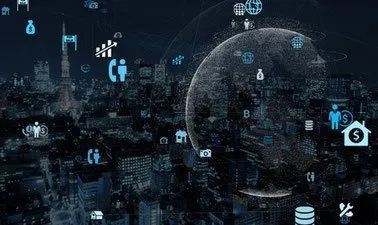
Text Analytics 1: Introduction to Natural Language Processing





Discover the power of Natural Language Processing (NLP) and Computational Linguistics with Text Analytics 1: Introduction to Natural Language Processing. Learn how to create automated pipelines for text classification and text similarity using Python packages like pandas, scikit-learn, and tensorflow. Understand the limits of a computational approach to language and the ethical guidelines for applying it to real-world problems. Explore topics like text processing, text mining, sentiment analysis, and topic modeling. ▼
ADVERTISEMENT
Course Feature
Cost:
Free
Provider:
Edx
Certificate:
Paid Certification
Language:
English
Start Date:
Self paced
Course Overview
❗The content presented here is sourced directly from Edx platform. For comprehensive course details, including enrollment information, simply click on the 'Go to class' link on our website.
Updated in [June 30th, 2023]
Text Analytics 1: Introduction to Natural Language Processing is a course that introduces the core techniques of natural language processing (NLP) and computational linguistics. It is part one of the Text Analytics with Python professional certificate, but can also be studied as a stand-alone course. The course provides a practical and scientific introduction to natural language processing, teaching students how to create pipelines for text classification and text similarity that use machine learning. Students will learn to use Python packages such as pandas, scikit-learn, and tensorflow. The course will also cover topics such as text processing, text mining, sentiment analysis, and topic modeling.
[Applications]
Upon completion of Text Analytics 1: Introduction to Natural Language Processing, students will be able to apply the core techniques of natural language processing and computational linguistics to create automated workflows for text classification and text similarity. They will also be able to use Python packages such as pandas, scikit-learn, and tensorflow to analyze text data. Additionally, students will have a better understanding of the limits of a computational approach to language and the ethical guidelines for applying it to real-world problems.
[Career Path]
A recommended career path for learners of this course is a Text Analytics Engineer. Text Analytics Engineers are responsible for developing and deploying text analytics solutions to extract insights from large amounts of unstructured text data. They use natural language processing (NLP) techniques to process and analyze text data, and then use machine learning algorithms to identify patterns and trends in the data. They also develop and maintain text analytics pipelines, and create visualizations to present the results of their analyses.
The development trend for Text Analytics Engineers is to become more specialized in their field. As the amount of text data continues to grow, the need for engineers who can develop more sophisticated solutions to extract insights from this data is increasing. Text Analytics Engineers are expected to become more knowledgeable in the latest NLP techniques and machine learning algorithms, and to be able to develop more complex pipelines and visualizations. Additionally, they must be able to work with a variety of data sources, including social media, webpages, and customer feedback.
[Education Path]
The recommended educational path for learners interested in Text Analytics 1: Introduction to Natural Language Processing is to pursue a degree in Computational Linguistics. This degree combines the study of linguistics, computer science, and artificial intelligence to develop an understanding of how language works and how to use computers to process and analyze language. Students will learn about the fundamentals of linguistics, such as syntax, semantics, and pragmatics, as well as the fundamentals of computer science, such as algorithms, data structures, and programming languages. They will also learn about the application of artificial intelligence to natural language processing, such as machine learning, deep learning, and natural language generation.
The development trend of Computational Linguistics is to focus on the development of more sophisticated algorithms and techniques for natural language processing. This includes the development of more powerful machine learning models, the use of deep learning to better understand language, and the development of natural language generation systems. Additionally, there is a focus on the ethical implications of using natural language processing, such as the potential for bias in algorithms and the need for responsible data collection and analysis.
Course Provider
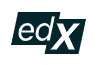
Provider Edx's Stats at AZClass
Discussion and Reviews
0.0 (Based on 0 reviews)
Explore Similar Online Courses

