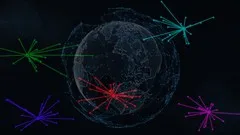
Unsupervised Machine Learning: Cluster Analysis Algorithms





Discover the power of unsupervised machine learning with the "Unsupervised Machine Learning: Cluster Analysis Algorithms" course. Clustering is a vital tool for data scientists, allowing them to uncover patterns in unlabelled data. In this course, you will delve into the core concepts of cluster analysis and learn five essential clustering algorithms. From centroid-based algorithms like KMeans and Meanshift to density-based algorithms like DBSCAN and OPTICS, you will gain a deep understanding of each algorithm's working, parameter tuning, and evaluation. Code along with detailed jupyter notebooks and apply your learnings to multiple datasets. With lifetime access, this course will be your go-to reference for mastering clustering algorithms. Get a comprehesive understanding of Unsupervised Machine Learning: Cluster Analysis Algorithms which is a pay course. AZ Class provides this course data for free. Learn more certificate and details here. ▼
ADVERTISEMENT
Course Feature
Cost:
Paid
Provider:
Udemy
Certificate:
Paid Certification
Language:
English
Start Date:
2020-08-09
Course Overview
❗The content presented here is sourced directly from Udemy platform. For comprehensive course details, including enrollment information, simply click on the 'Go to class' link on our website.
Updated in [October 16th, 2023]
What does this course tell?
(Please note that the following overview content is from the original platform)
Clustering is the activity of splitting the data into partitions that give an insight about the unlabelled data. It gives a structure to the data by grouping similar data pointsWe see these clustering algorithms almost everywhere in our everyday life. Cluster Analysis has and always will be a staple for all Machine Learning. Clustering has its applications in many Machine Learning tasks: label generation, label validation, dimensionality reduction, semi supervised learning, Reinforcement learning, computer vision, natural language processing.For a data scientist, cluster analysis is one of the first tools in their arsenal during exploratory analysis, that they use to identify natural partitions in the data.In this course, you will learn some of the most important algorithms used for Cluster AnalysisEach dataset and feature space is unique. You cannot use a one-size-fits-all method for recognizing patterns in the data. Each algorithm has its own purpose.By studying the core concepts and working in detail and writing the code for each algorithm from scratch, will empower you, to identify the correct algorithm to use for each scenario.Some algorithms are fast and are a good starting point to quickly identify the pattern of the dataAnd some algorithms are slow but more precise, and allow you to capture the pattern very accurately.You will get to understand each algorithm in detail, which will give you the intuition for tuning their parameters and maximizing their utilityIn this course, for cluster analysis you will learn five clustering algorithms:You will learn about KMeans and Meanshift. These are two centroid based algorithms, which means their definition of a cluster is based around the center of the cluster.Next you will study DBSCAN and OPTICS. These are density based algorithms, in which they find high density zones in the data and for such continuous density zones, they identify them as clusters.Another type of algorithm that you will learn is Agglomerative Clustering, a hierarchical style of clustering algorithm, which gives us a hierarchy of clusters.For each algorithm, you will understand the core working of the algorithm. What parameters they use. How to choose and tune these parameters. How to evaluate the results for each algorithm. To consolidate your understanding, you will also apply all these learnings on multiple datasets for each algorithm. You can later compare all the algorithms and their performance.This course can be your only reference that you need, for learning about various clustering algorithms.The correct approach to this course is going in the given order the first time.Follow along the introductory lecture. It is highly recommended that during the coding lessons, you must code along. I have provided detailed jupyter notebooks along the course. You can keep them for reference. But it is highly recommended that you code along.You can pause the lesson. Write the code needed and at the same time think about the working flow.I assure you, there onwards, this course can be your go-to reference to answer all questions about these algorithms. You will have a lifetime of access to this course, and thus you can keep coming back to quickly brush up on these algorithms
We considered the value of this course from many aspects, and finally summarized it for you from two aspects: skills and knowledge, and the people who benefit from it:
(Please note that our content is optimized through artificial intelligence tools and carefully reviewed by our editorial staff.)
What skills and knowledge will you acquire during this course?
Understanding Cluster Analysis: Gain a comprehensive understanding of cluster analysis, a fundamental technique in machine learning used for partitioning unlabelled data into meaningful clusters.
Practical Application: Learn how to practically apply cluster analysis in a variety of fields, including label generation, label validation, dimensionality reduction, semi-supervised learning, reinforcement learning, computer vision, and natural language processing.
Exploratory Data Analysis: Develop the ability to utilize cluster analysis for exploratory data analysis, allowing data scientists to identify natural partitions within datasets.
Algorithmic Expertise: Explore five essential clustering algorithms, including K-Means, Mean Shift, DBSCAN, OPTICS, and Agglomerative Clustering. Understand the unique characteristics, working principles, and parameter tuning for each algorithm.
Hierarchical Clustering: Gain proficiency in hierarchical clustering, a method that creates a hierarchy of clusters, providing insights into the data's structure.
Parameter Tuning: Acquire the skills to intuitively tune parameters for each clustering algorithm to optimize their performance based on the specific dataset and objectives.
