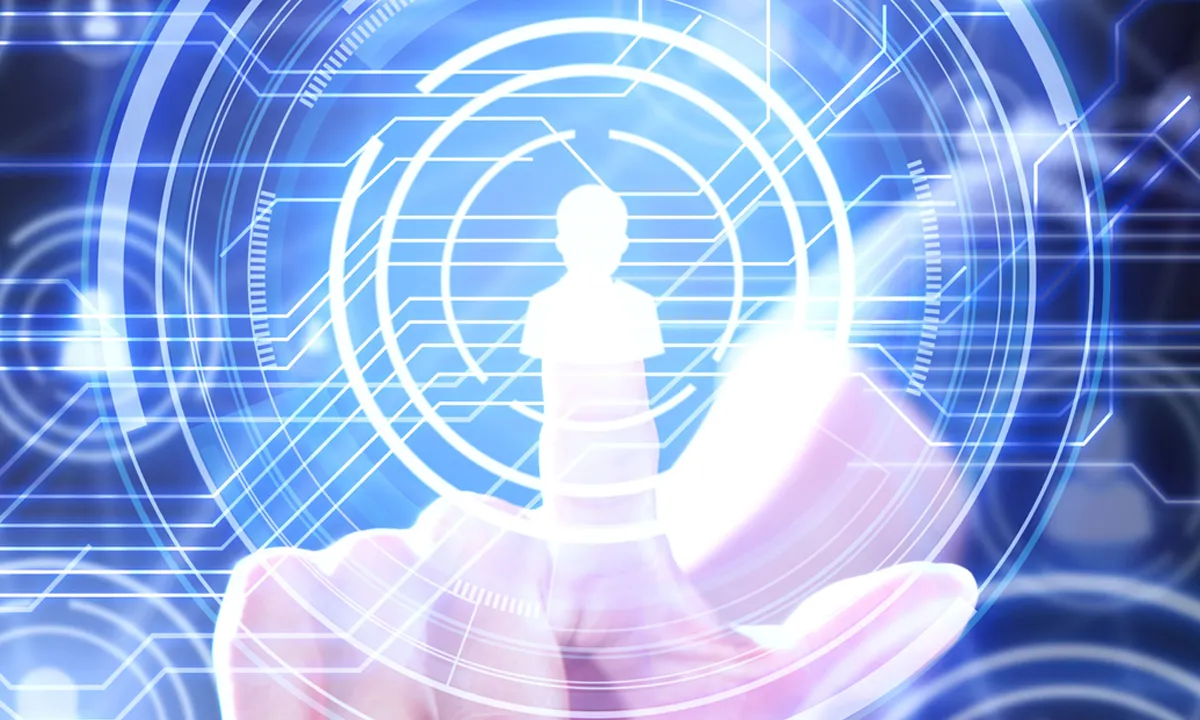
Social Network Analysis

Introduction to Systematic Review and Meta-Analysis
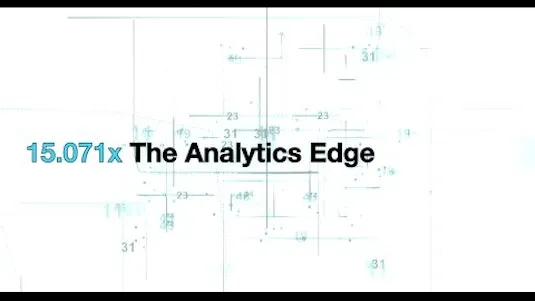
The Analytics Edge

DCO042 - Python For Informatics

Causal Diagrams: Draw Your Assumptions Before Your Conclusions
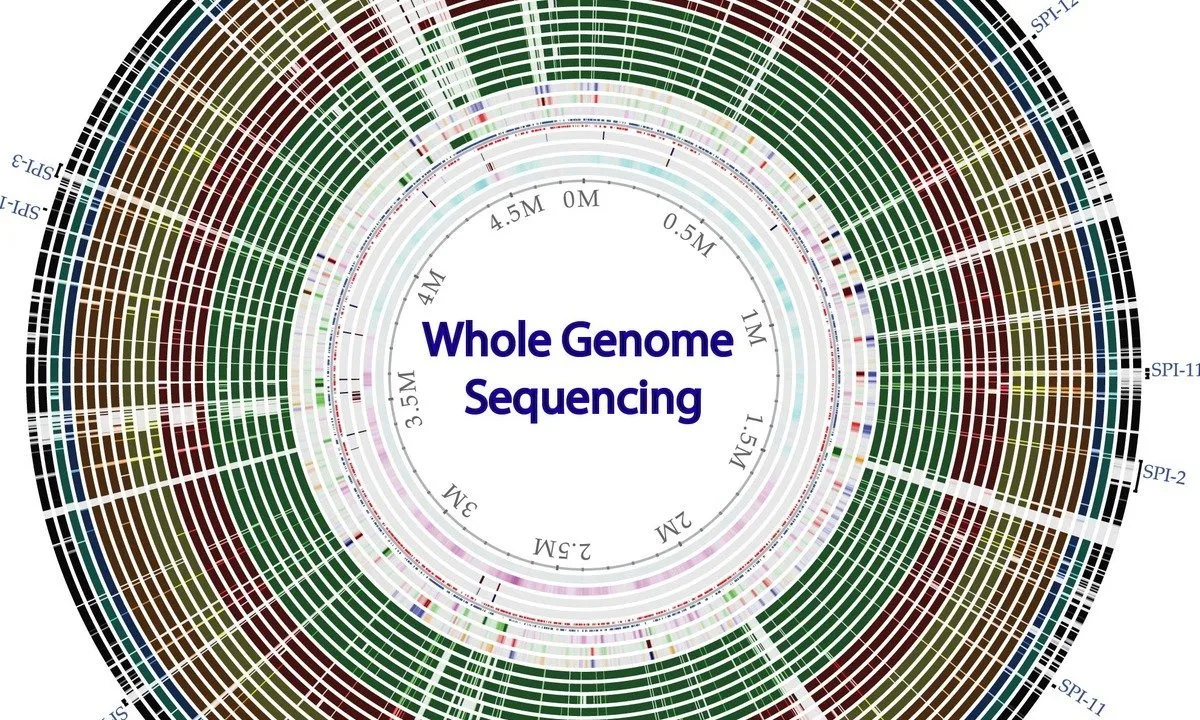
Whole genome sequencing of bacterial genomes - tools and applications
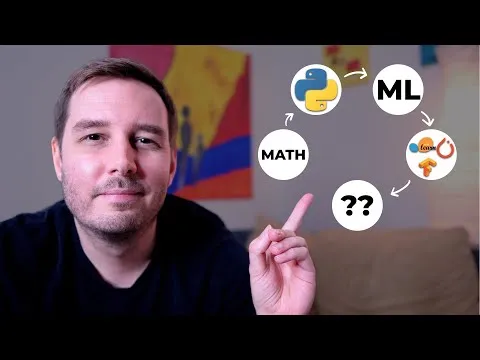
How I would learn Machine Learning (if I could start over)
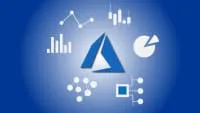
Learn Data Science and Machine Learning on Microsoft Azure
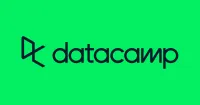
Start your review of Machine Learning: Unsupervised Learning