Python Sequences Course
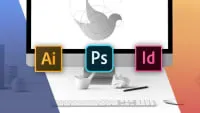
Graphic Design Masterclass Intermediate: The NEXT Level
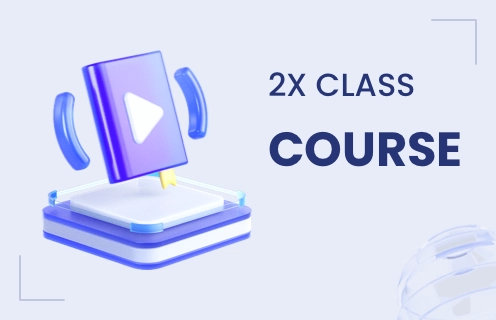
Python for Informatics: Exploring Information
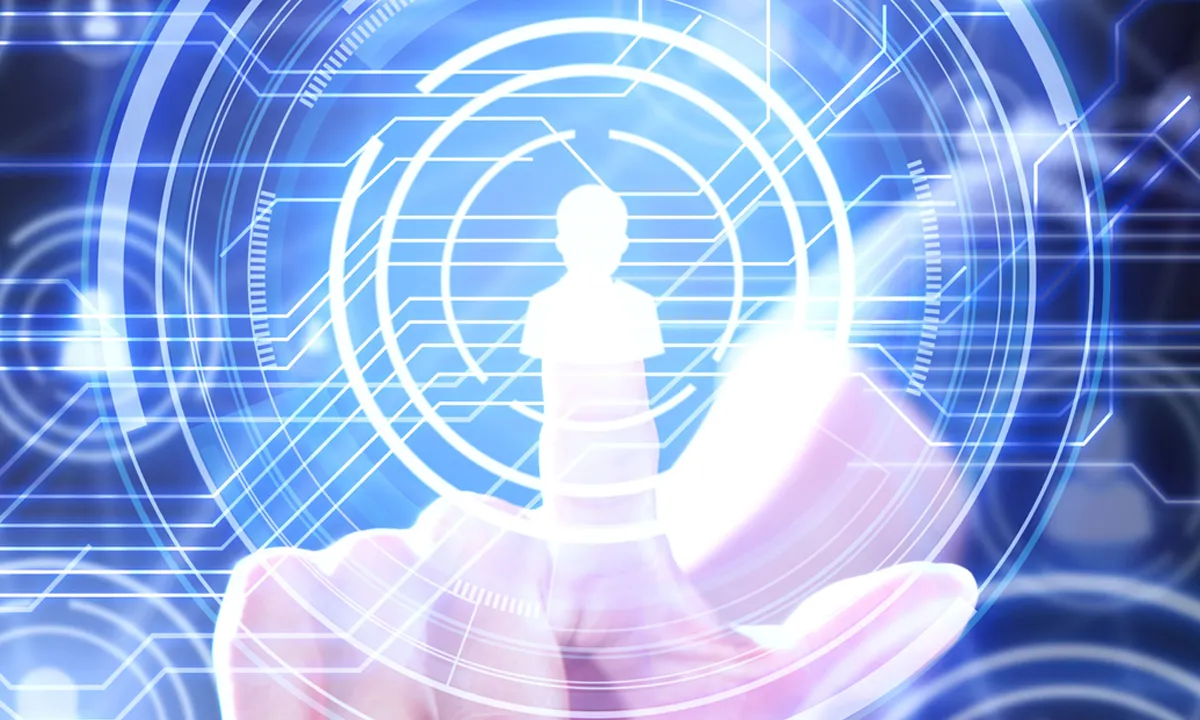
Social Network Analysis

Introduction to Systematic Review and Meta-Analysis
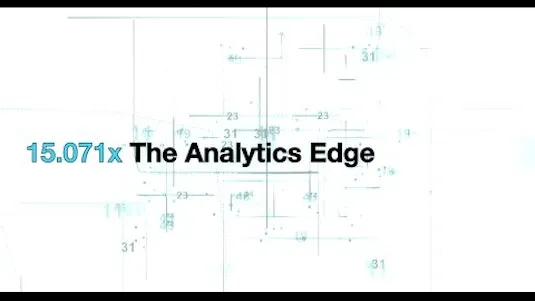
The Analytics Edge