Results Evaluation: Learn techniques to evaluate and compare the results obtained from different clustering algorithms, allowing for informed decision-making.
Hands-On Practice: Code along with practical examples using Jupyter notebooks provided in the course, enhancing your ability to implement these algorithms in real-world scenarios.
Lifelong Reference: With lifetime access to the course, it serves as a comprehensive reference for learners, enabling them to revisit and refresh their understanding of clustering algorithms whenever needed.
Who will benefit from this course?
This course on Unsupervised Machine Learning: Cluster Analysis Algorithms will benefit data scientists and machine learning practitioners who are interested in exploring and analyzing unlabelled data. It is particularly useful for those who want to gain insights from data by identifying natural partitions and patterns.
Specific professions that can benefit from this course include:
1. Data Scientists: Cluster analysis is an essential tool for data scientists during exploratory analysis. This course will provide them with the necessary knowledge and skills to effectively use clustering algorithms in their work.
2. Machine Learning Practitioners: Professionals working in the field of machine learning will find this course valuable as it covers important clustering algorithms used in various machine learning tasks such as label generation, label validation, dimensionality reduction, semi-supervised learning, reinforcement learning, computer vision, and natural language processing.
3. Researchers: Researchers in fields such as computer vision, natural language processing, and data analysis can benefit from this course as it provides a comprehensive understanding of different clustering algorithms and their applications.