DCO042 - Python For Informatics

Causal Diagrams: Draw Your Assumptions Before Your Conclusions
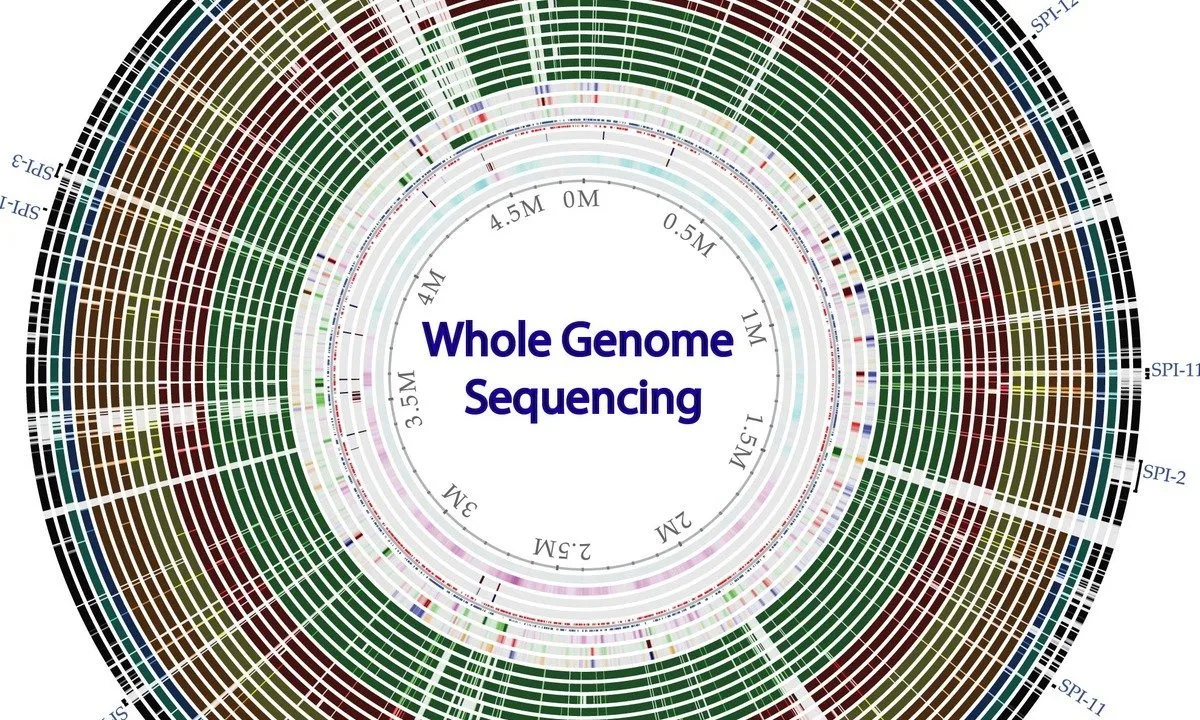
Whole genome sequencing of bacterial genomes - tools and applications
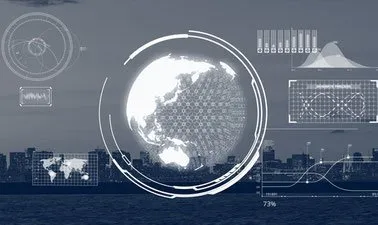
Text Analytics 2: Visualizing Natural Language Processing
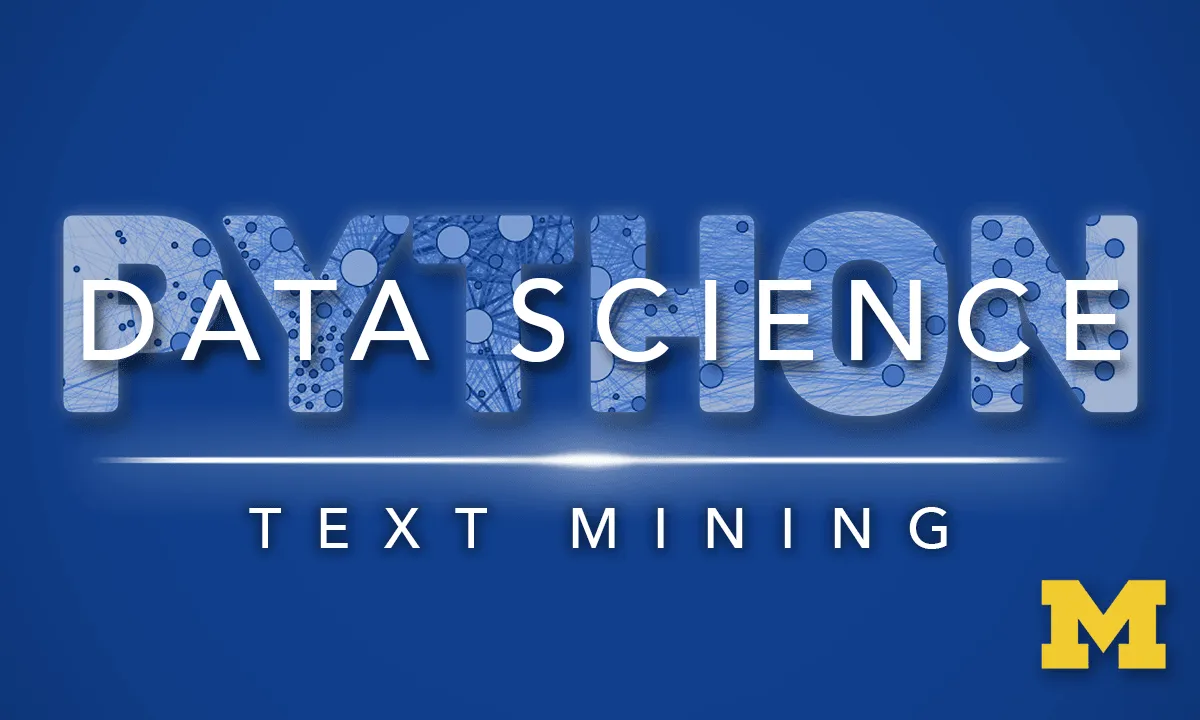
Applied Text Mining in Python
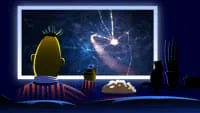
Start your review of Text Analytics 1: Introduction to Natural Language Processing