4. Programmers and Developers: This course is suitable for programmers and developers who want to expand their knowledge in machine learning and data analysis. It covers the core concepts of clustering algorithms and provides hands-on coding exercises to enhance their understanding.
5. Students and Enthusiasts: Students studying data science, machine learning, or related fields, as well as individuals with a general interest in unsupervised machine learning, can benefit from this course. It provides a solid foundation in cluster analysis algorithms and offers practical examples to reinforce learning.
Course Syllabus
Clustering : Getting Started
K-Means Clustering
DBSCAN
OPTICS
Meanshift Clustering
Hierarchical Agglomerative Clustering
Course Provider
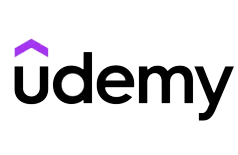
Provider Udemy's Stats at AZClass
Discussion and Reviews
0.0 (Based on 0 reviews)
Explore Similar Online Courses
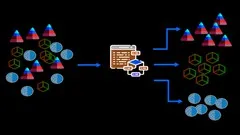
Cluster Analysis : Unsupervised Machine Learning in Python
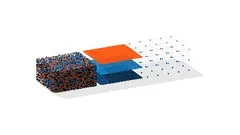
Introduction to Clustering using R
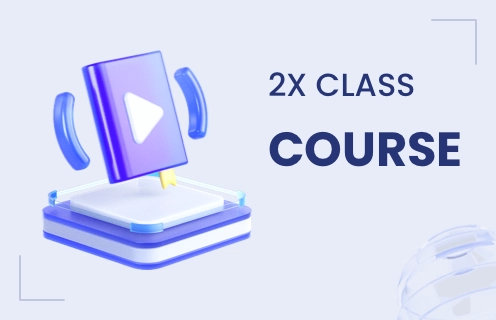
Python for Informatics: Exploring Information
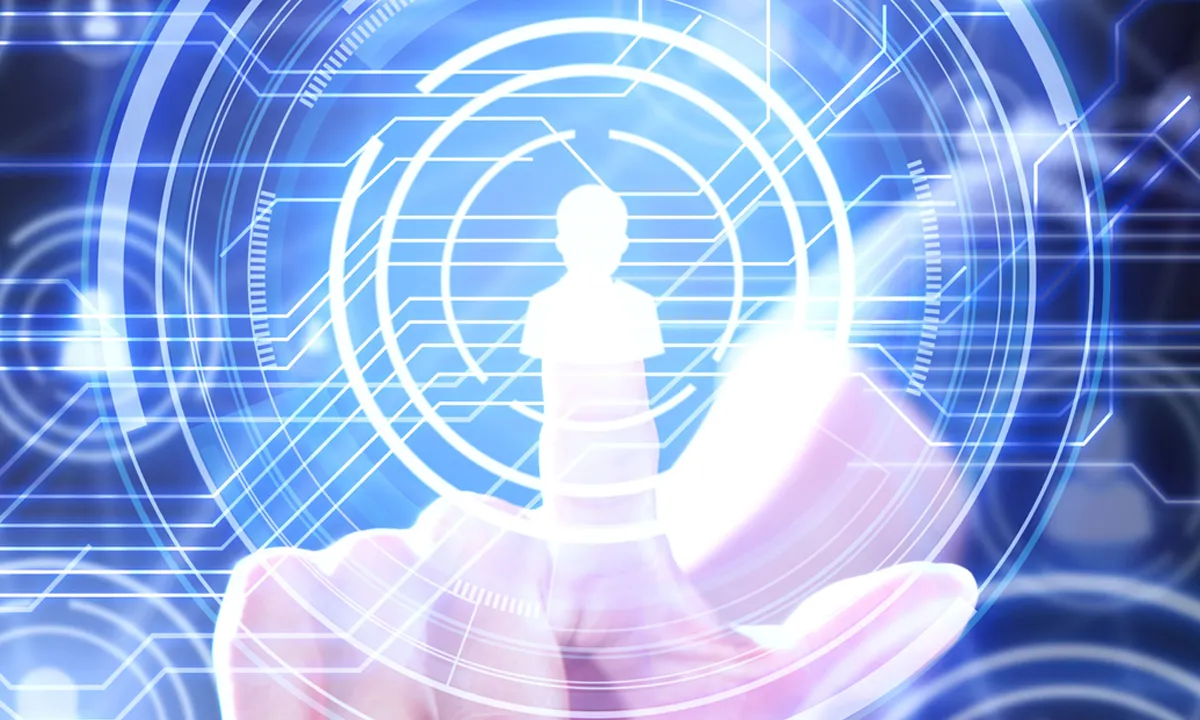
Social Network Analysis

Introduction to Systematic Review and Meta-Analysis
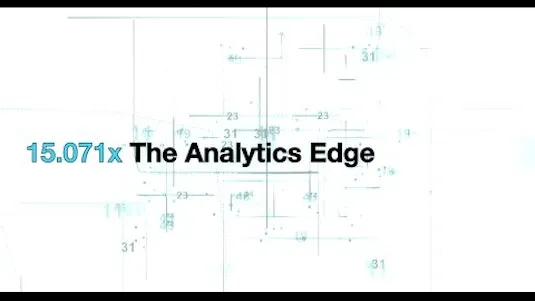
The Analytics Edge

DCO042 - Python For Informatics

Causal Diagrams: Draw Your Assumptions Before Your Conclusions
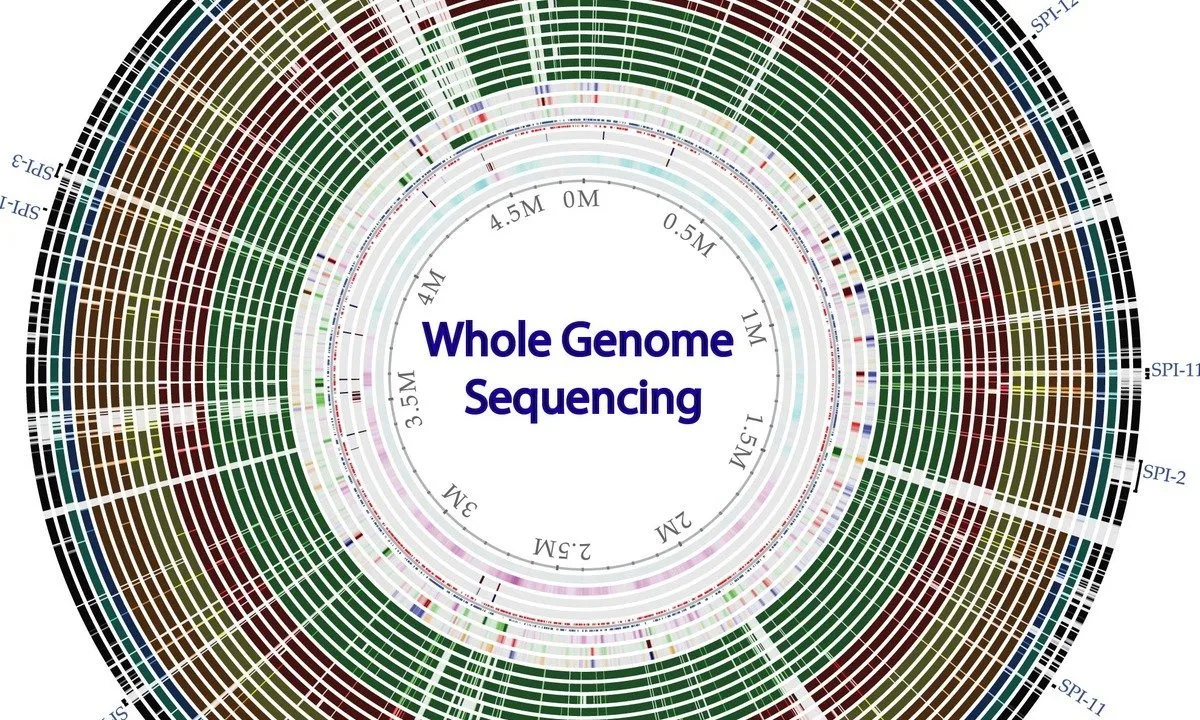
Whole genome sequencing of bacterial genomes - tools and applications
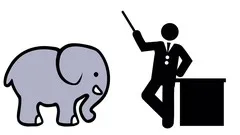
Hadoop Cluster Administration Course: Guide for Hadoop Admin
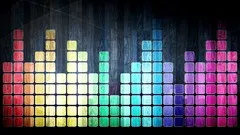
Cluster Analysis and Unsupervised Machine Learning in Python
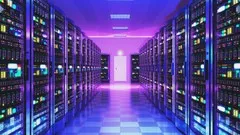
Start your review of Unsupervised Machine Learning: Cluster Analysis Algorithms